Goal-LBP: Goal-Based Local Behavior Guided Trajectory Prediction for Autonomous Driving
IEEE TRANSACTIONS ON INTELLIGENT TRANSPORTATION SYSTEMS(2023)
摘要
In recent years, the design of models for performing the trajectory prediction task, one of the critical tasks in autonomous driving, has received great attention from researchers. However, accurately predicting future locations is challenging due to the difficulty of learning accurate intentions and modeling multimodality. Historical paths at a certain location can help predict the future trajectory of an agent currently located in that position and address these limitations. In this work, we propose a goal-based local behavior guided model, Goal-LBP, using such information (referred to as local behavior data) to generate potential goals and guide the prediction of trajectories conditioned on such goals. Goal-LBP uses Transformer encoders to extract homogeneous features and attention mechanism to represent the heterogeneous interactions and subsequently uses an encoder-decoder Gated Recurrent Unit (GRU) model to generate predictions. We evaluate our Goal-LBP using two large-scale real-world autonomous driving datasets, namely nuScenes and Argoverse. Our results show that compared to several SOTA models, Goal-LBP achieves the best ADE/FDE performance and it ranked #2 on the leaderboard of the nuScenes trajectory benchmark in June 2023. In addition, we also demonstrate that our local behavior estimator block can be easily added to two existing SOTA methods, namely AgentFormer and LaPred. Adding this LBE block improves the original AgentFormer and LaPred performance by at least 10%.
更多查看译文
关键词
Trajectory prediction,historical local data,goal-based method,attention mechanism
AI 理解论文
溯源树
样例
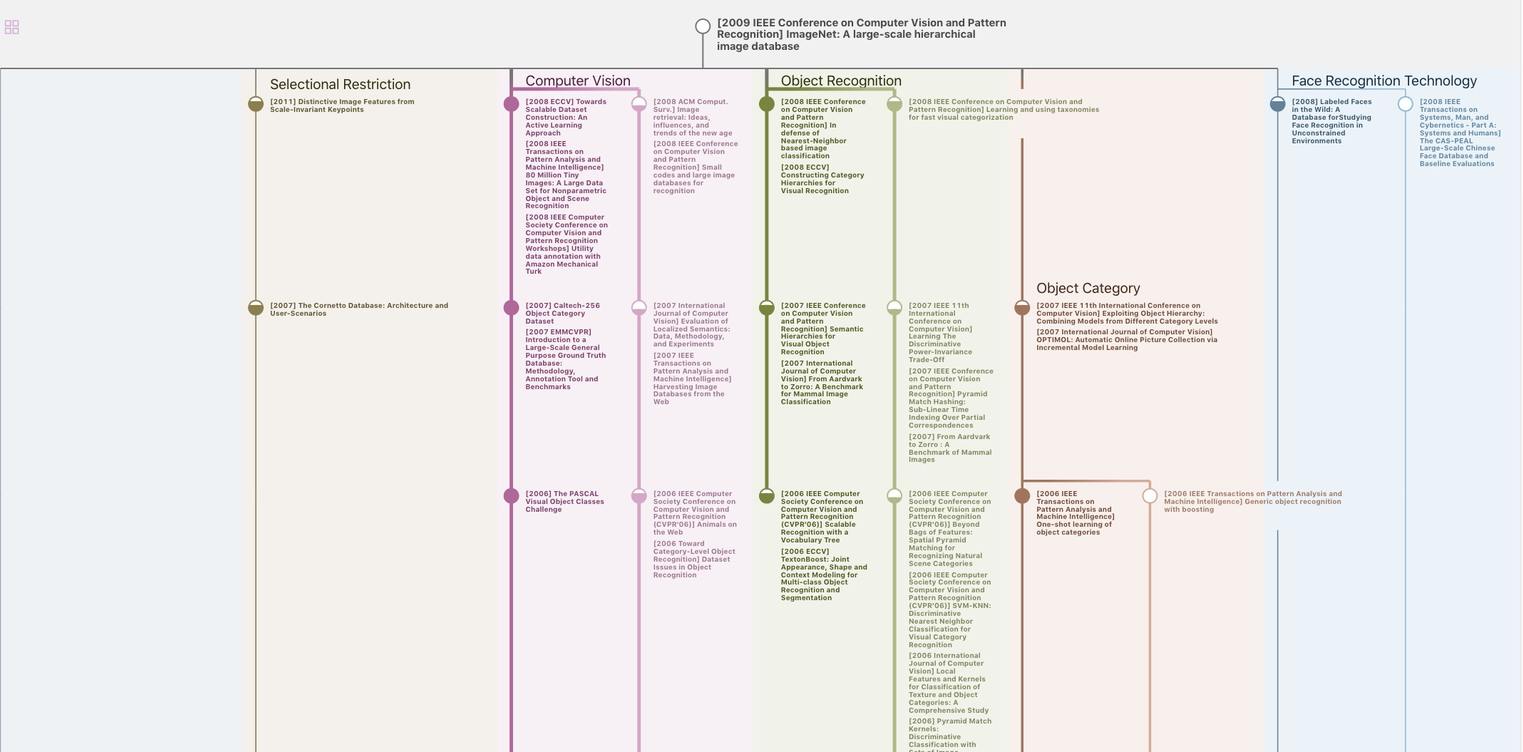
生成溯源树,研究论文发展脉络
Chat Paper
正在生成论文摘要