The Effect of Using Aerosol Variables on the Performance of Deep Learning-based GHI Forecasting Models
crossref(2024)
摘要
Solar energy adoption worldwide has expanded exponentially due to a surge in international interest in producing clean energy and the declining cost of solar power plants and their technology. It is anticipated that by 2050, solar will have surpassed fossil fuels to become the primary source of energy. However, one of the main challenges associated with solar energy production is the instability of photovoltaic (PV) power generation because of weather changes. Short-term forecasting of the power output of photovoltaic systems is essential for efficient management of the power grid and energy markets. This paper aims to evaluate the ability of deep learning (DL) models to provide accurate forecasting of hourly global horizontal irradiance (GHI) using different sets of features, including weather and aerosol variables along with solar radiation components. The results show that the best forecast skills are achieved by the long short-term memory autoencoder (LSTM-AE) model.
更多查看译文
AI 理解论文
溯源树
样例
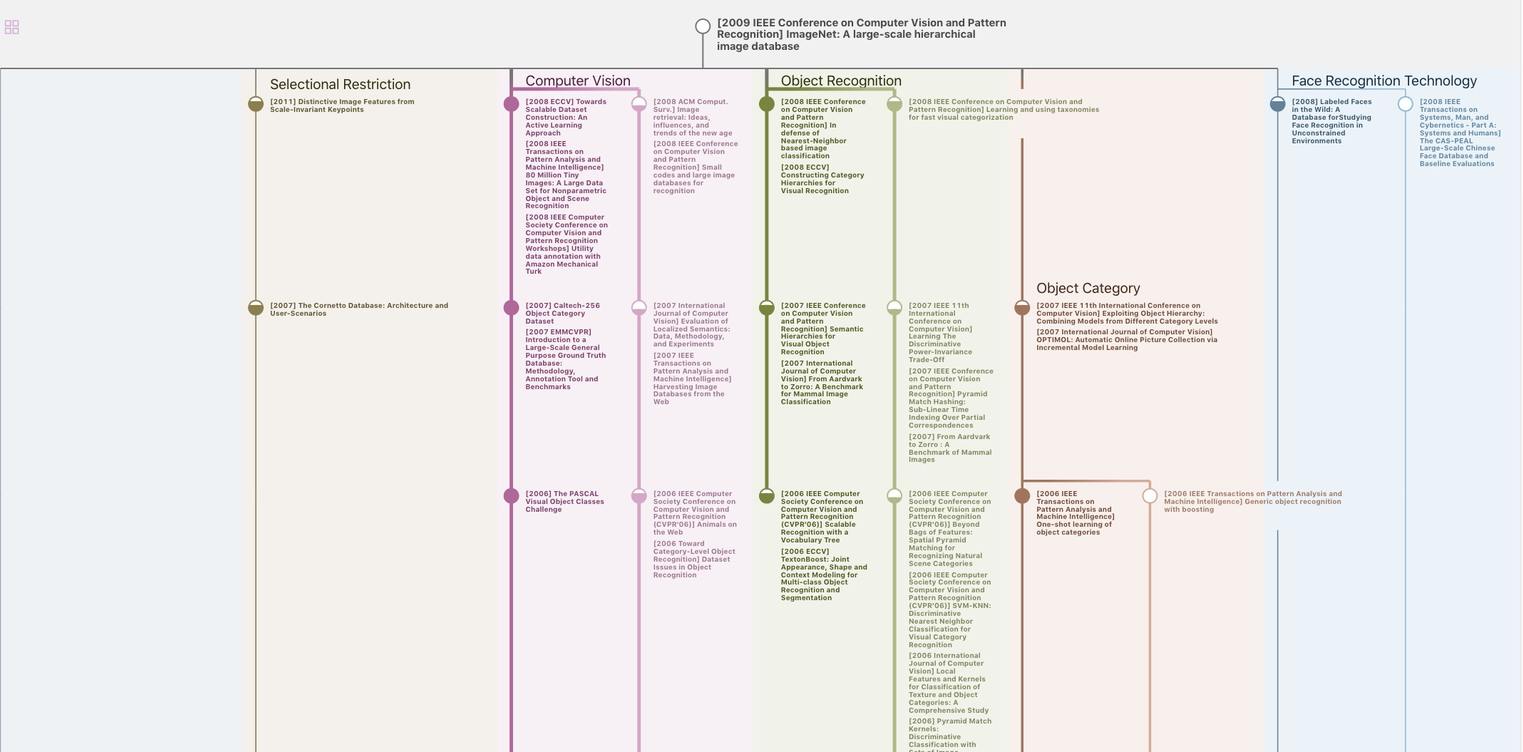
生成溯源树,研究论文发展脉络
Chat Paper
正在生成论文摘要