KONet: Toward a Weighted Ensemble Learning Model for Knee Osteoporosis Classification
IEEE ACCESS(2024)
摘要
Knee osteoporosis (KOP) is a skeletal disorder characterized by bone tissue degradation and low bone density, leading to a high risk of bone fractures in the knee area. The traditional method for identifying knee osteoporosis is knee radiography, which requires sufficient expertise from specialists. However, the sheer volume of X-rays and the subtle variations among them may lead to misinterpretation. In recent years, deep learning algorithms have revolutionized medical diagnosis and reduced misclassification. Specifically, convolutional neural network (CNN)-based algorithms have been utilized to automate the diagnostic process as they have the inherent ability to extract important features that are difficult to identify manually. However, relying on a single method may result in suboptimal performance, leading to ineffective deployment in the medical domain. To alleviate this issue, in this study, we propose a robust detection method, KONet, which utilizes a weighted ensemble approach to distinguish between normal and osteoporotic knee conditions, even when there are minor variations in the data. To validate the architectural choices in the ensemble approach, we conducted experiments on various state-of-the-art CNN-based models using transfer learning. Extensive experiments indicated that the proposed model achieves a higher accuracy than existing models, outperforming the state-of-the-art models by a significant margin.
更多查看译文
关键词
Convolutional neural network,classification model,knee osteoporosis,skeletal health,transfer learning,weighted ensemble learning
AI 理解论文
溯源树
样例
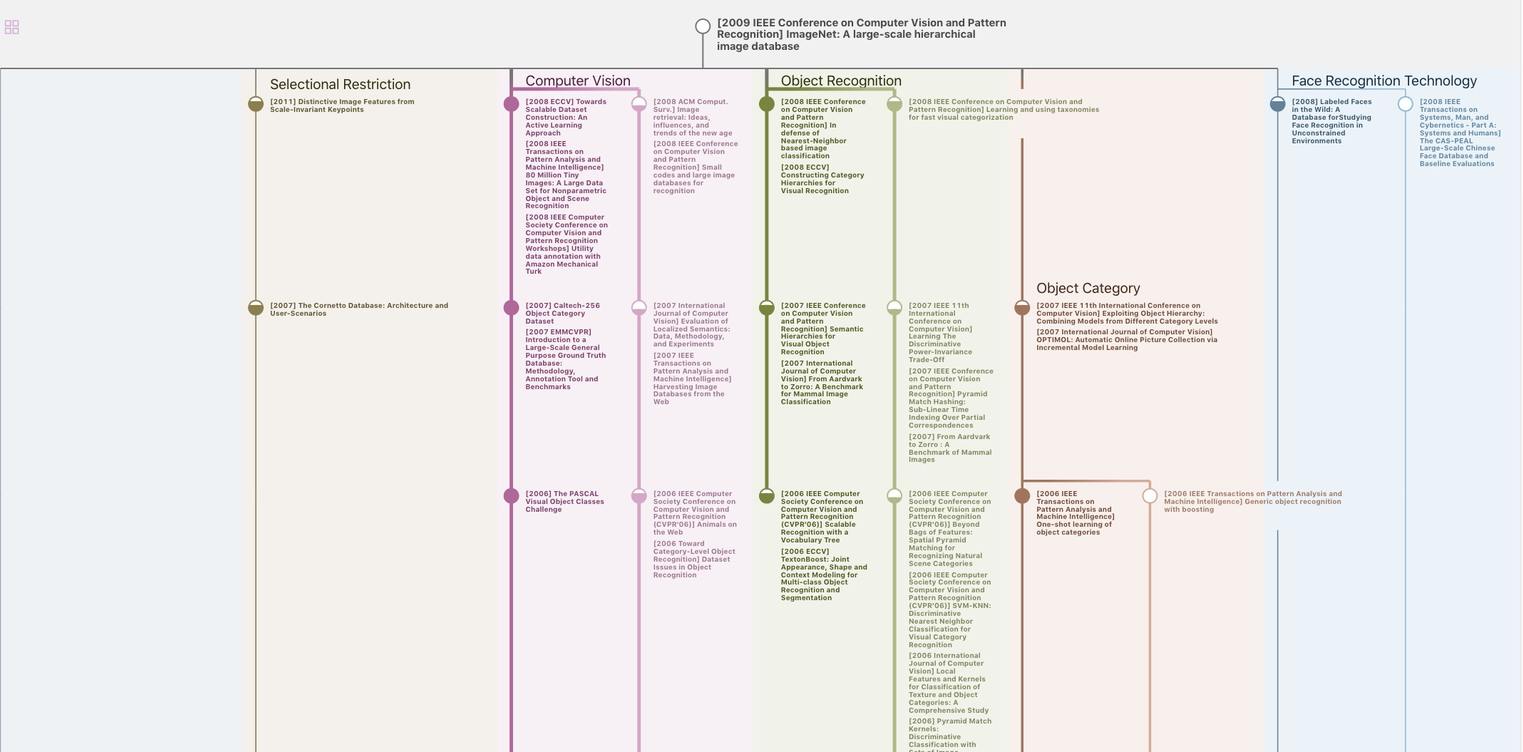
生成溯源树,研究论文发展脉络
Chat Paper
正在生成论文摘要