Data-driven adaptive testing resource allocation strategies for real-time monitoring of infectious diseases
IISE TRANSACTIONS(2023)
摘要
Since 2002, with the SARS outbreak, infectious diseases, including the ongoing COVID-19 pandemic, have continued to be a major global public health threat. It is critical to develop effective data science methods to quickly detect disease outbreaks and contain their rapid globalized spread. However, in practice, limited testing availability, and thus insufficient testing data poses significant challenges in effective analysis and real-time monitoring of infectious diseases, especially during early stages of a novel disease outbreak. To tackle these challenges, this article proposes adaptive testing resource allocation strategies integrated with a physics-informed model to dynamically allocate limited testing resources across different communities. The physics-informed model accounts for transmission dynamics and health disparities, enabling effective health risk assessment despite limited data. By integrating nonstationary Multi-Armed Bandit techniques that strike superior balance between exploring the communities with high uncertain risks and exploiting those with high risk levels, the proposed methodology facilitates test allocation to collect high-quality testing data for early outbreak detection. Theoretical analysis is carried out to evaluate the performance of the proposed allocation strategies, ensuring either sublinear or linear dynamic pseudo-regret under regularity assumptions. A comprehensive simulation study is conducted under three transmission scenarios to thoroughly evaluate the proposed methodology.
更多查看译文
关键词
Data-driven,health disparity,infectious diseases,multi-armed bandit,real-time monitoring,resource allocation,transmission dynamics
AI 理解论文
溯源树
样例
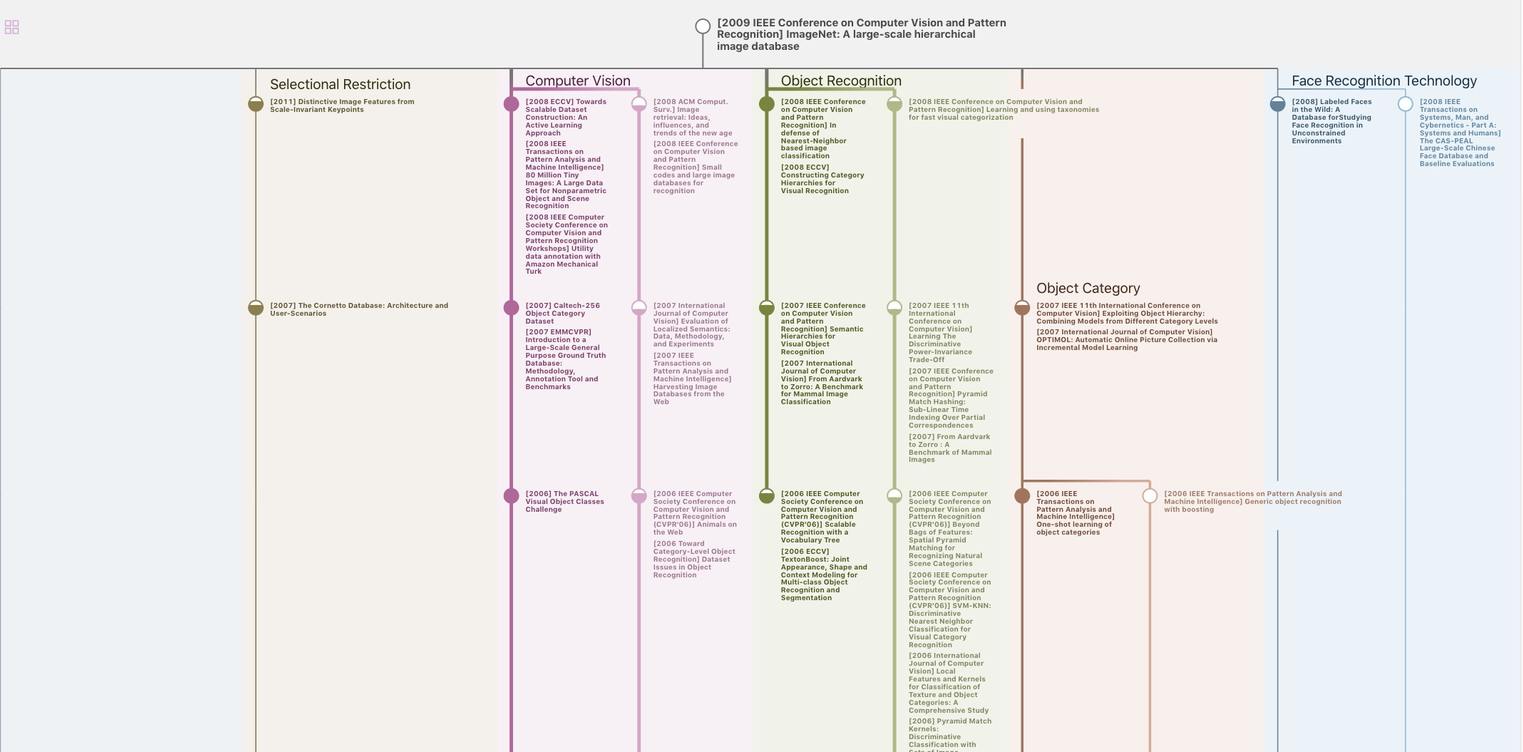
生成溯源树,研究论文发展脉络
Chat Paper
正在生成论文摘要