Privately Estimating a Gaussian: Effiicient, Robust, and Optimal
PROCEEDINGS OF THE 55TH ANNUAL ACM SYMPOSIUM ON THEORY OF COMPUTING, STOC 2023(2023)
摘要
In this work, we give efficient algorithms for privately estimating a Gaussian distribution in both pure and approximate differential privacy (DP) models with optimal dependence on the dimension in the sample complexity. In the pure DP setting, we give an efficient algorithm that estimates an unknown 3 -dimensional Gaussian distribution up to an arbitrary tiny total variation error using (O) over tilde (d(2) log kappa) samples while tolerating a constant fraction of adversarial outliers. Here, kappa is the condition number of the target covariance matrix. The sample bound matches best non-private estimators in the dependence on the dimension (up to a polylogarithmic factor). We prove a new lower bound on differentially private covariance estimation to show that the dependence on the condition number kappa in the above sample bound is also tight. Prior to our work, only identifiability results (yielding inefficient super-polynomial time algorithms) were known for the problem. In the approximate DP setting, we give an efficient algorithm to estimate an unknown Gaussian distribution up to an arbitrarily tiny total variation error using (O) over bar (d(2)) samples while tolerating a constant fraction of adversarial outliers. Prior to our work, all effcient approximate DP algorithms incurred a super-quadratic sample cost or were not outlier-robust. For the special case of mean estimation, our algorithm achieves the optimal sample complexity of (O) over bar (d), improving on a (O) over bar (d(1.5)) bound from prior work. Our pure DP algorithm relies on a recursive private preconditioning subroutine that utilizes recent work of Hopkins et al. (STOC 2022) on private mean estimation. Our approximate DP algorithms are based on a substantial upgrade of the method of stabilizing convex relaxations introduced by Kothari et al. (COLT 2022). In particular, we improve on their mechanism by using a new unnormalized entropy regularization and a new and surprisingly simple mechanism for privately releasing covariances.
更多查看译文
关键词
DIfferential Privacy,Robust Statistics,High-Dimensional Statistics,Private Statistics
AI 理解论文
溯源树
样例
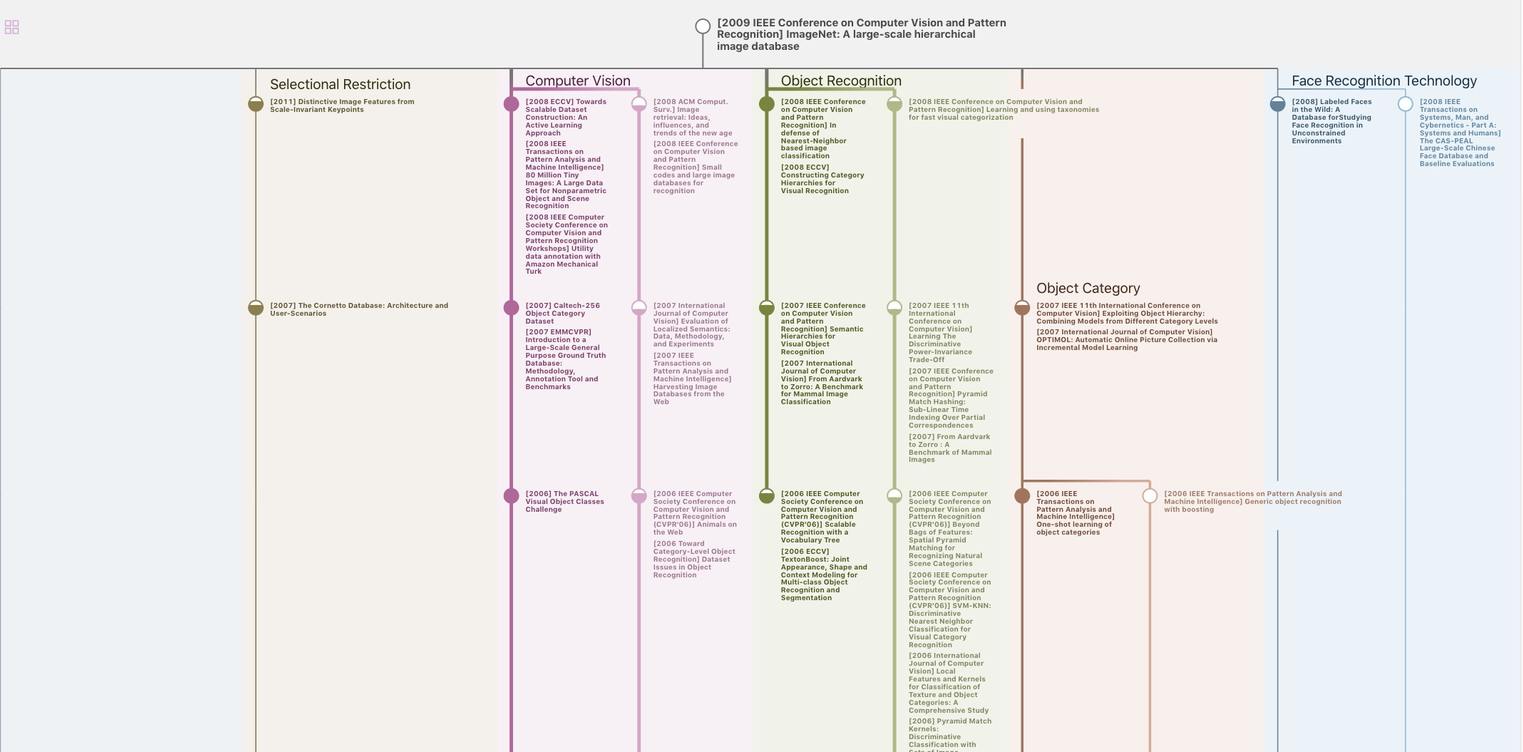
生成溯源树,研究论文发展脉络
Chat Paper
正在生成论文摘要