AI-driven behavior biometrics framework for robust human activity recognition in surveillance systems
ENGINEERING APPLICATIONS OF ARTIFICIAL INTELLIGENCE(2024)
摘要
The integration of artificial intelligence (AI) into human activity recognition (HAR) in smart surveillance systems has the potential to revolutionize behavior monitoring. These systems analyze an individual's physiological or behavioral features to continuously monitor and identify any unusual or suspicious activity in video streams, thereby improving security and surveillance measures. Traditional surveillance systems often rely on manual human monitoring, which is resource-intensive, error-prone, and time-consuming. To address these limitations, computer vision-based behavior biometrics has emerged as a solution for secure video surveillance applications. However, implementing automated HAR in real-world scenarios is challenging due to the diversity of human behavior, complex spatiotemporal patterns, varying viewpoints, and cluttered backgrounds. To tackle these challenges, an AI-based behavior biometrics framework is introduced that is based on a dynamic attention fusion unit (DAFU) followed by a temporal-spatial fusion (TSF) network to effectively recognize human activity in surveillance systems. In the first phase of the proposed framework, a lightweight EfficientNetB0 backbone is enhanced by the DAFU to extract human-centric salient features using a unified channel-spatial attention mechanism. In the second phase, the DAFU features with fixed sequence lengths are passed to the proposed TSF network to capture the temporal, spatial, and behavioral dependencies in video data streams. The integration of Echo-ConvLSTM in the TSF further enhances accuracy and robustness by combining temporal dependencies from the echo state network with spatial and temporal dependencies from the convolutional long short-term memory (ConvLSTM). The proposed AI-based behavior biometrics framework is evaluated using four publicly available HAR datasets (UCF101, HMDB51, UCF50, and YouTube Action), yielding higher accuracies of 98.734%, 80.342%, 98.987%, and 98.927%, which demonstrate the superior performance when compared with state-of -the-art (SOTA) methods.
更多查看译文
关键词
Activity recognition,Surveillance system,Machine learning,Computer vision,Video classification,Deep learning
AI 理解论文
溯源树
样例
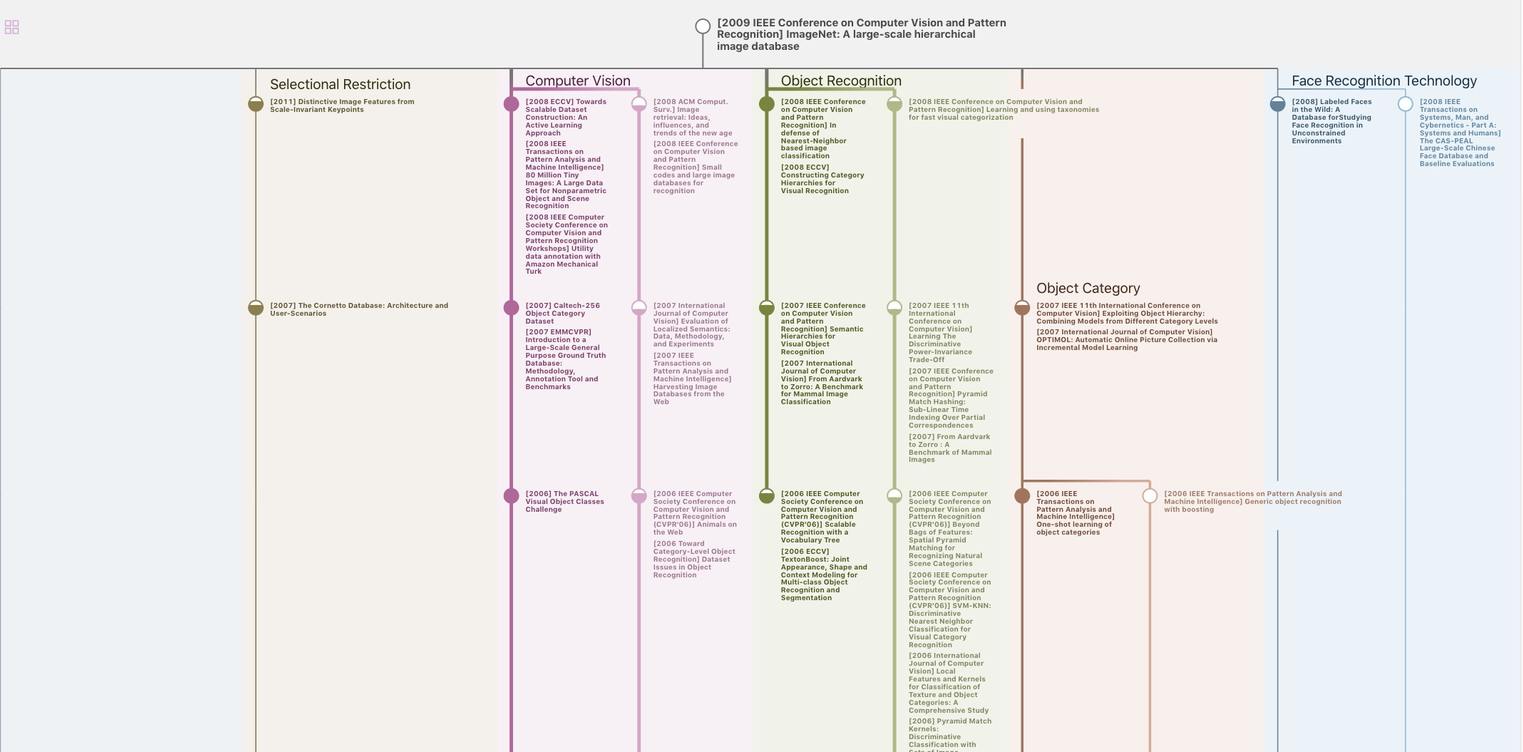
生成溯源树,研究论文发展脉络
Chat Paper
正在生成论文摘要