A novel AI-driven model for erosion prediction for elbow in gas-solid two-phase flows
WEAR(2024)
摘要
Erosion is inevitable in pipeline gathering and transportation systems. Predicting erosion in typical pipe fittings is pivotal to ensure the safety and efficiency of the equipment. This work develops a novel predictive model for erosion in elbows under gas-solid flow conditions. The proposed model employs the Deep Belief Network (DBN) for feature extraction from the input parameters. Meanwhile, Kernel Extreme Learning Machine (KELM) is implemented to deeply analyze correlation between the eigenvalues and the erosion rate, thus improving the model's generalization performance. Furthermore, the Improved Coati Optimization Algorithm (ICOA) is utilized to optimize the model's hyperparameters, contributing to increased accuracy. To validate the reliability of the ICOA - DBN - KELM model, it was applied to both the experimental and simulated datasets. The outcomes demonstrate that the proposed model attains the highest prediction precision in both datasets compared to other models. Additionally, the model was assessed for reasonableness concerning trend consistency and dimensionless numbers.
更多查看译文
关键词
Gas -solid flow,Elbow,Erosion,Deep belief networks,Kernel extreme learning machine,Heuristic algorithm
AI 理解论文
溯源树
样例
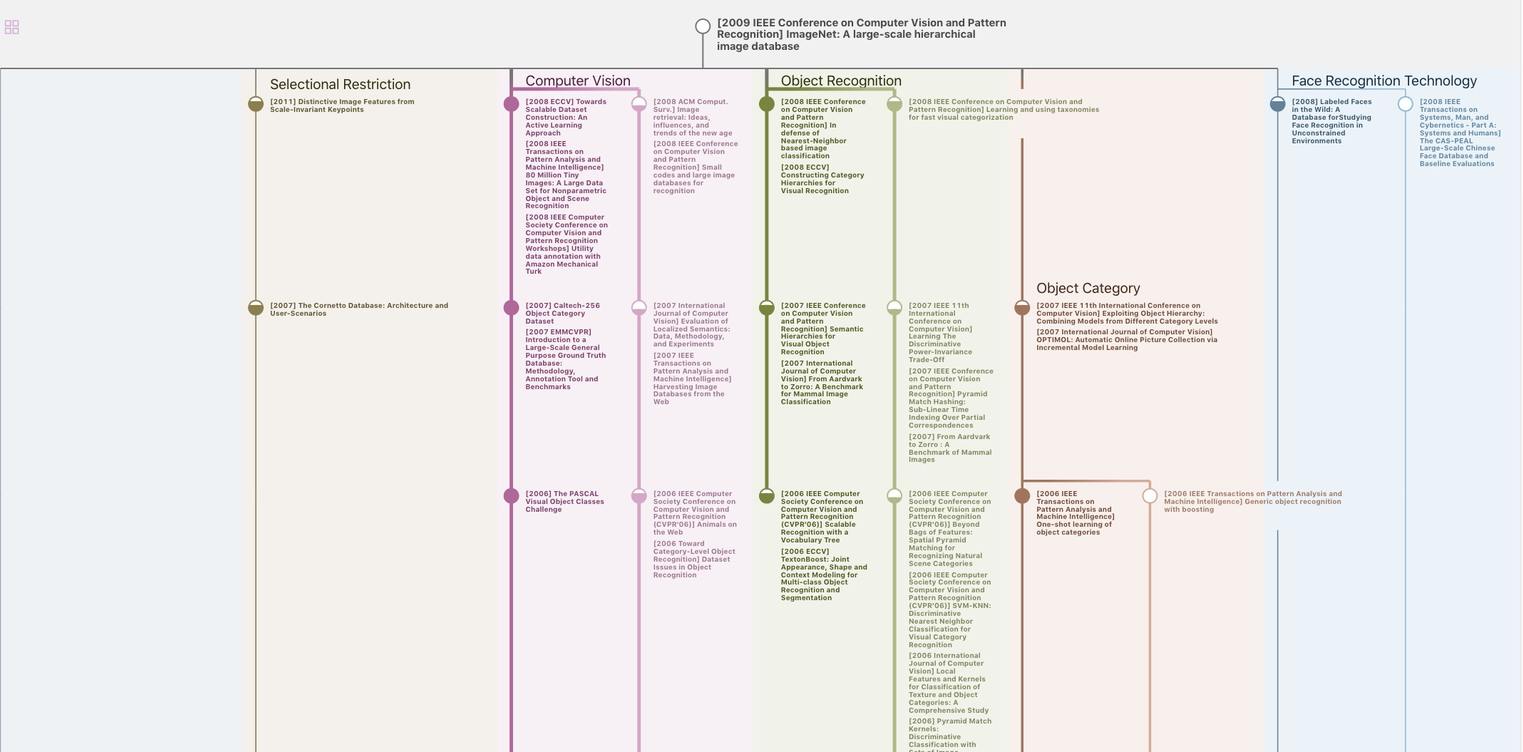
生成溯源树,研究论文发展脉络
Chat Paper
正在生成论文摘要