Safe Reinforcement Learning in Uncertain Contexts
IEEE TRANSACTIONS ON ROBOTICS(2024)
摘要
When deploying machine learning algorithms in the real world, guaranteeing safety is an essential asset. Existing safe learning approaches typically consider continuous variables, i.e., regression tasks. However, in practice, robotic systems are also subject to discrete, external environmental changes, e.g., having to carry objects of certain weights or operating on frozen, wet, or dry surfaces. Such influences can be modeled as discrete context variables. In the existing literature, such contexts are, if considered, mostly assumed to be known. In this work, we drop this assumption and show how we can perform safe learning when we cannot directly measure the context variables. To achieve this, we derive frequentist guarantees for multiclass classification, allowing us to estimate the current context from measurements. Furthermore, we propose an approach for identifying contexts through experiments. We discuss under which conditions we can retain theoretical guarantees and demonstrate the applicability of our algorithm on a Furuta pendulum with camera measurements of different weights that serve as contexts.
更多查看译文
关键词
Heuristic algorithms,Robots,Safety,Uncertainty,Current measurement,Cameras,Dynamical systems,Frequentist bounds,multiclass classification,safe reinforcement learning
AI 理解论文
溯源树
样例
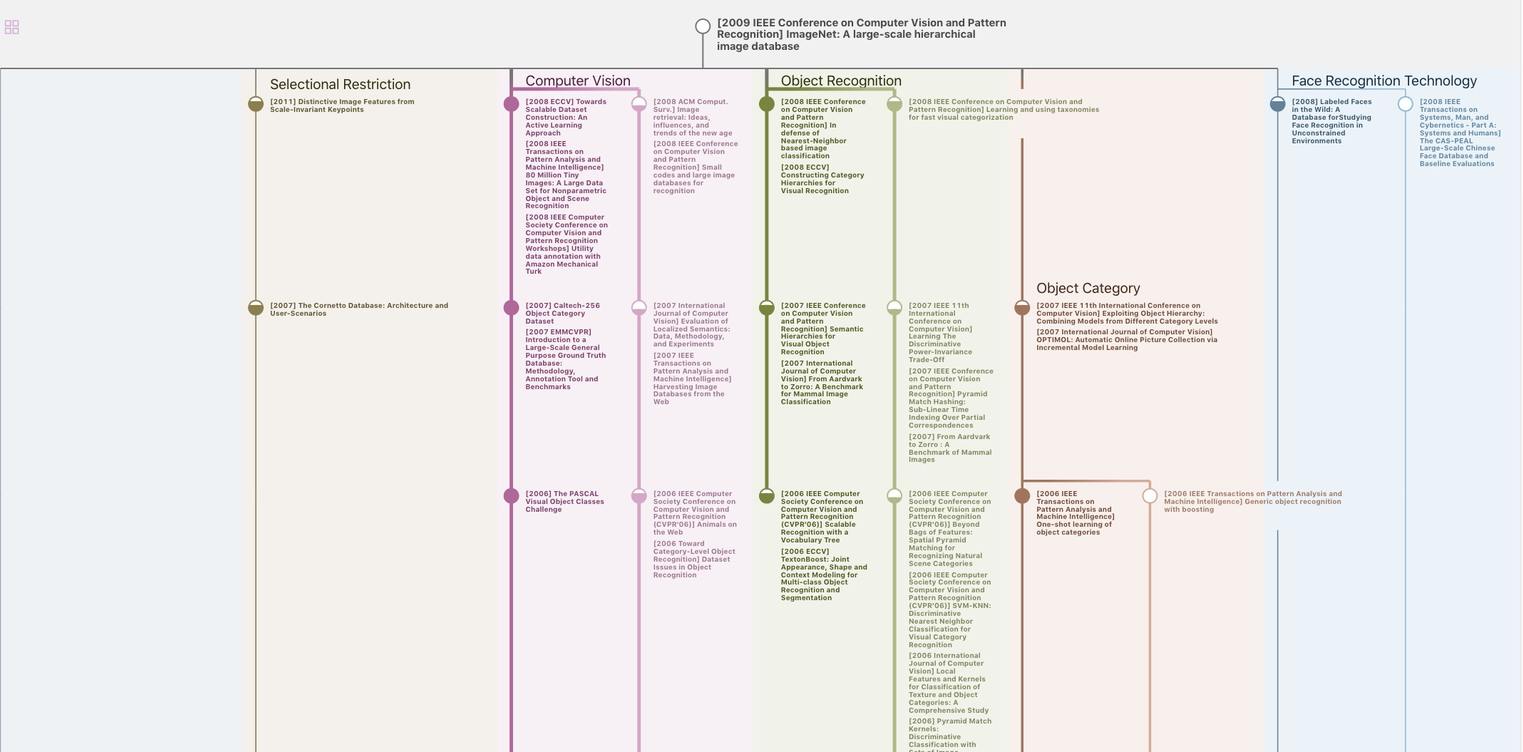
生成溯源树,研究论文发展脉络
Chat Paper
正在生成论文摘要