TC-GCN: Triple cross-attention and graph convolutional network for traffic forecasting
INFORMATION FUSION(2024)
摘要
With the rapid development of urbanization, increasingly more data are being acquired by intelligent transportation systems (ITSs), which is of great significance for traffic flow forecasting. Efficient intelligent traffic management systems (TMSs) depend on accurately forecasting traffic flows as well as reasonably researching and judging traffic states. However, the current prediction models used in transportation forecasting tasks are traditional temporal- and spatial -dimensional prediction approaches. Especially when faced with road channelization, the numbers of lanes and lane types increase significantly, causing significant increases in the dimensionality and complexity of traffic data, and research models utilizing the cross -interaction relationships among multiple dimensions have not been considered. This has led to unsatisfactory prediction results in complex traffic environments. Therefore, it is very important to explore the exchange-correlation of complex dimensional data and the mining of hidden attributes. This paper presents a method for performing multidimensional cross -attention and spatiotemporal graph convolution. This method fully considers crossinformation and constructs an attention cross -view between every pair of dimensions among the channel, time and space domains to model the cross -dimensional dependencies of traffic data. We innovatively propose a triple cross -attention and graph convolutional network (TC-GCN), which can achieve further improved traffic forecasting performance. The TC-GCN is verified on two real -world traffic datasets, namely, METR-LA and PEMS-BAY, and the experimental results are compared with those of multiple advanced baselines, showing that the proposed approach outperforms most of these baselines, which proves the effectiveness of the method proposed in this paper.
更多查看译文
关键词
Cross-attention,Graph convolutional network,Dynamic graph modeling,Traffic forecasting
AI 理解论文
溯源树
样例
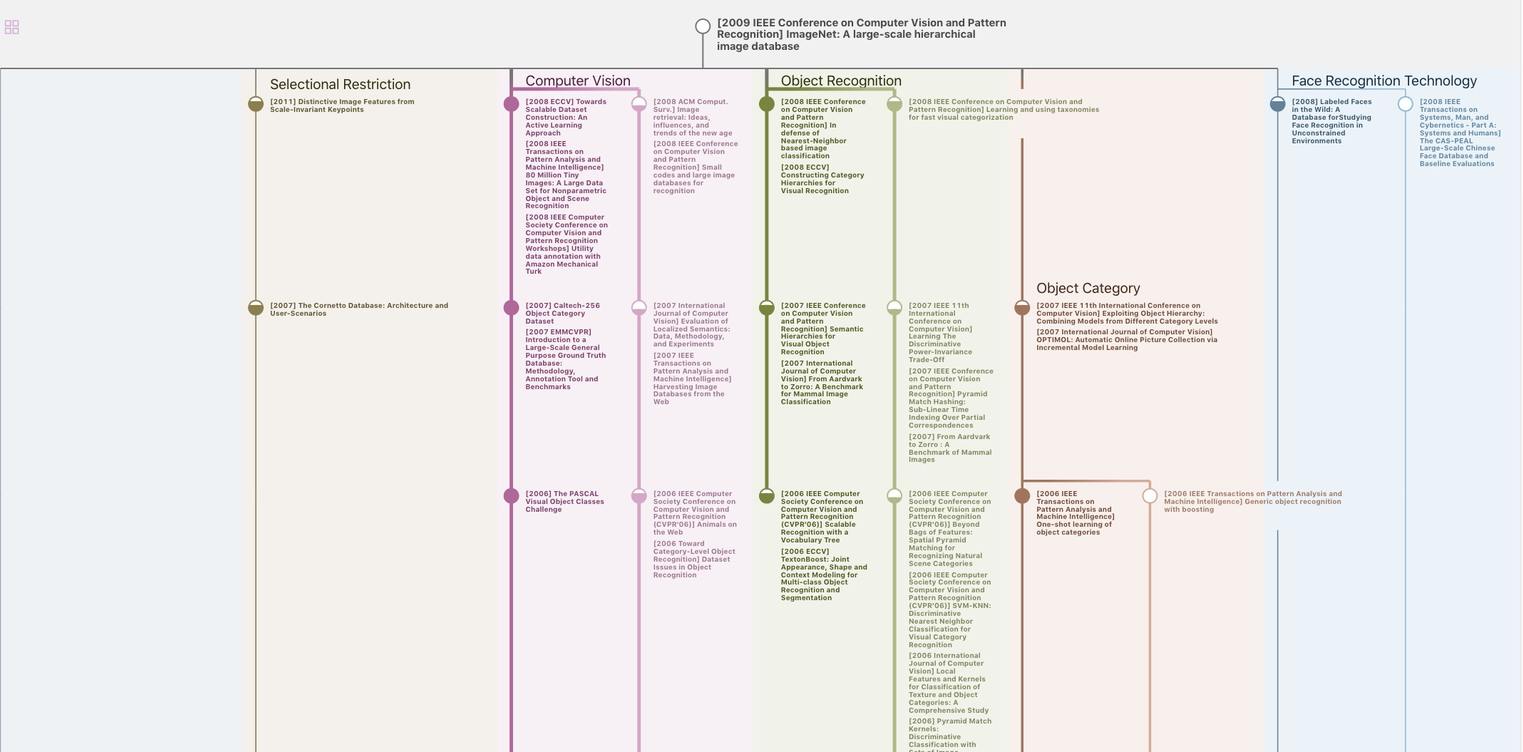
生成溯源树,研究论文发展脉络
Chat Paper
正在生成论文摘要