Designing for Appropriate Reliance: The Roles of AI Uncertainty Presentation, Initial User Decision, and User Demographics in AI-Assisted Decision-Making
CoRR(2024)
摘要
Appropriate reliance is critical to achieving synergistic human-AI
collaboration. For instance, when users over-rely on AI assistance, their
human-AI team performance is bounded by the model's capability. This work
studies how the presentation of model uncertainty may steer users'
decision-making toward fostering appropriate reliance. Our results demonstrate
that showing the calibrated model uncertainty alone is inadequate. Rather,
calibrating model uncertainty and presenting it in a frequency format allow
users to adjust their reliance accordingly and help reduce the effect of
confirmation bias on their decisions. Furthermore, the critical nature of our
skin cancer screening task skews participants' judgment, causing their reliance
to vary depending on their initial decision. Additionally, step-wise multiple
regression analyses revealed how user demographics such as age and familiarity
with probability and statistics influence human-AI collaborative
decision-making. We discuss the potential for model uncertainty presentation,
initial user decision, and user demographics to be incorporated in designing
personalized AI aids for appropriate reliance.
更多查看译文
AI 理解论文
溯源树
样例
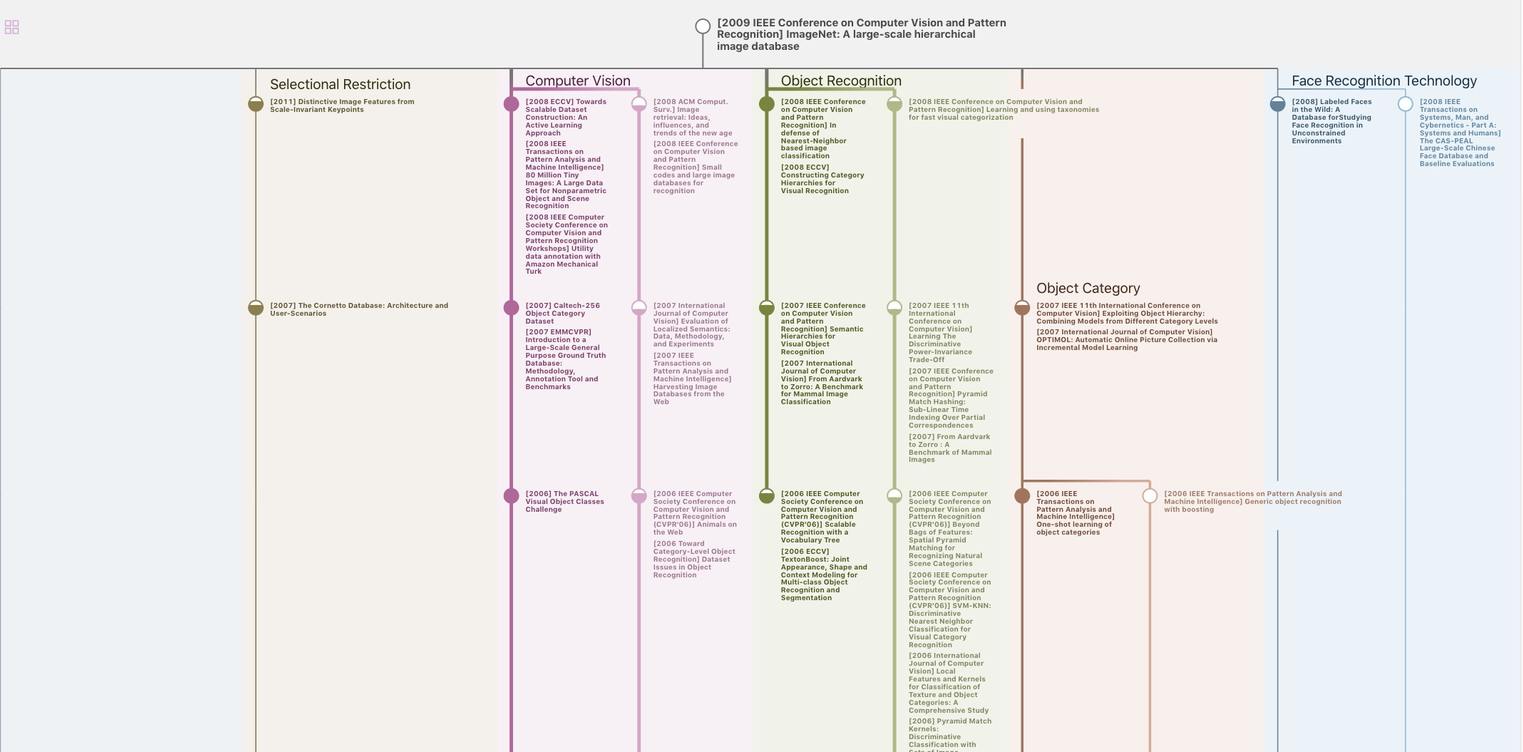
生成溯源树,研究论文发展脉络
Chat Paper
正在生成论文摘要