Machine-learning-assisted discovery of 212-Zintl-phase compounds with ultra-low lattice thermal conductivity
JOURNAL OF MATERIALS CHEMISTRY A(2024)
摘要
Zintl-phase compounds hold immense potential for thermoelectric applications owing to their intrinsically low lattice thermal conductivity (kappa L). However, numerous 212-Zintl-phase compounds remain largely unexplored due to the challenges in assessing their thermal and electrical transport properties via traditional trial-and-error approaches. Here, we present a gradient boosting regressor (GBR) machine-learning (ML) model to predict and discover 5 unexplored and promising 212-Zintl-phase compounds with kappa L lower than 2 W (mK)-1 at 300 K. The model demonstrated excellent predictive capability with a coefficient of determination (R2) of 0.988 and root mean square error (RMSE) of 0.083 W (mK)-1 on the test set using tenfold cross-validation. Notably, the top-ranked compound Ba2ZnBi2 exhibited an ultra-low kappa L of approximately 1 W (mK)-1 at 300 K, substantially lower than those of other types of Zintl-phase compounds like 122-, and 111-types. Our theoretical calculations further validated the ultra-low kappa L of Ba2ZnBi2, and revealed that it originates from the large three-phonon scattering rates and the low group velocities due to the weak atomic interaction in the system. Therefore, our study demonstrates the power of combining ML and first-principles calculations to rapidly identify promising candidates for thermoelectric applications. Machine-learning-assisted discovery of 212-Zintl-phase compounds with ultra-low lattice thermal conductivity.
更多查看译文
AI 理解论文
溯源树
样例
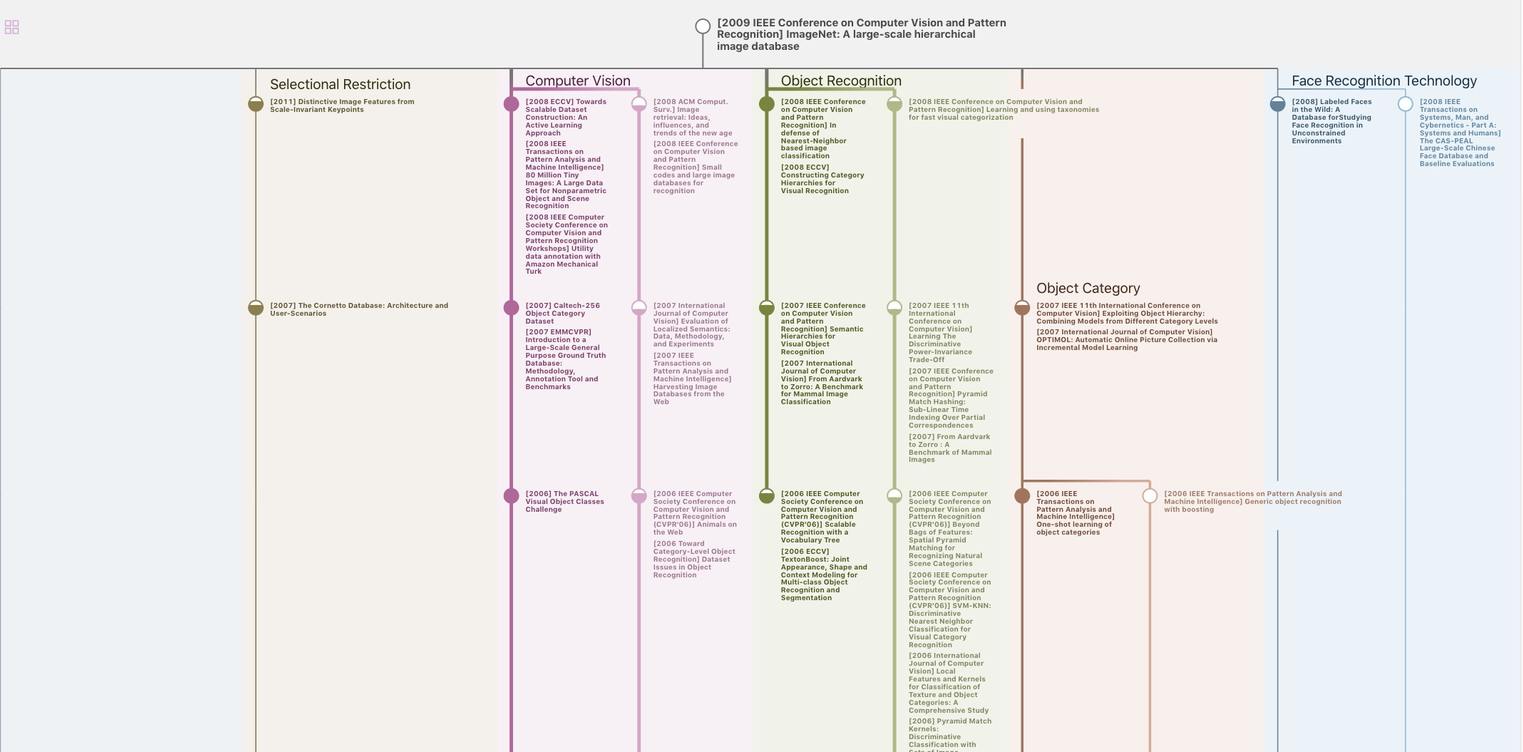
生成溯源树,研究论文发展脉络
Chat Paper
正在生成论文摘要