Anomaly Detection in Cloudnative B5G Systems using Observability and Machine Learning COTS Solutions
JOURNAL OF INTERNET SERVICES AND APPLICATIONS(2023)
摘要
The advent of B5G networks has revolutionized the telecommunications landscape by transitioning hard-ware resources to software components, predominantly running on cloud-based infrastructures. However, this 'soft-warization' extends across the radio access, transport, and core networks, introducing complex challenges in real-time network management. In this context of the 'softwarization', it is imperative to make the behavior of B5G systems readily observable for effective management and fault diagnosis. This article presents a comprehensive empirical investigation of observability within a B5G system, specifically focusing on its radio access and core networks. The study enhances the system's observability by combining advanced metric analysis and log parsing. Our method integrates Commercial Off-The-Shelf machine learning algorithms to diagnose anomalies and automate failure tasks. Besides that, our evaluation of the Cloud-Native Observability Tools services revealed a significant memory footprint, accounting for 86% of the total memory usage and 22% overall CPU utilization. The findings also highlight that our approach mitigates the issue of non-standardization in log data, thereby facilitating proactive failure anticipation. This study can aggregate significant value for developing automated, self-healing B5G network systems.
更多查看译文
关键词
Observability,5G Systems,Metrics,Log Processing,Machine Learning,COTS
AI 理解论文
溯源树
样例
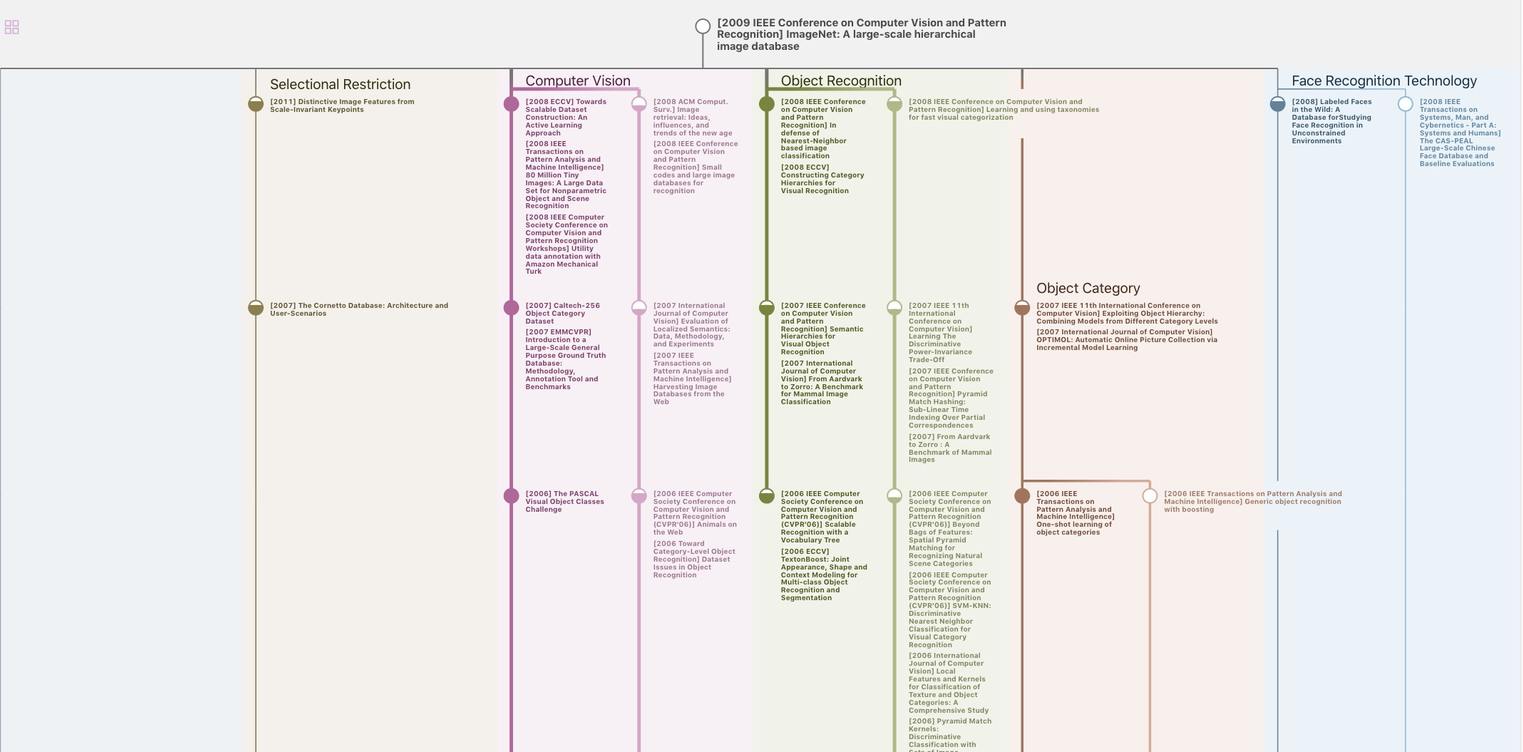
生成溯源树,研究论文发展脉络
Chat Paper
正在生成论文摘要