Predicting Student Engagement Using Sequential Ensemble Model
IEEE TRANSACTIONS ON LEARNING TECHNOLOGIES(2024)
摘要
Predicting student engagement can provide timely feedback and help teachers make adjustments to their practices to meet student needs and improve their learning experience. This article proposes a four-step approach using a sequential ensemble model for engagement prediction, discusses the contribution of different features to the model and the influence of video segmentation in the prediction, reports on two in-the-wild datasets-The Emotion Recognition in the Wild Engagement Prediction (EmotiW-EP) dataset published in 2018 as part of a student engagement task and the Dataset for Affective States in E-Environments (DAiSEE), a general purpose dataset also used in the educational context but not limited to it, and, finally, presents a comprehensive and thorough critical analysis, highlighting crucial factors to consider when using AI/computer vision models in educational datasets for learning purposes. Experiments show that our proposed approach outperforms state-of-the-art approaches by obtaining a mean square error of 0.0386 on the DAiSEE dataset and 0.0610 on the EmotiW-EP dataset. We conclude this article with a critical analysis of the reliability of such predictions in learning environments and propose future directions for the effective use of AI/computer vision models in education.
更多查看译文
关键词
Feature extraction,Predictive models,Face recognition,Task analysis,Head,Mathematical models,Analytical models,Emotion analysis,facial expression recognition,sequential model,student engagement prediction,video analysis
AI 理解论文
溯源树
样例
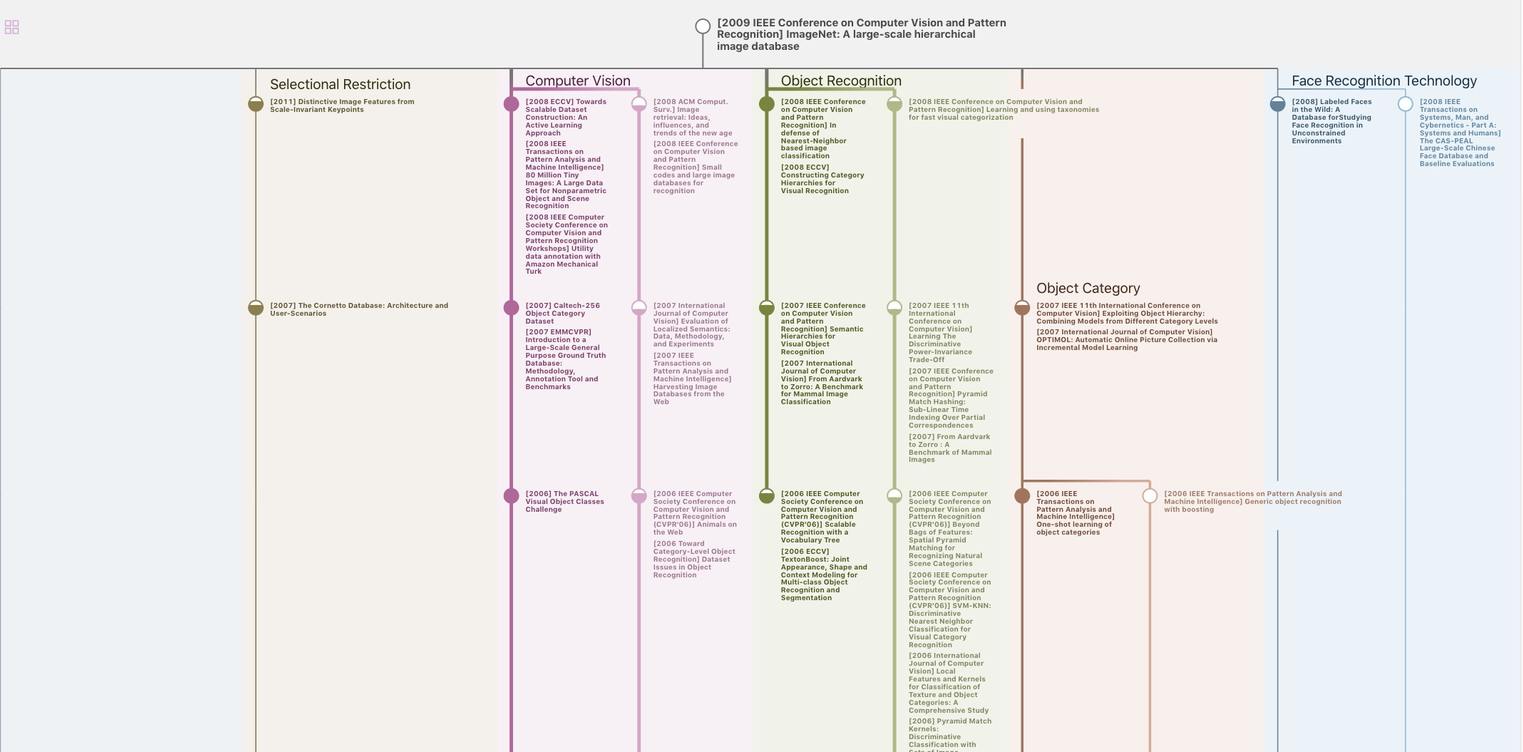
生成溯源树,研究论文发展脉络
Chat Paper
正在生成论文摘要