The predictability of near-term forest biomass change in boreal North America
ECOSPHERE(2024)
摘要
Climate change is driving substantial changes in North American boreal forests, including changes in productivity, mortality, recruitment, and biomass. Despite the importance for carbon budgets and informing management decisions, there is a lack of near-term (5-30 year) forecasts of expected changes in aboveground biomass (AGB). In this study, we forecast AGB changes across the North American boreal forest using machine learning, repeat measurements from 25,000 forest inventory sites, and gridded geospatial datasets. We find that AGB change can be predicted up to 30 years into the future, and that training on sites across the entire domain allows accurate predictions even in regions with only a small amount of existing field data. While predicting AGB loss is less skillful than gains, using a multi-model ensemble can improve the accuracy in detecting change direction to >90% for observed increases, and up to 70% for observed losses. Higher stem density, winter temperatures, and the presence of temperate tree species in forest plots were positively associated with AGB change, whereas greater initial biomass, continentality (difference between mean summer and winter temperatures), prevalence of black spruce (Picea mariana), summer precipitation, and early warning metrics from long-term remote sensing time series were negatively associated with AGB change. Across the domain, we predict nondisturbance-induced declines in AGB at 23% of sites by 2030. The approach developed here can be used to estimate near-future forest biomass in boreal North America and inform relevant management decisions. Our study also highlights the power of machine learning multi-model ensembles when trained on a large volume of forest inventory plots, which could be applied to other regions with adequate plot density and spatial coverage.
更多查看译文
关键词
biomass change,boreal forest,early warning signals,Extreme Gradient Boost,machine learning,North America
AI 理解论文
溯源树
样例
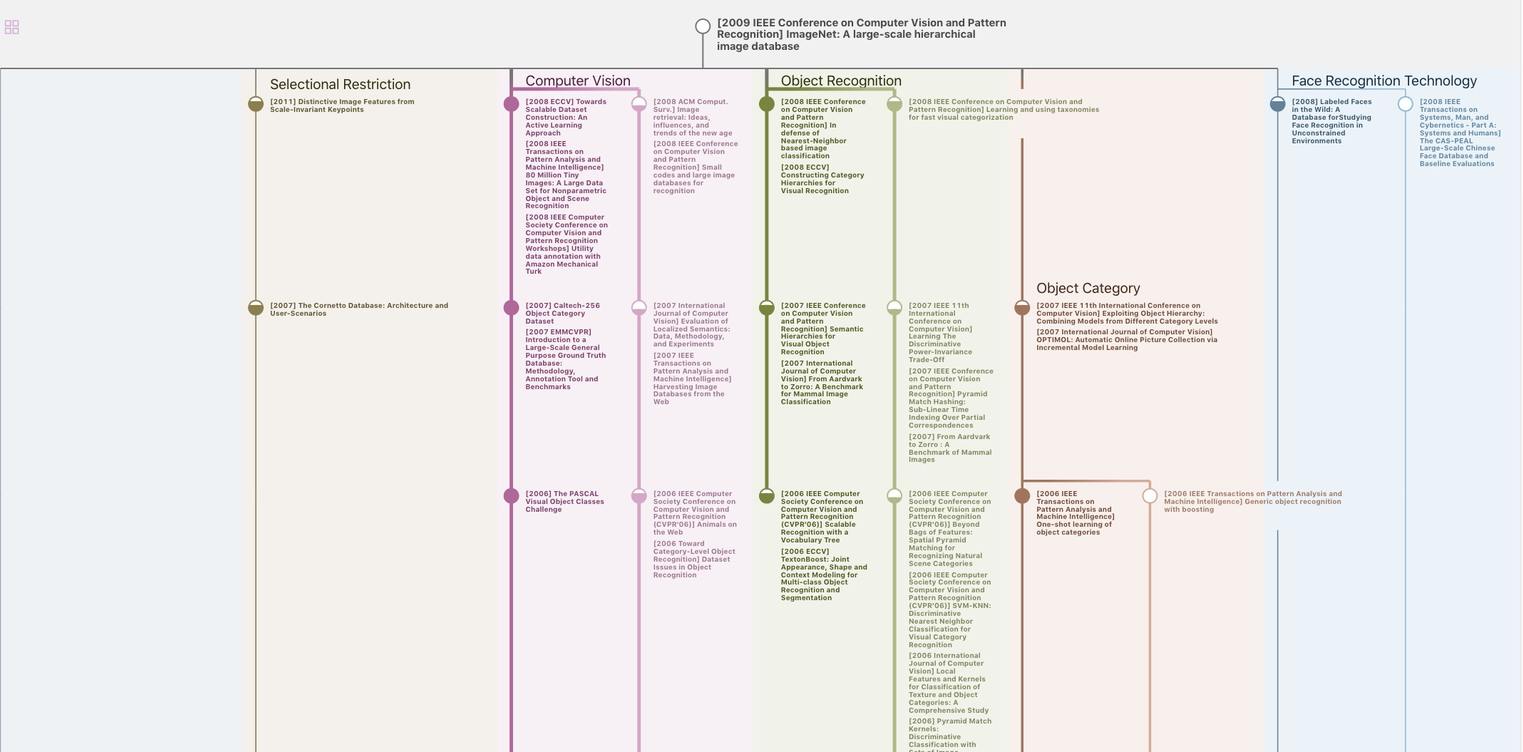
生成溯源树,研究论文发展脉络
Chat Paper
正在生成论文摘要