Neural network approach for shape-based euhedral pyrite identification in X-ray CT data with adversarial unsupervised domain adaptation
APPLIED COMPUTING AND GEOSCIENCES(2024)
摘要
We explore an attenuation and shape-based identification of euhedral pyrites in high -resolution X-ray Computed Tomography (XCT) data using deep neural networks. To deal with the scarcity of annotated data we generate a complementary training set of synthetic images. To investigate and address the domain gap between the synthetic and XCT data, several deep learning models, with and without domain adaption, are trained and compared. We find that a model trained on a small set of human annotations, while displaying over-fitting, can rival the human annotators. The unsupervised domain adaptation approaches are successful in bridging the domain gap, which significantly improves their performance. A domain-adapted model, trained on a dataset that fuses synthetic and real data, is the overall best-performing model. This highlights the possibility of using synthetic datasets for the application of deep learning in mineralogy.
更多查看译文
关键词
Mineral identification,Unsupervised domain adaptation,Deep convolutional neural network,Semantic segmentation,Euhedral pyrites
AI 理解论文
溯源树
样例
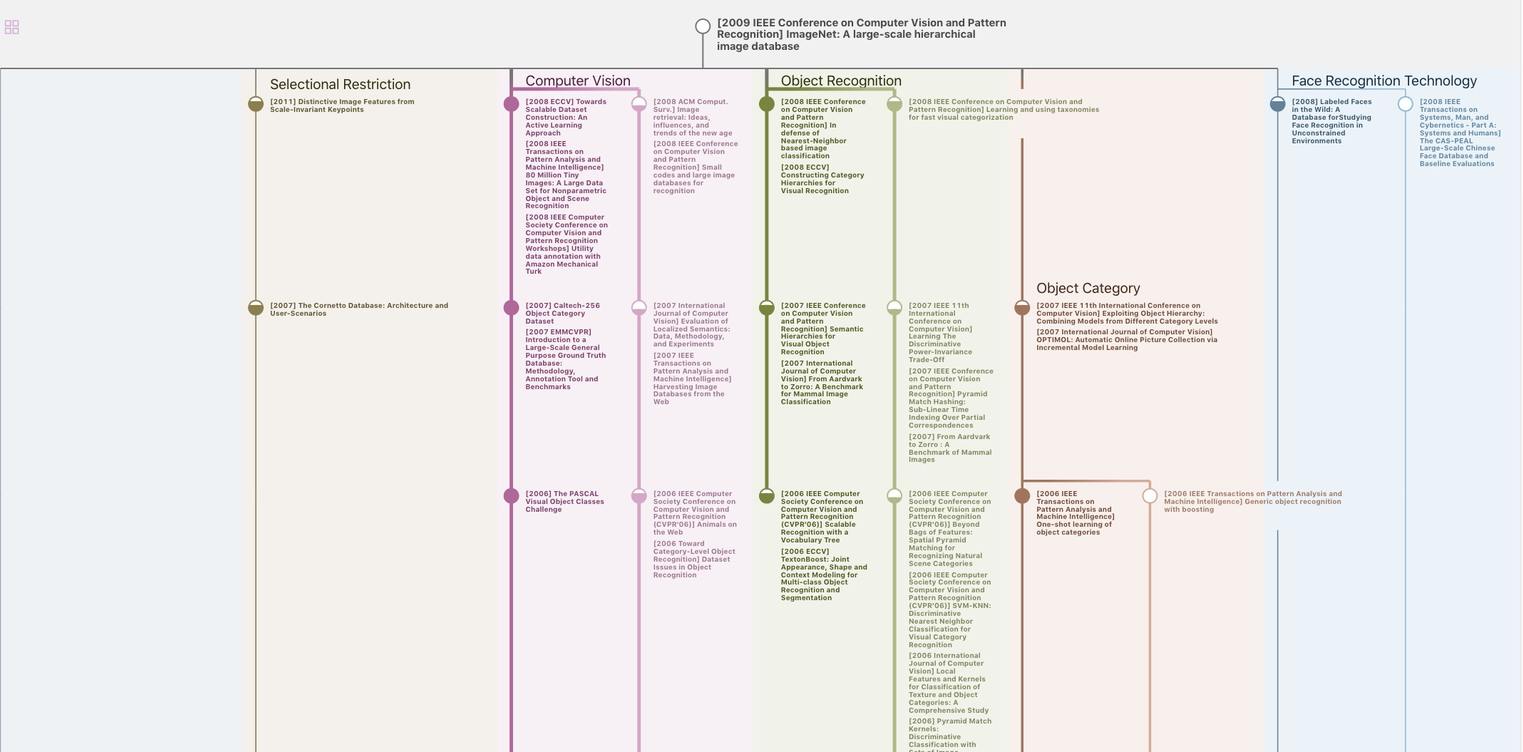
生成溯源树,研究论文发展脉络
Chat Paper
正在生成论文摘要