Adaptive Regularized Low-Rank Tensor Decomposition for Hyperspectral Image Denoising and Destriping
IEEE Transactions on Geoscience and Remote Sensing(2024)
摘要
Hyperspectral images (HSIs) are inevitably degraded by a mixture of various
types of noise, such as Gaussian noise, impulse noise, stripe noise, and dead
pixels, which greatly limits the subsequent applications. Although various
denoising methods have already been developed, accurately recovering the
spatial-spectral structure of HSIs remains a challenging problem to be
addressed. Furthermore, serious stripe noise, which is common in real HSIs, is
still not fully separated by the previous models. In this paper, we propose an
adaptive hyperLaplacian regularized low-rank tensor decomposition (LRTDAHL)
method for HSI denoising and destriping. On the one hand, the stripe noise is
separately modeled by the tensor decomposition, which can effectively encode
the spatial-spectral correlation of the stripe noise. On the other hand,
adaptive hyper-Laplacian spatial-spectral regularization is introduced to
represent the distribution structure of different HSI gradient data by
adaptively estimating the optimal hyper-Laplacian parameter, which can reduce
the spatial information loss and over-smoothing caused by the previous total
variation regularization. The proposed model is solved using the alternating
direction method of multipliers (ADMM) algorithm. Extensive simulation and
real-data experiments all demonstrate the effectiveness and superiority of the
proposed method.
更多查看译文
关键词
Hyperspectral images (HSIs),adaptive hyper-Laplacian,tensor completion,denoising and destriping
AI 理解论文
溯源树
样例
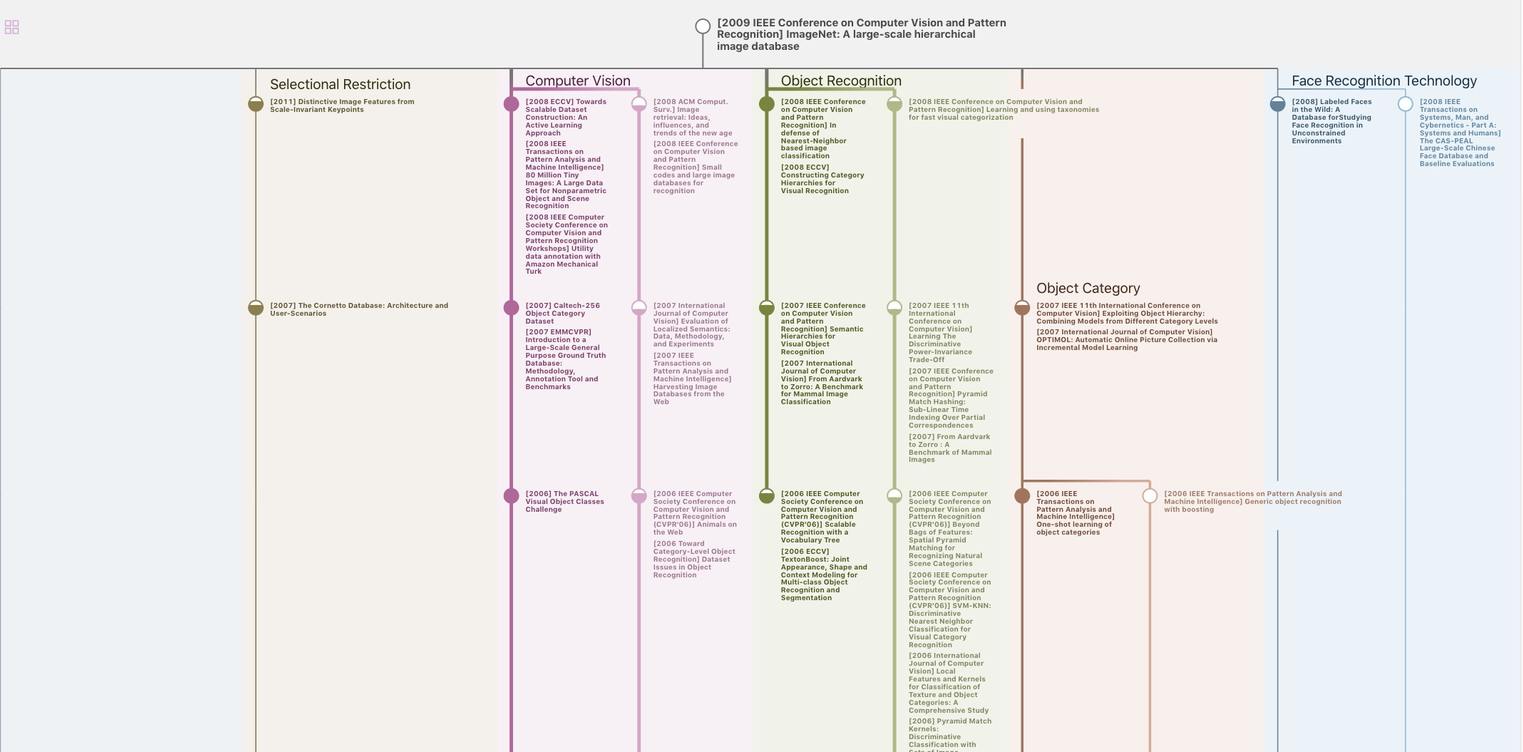
生成溯源树,研究论文发展脉络
Chat Paper
正在生成论文摘要