CoLafier: Collaborative Noisy Label Purifier With Local Intrinsic Dimensionality Guidance
CoRR(2024)
摘要
Deep neural networks (DNNs) have advanced many machine learning tasks, but their performance is often harmed by noisy labels in real-world data. Addressing this, we introduce CoLafier, a novel approach that uses Local Intrinsic Dimensionality (LID) for learning with noisy labels. CoLafier consists of two subnets: LID-dis and LID-gen. LID-dis is a specialized classifier. Trained with our uniquely crafted scheme, LID-dis consumes both a sample's features and its label to predict the label - which allows it to produce an enhanced internal representation. We observe that LID scores computed from this representation effectively distinguish between correct and incorrect labels across various noise scenarios. In contrast to LID-dis, LID-gen, functioning as a regular classifier, operates solely on the sample's features. During training, CoLafier utilizes two augmented views per instance to feed both subnets. CoLafier considers the LID scores from the two views as produced by LID-dis to assign weights in an adapted loss function for both subnets. Concurrently, LID-gen, serving as classifier, suggests pseudo-labels. LID-dis then processes these pseudo-labels along with two views to derive LID scores. Finally, these LID scores along with the differences in predictions from the two subnets guide the label update decisions. This dual-view and dual-subnet approach enhances the overall reliability of the framework. Upon completion of the training, we deploy the LID-gen subnet of CoLafier as the final classification model. CoLafier demonstrates improved prediction accuracy, surpassing existing methods, particularly under severe label noise. For more details, see the code at https://github.com/zdy93/CoLafier.
更多查看译文
AI 理解论文
溯源树
样例
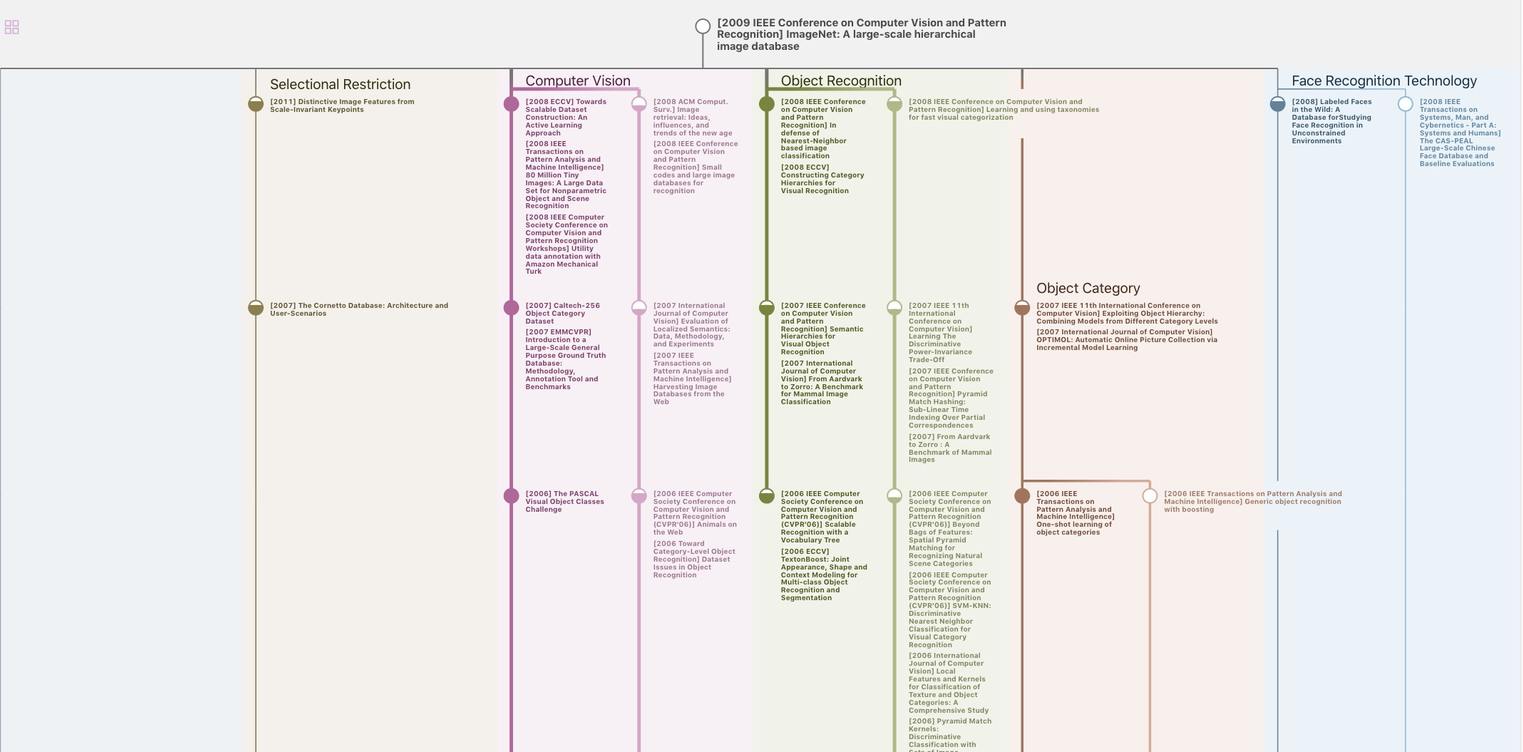
生成溯源树,研究论文发展脉络
Chat Paper
正在生成论文摘要