Incomplete Cross-Modal Retrieval with Deep Correlation Transfer
ACM TRANSACTIONS ON MULTIMEDIA COMPUTING COMMUNICATIONS AND APPLICATIONS(2024)
摘要
Most cross-modal retrieval methods assume the multi-modal training data is complete and has a one-to-one correspondence. However, in the real world, multi-modal data generally suffers from missing modality information due to the uncertainty of data collection and storage processes, which limits the practical application of existing cross-modal retrieval methods. Although some solutions have been proposed to generate the missing modality data using a single pseudo sample, this may lead to incomplete semantic restoration and suboptimal retrieval results due to the limited semantic information it provides. To address this challenge, this article proposes an Incomplete Cross-Modal Retrieval with Deep Correlation Transfer (ICMR-DCT) method that can robustly model incomplete multi-modal data and dynamically capture the adjacency semantic correlation for cross-modal retrieval. Specifically, we construct intra-modal graph attention-based auto-encoder to learn modality-invariant representations by performing semantic reconstruction through intra-modality adjacency correlation mining. Then, we design dual cross-modal alignment constraints to project multi-modal representations into a common semantic space, thus bridging the heterogeneous modality gap and enhancing the discriminability of the common representation. We further introduce semantic preservation to enhance adjacency semantic information and achieve cross-modal semantic correlation. Moreover, we propose a nearest-neighbor weighting integration strategy with cross-modal correlation transfer to generate the missing modality data according to inter-modality mapping relations and adjacency correlations between each sample and its neighbors, which improves the robustness of our method against incomplete multi-modal training data. Extensive experiments on three widely tested benchmark datasets demonstrate the superior performance of our method in cross-modal retrieval tasks under both complete and incomplete retrieval scenarios. Our used datasets and source codes are available at https://github.com/shidan0122/DCT.git.
更多查看译文
关键词
Incomplete cross-modal retrieval,adjacency semantic correlation,robustness,graph attention
AI 理解论文
溯源树
样例
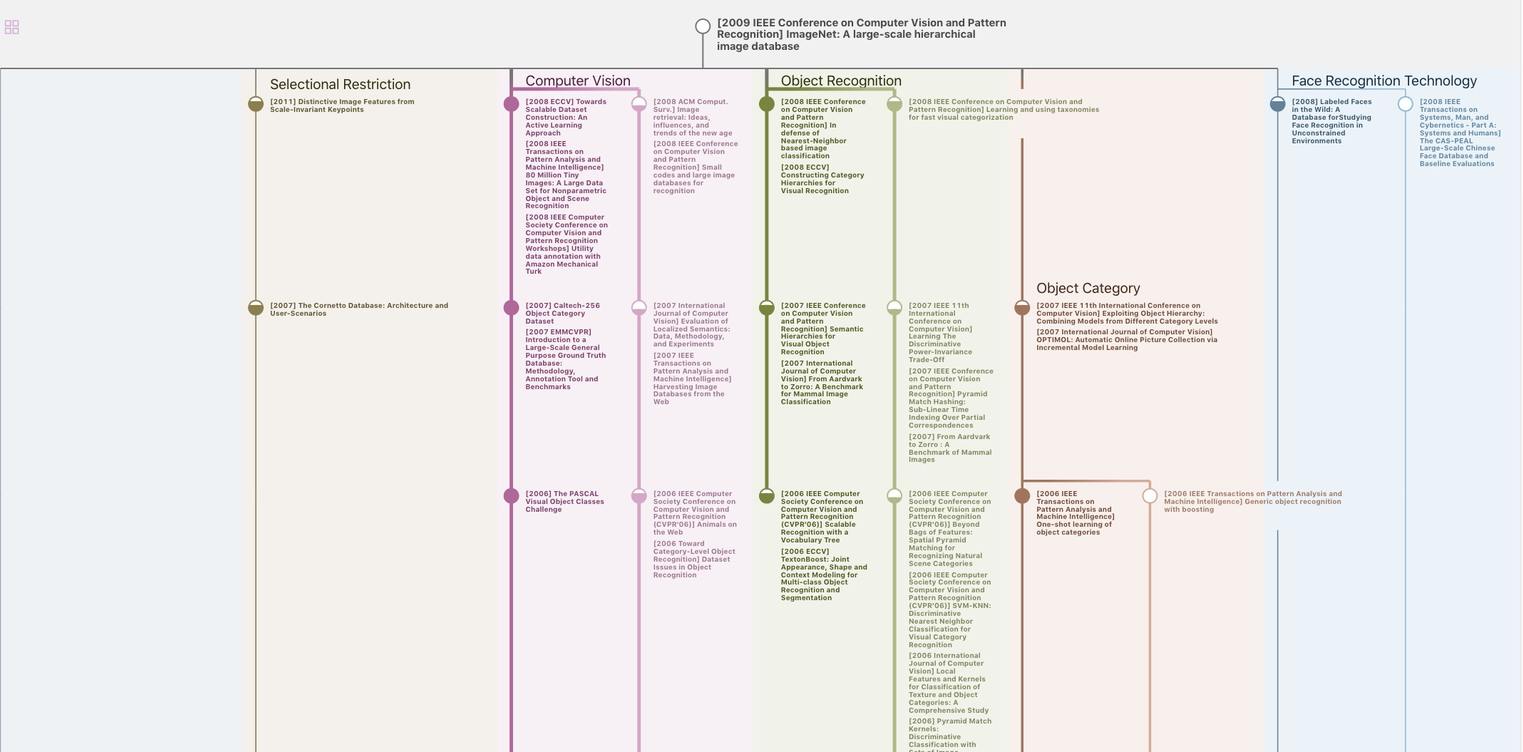
生成溯源树,研究论文发展脉络
Chat Paper
正在生成论文摘要