A High Throughput In-MRAM-Computing Scheme Using Hybrid p-SOT-MTJ/GAA-CNTFET
IEEE TRANSACTIONS ON CIRCUITS AND SYSTEMS I-REGULAR PAPERS(2023)
摘要
Silicon-based semiconductor transistors are approaching their physical limits due to shrinking feature sizes. Simultaneously, traditional silicon-based von Neumann architectures exhibit significant latency and power consumption issues in data-centric applications, such as the Internet of Things and artificial intelligence. To tackle these challenges, this study introduces a novel approach: Magnetoresistance Random Access Memory (MRAM) computing in-memory (CIM) using gate-all-around carbon nanotube field-effect transistors (GAA-CNTFET). The proposed MRAM array comprised three transistors and one perpendicular magnetic anisotropy spin-orbit torque magnetic tunnel junction (p-SOT-MTJ) (3T1M) cell and achieves full-array Boolean logic operations and half/full-adder operations. The calculated results can be stored in-situ during the computing phase without requiring additional peripheral circuits. A 16 Kb MRAM was simulated in both GAA-CNTFET/p-SOT-MTJ and 14-nm FinFET/p-SOT-MTJ technologies to examine the effectiveness of the proposed design. Compared to its 14-nm FinFET/p-SOT-MTJ counterparts, the write and computing latencies of the GAA-CNTFET/p-SOT-MTJ CIM macro were reduced by approximately 21% and 20.6%, respectively, while the read and computing energy consumption by approximately 45.3% and 24.7%, respectively. Moreover, the proposed in-memory Boolean logic throughput was 8192 GOPS, which was approximately 160-250 times higher than that of existing CIM solutions, in which only two rows of word lines can be activated.
更多查看译文
关键词
Boolean logic operation,magnetoresistance random access memory,computing in-memory,in-situ storing,half/full adder,gate-all-around carbon nanotube field-effect-transistor,von Neumann bottleneck
AI 理解论文
溯源树
样例
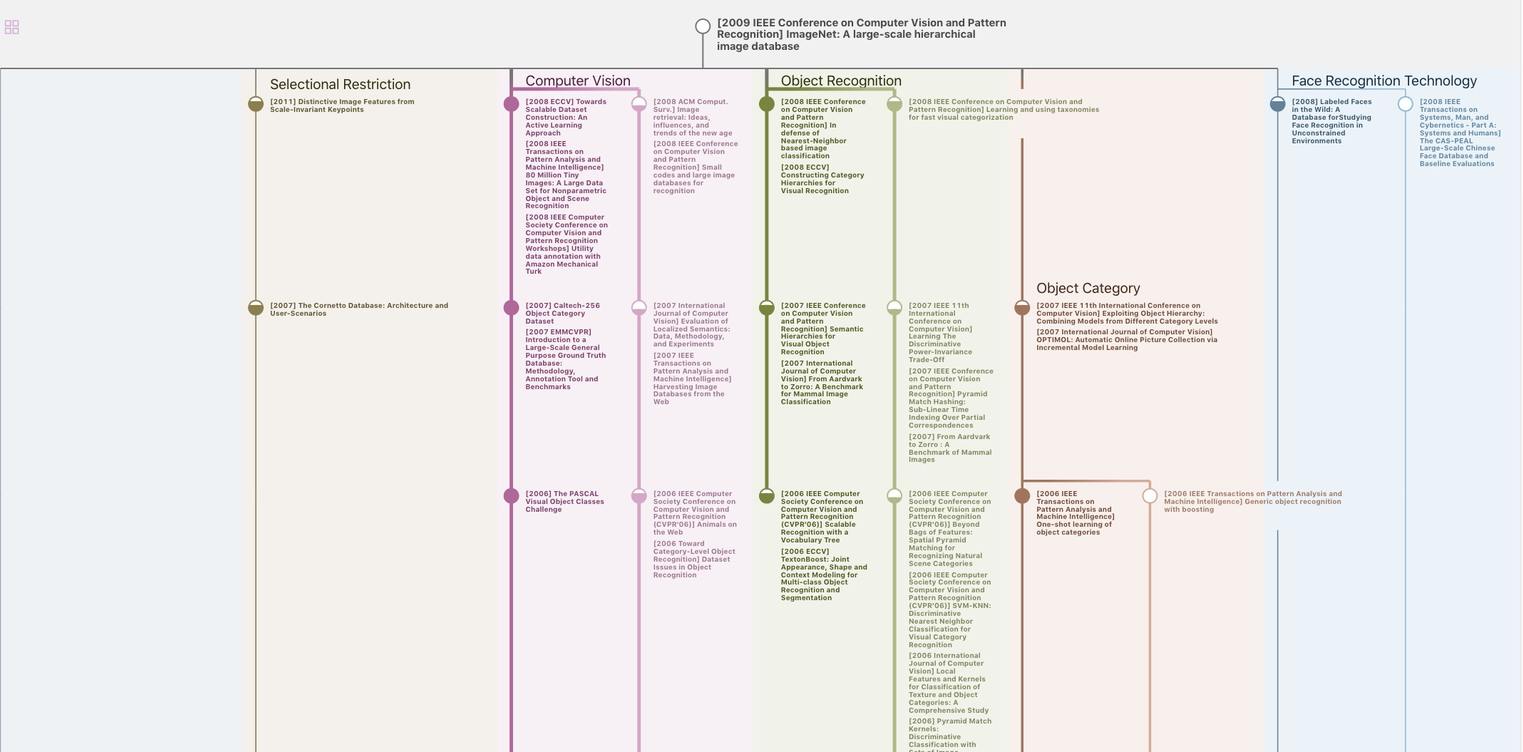
生成溯源树,研究论文发展脉络
Chat Paper
正在生成论文摘要