RCFL: Redundancy-Aware Collaborative Federated Learning in Vehicular Networks
IEEE TRANSACTIONS ON INTELLIGENT TRANSPORTATION SYSTEMS(2023)
摘要
In vehicular networks (VNets), vehicular federated learning (VFL) is a new learning paradigm that can protect data privacy of vehicle nodes (VNs) while training models. In VFL, the importance of data (IoD) is a key factor that affects model training accuracy. However, due to the heterogeneity of data in the VFL, it is a challenge to evaluate the quality of data owned by different VNs and design an efficient federated learning scheme to enable the VNs to complete learning tasks collaboratively. In this paper, we consider the IoD and propose a redundancy-aware collaborative federated learning (RCFL) scheme for the VFL. In the scheme, by jointly considering the data quality and the cooperation among VNs, we first design a redundancy-aware federated learning architecture to efficiently provide learning services in VNets. Then, we develop a data importance model that integrates the non-independent and identically distributed (non-IID) degree and the redundancy of data (RoD) to evaluate the data quality and formulate the cooperation of the VNs as a coalition game to improve their data importance, where the equilibrium of the coalition game is obtained by designing a coalition formation algorithm. After that, by considering the diversified characteristics of data and the available resources of different VNs in each coalition, a coalition-based federated learning algorithm is designed to enable the distributed coalitions to complete the learning task cooperatively with the target of improving the learning accuracy. The simulation results show that the proposed scheme outperforms the benchmark schemes in terms of the IoD obtained by the VNs and the training accuracy.
更多查看译文
关键词
Federated learning,vehicular networks,data importance,coalition game
AI 理解论文
溯源树
样例
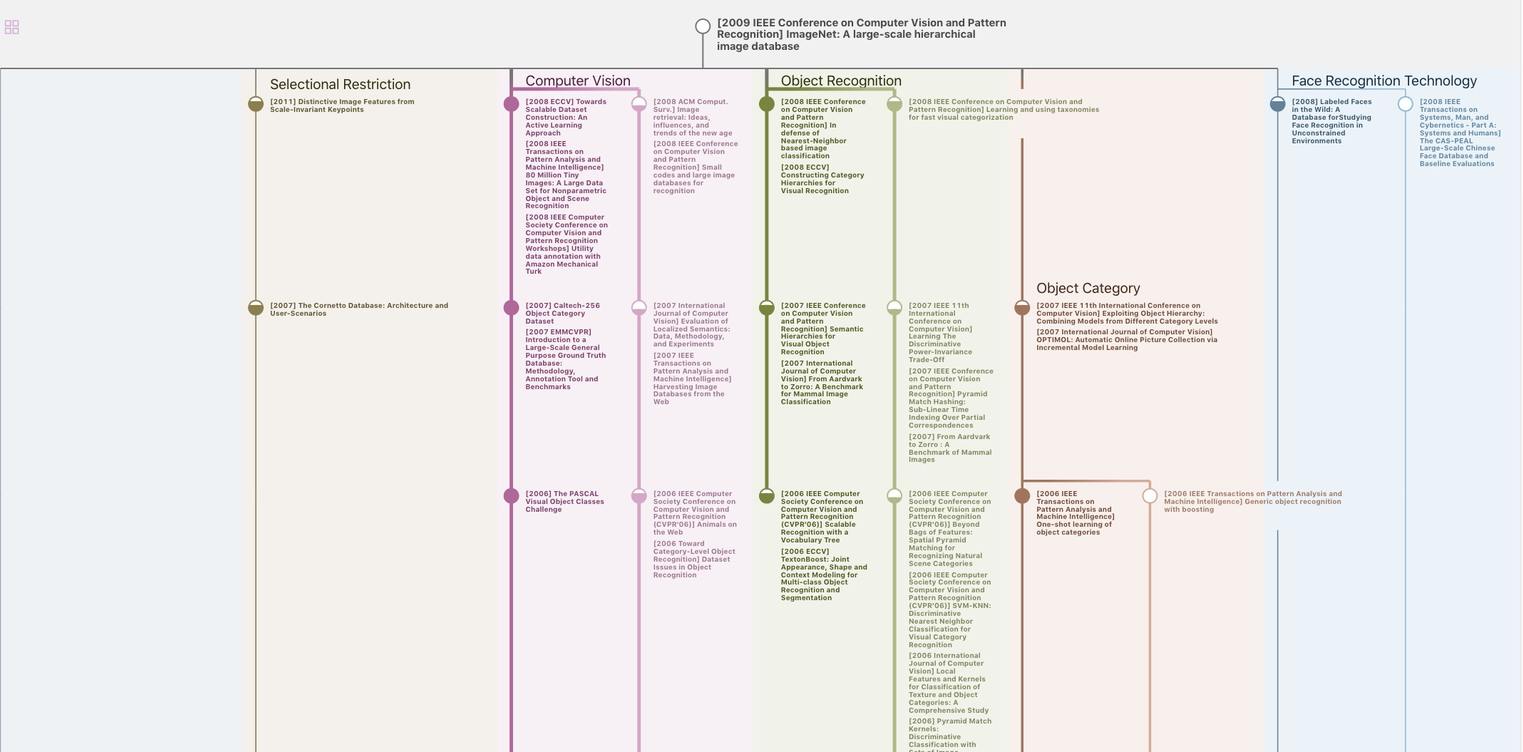
生成溯源树,研究论文发展脉络
Chat Paper
正在生成论文摘要