Intelligent fault identification in sample imbalance scenarios using robust low-rank matrix classifier with fuzzy weighting factor
APPLIED SOFT COMPUTING(2024)
摘要
Low-rank matrix learning techniques, especially support matrix machine (SMM) approach, have significantly altered mechanical fault diagnosis by efficiently uncovering correlations within matrix-form data. However, SMM exhibits limitations to eliminate the influence of redundant features and struggles with imbalanced classification scenarios, hampering the precise creation of SMM-based prediction model. To overcome these challenges, this paper introduces an innovative algorithm termed robust low-rank matrix classifier with fuzzy weighting factor (RLRMC-FWF). The core innovation of RLRMC-FWF lies in the integration of an inventive grouping elastic net within its framework. This novel inclusion empowers the control of essential attributes such as low-rank, sparsity, and grouping effects. Particularly noteworthy is grouping effects can autonomously identify relevant features without limitation of sample number. This greatly improving RLRMC-FWF's ability to extract information from minority class samples in imbalanced data. Furthermore, RLRMC-FWF introduces the incorporation of a fuzzy weighting factor, enabling the assignment of distinct weights to samples originating from different classes. This weighting mechanism refines the treatment of diverse sample classes, enhancing the adaptability and performance of RLRMC-FWF model. The effectiveness of the proposed method is validated through experimentation on two fault datasets associated with roller bearings. The experimental findings unequivocally highlight the exceptional performance of RLRMC-FWF within the domain of roller bearing fault diagnosis.
更多查看译文
关键词
Support matrix machine,Low -rank matrix learning,Fuzzy membership function,Data imbalance,Fault identification
AI 理解论文
溯源树
样例
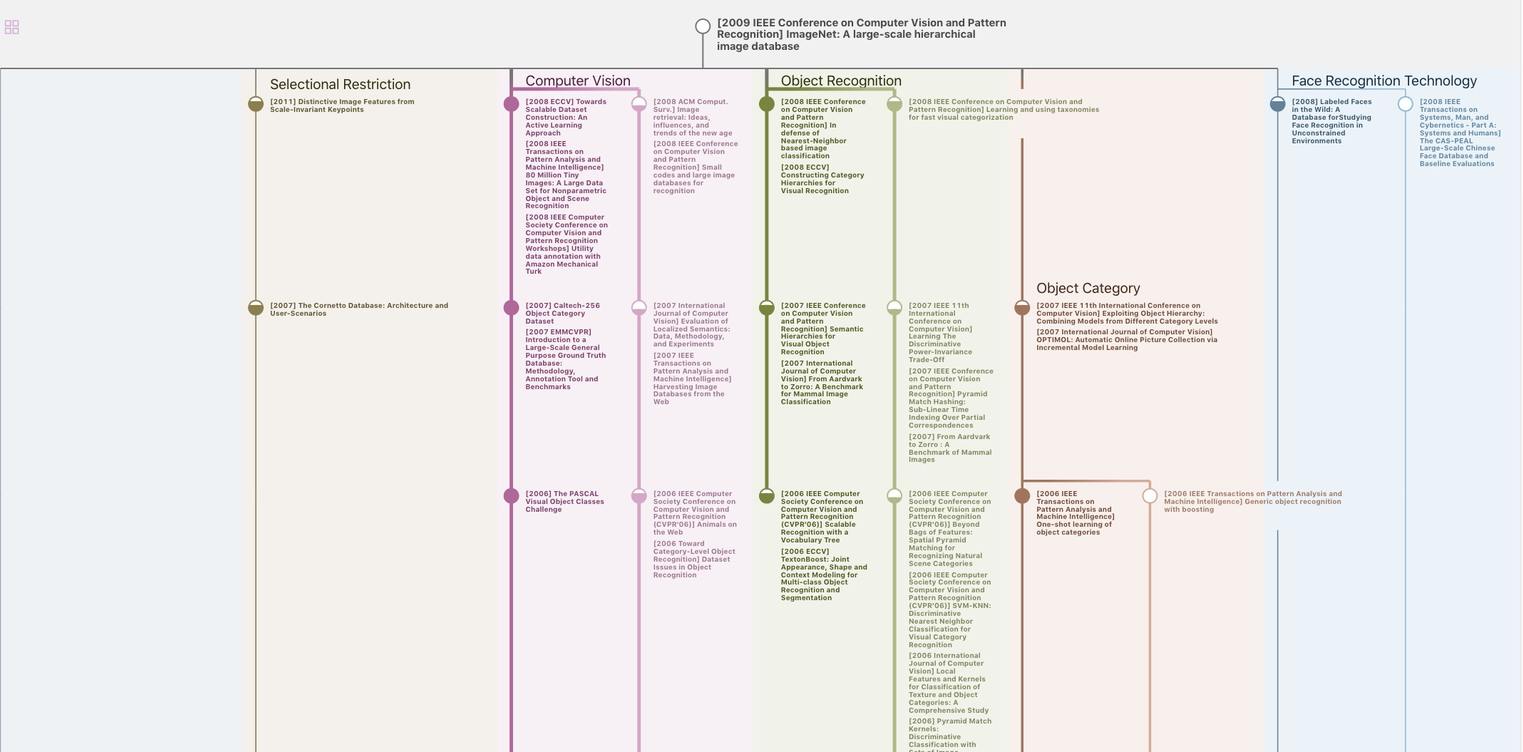
生成溯源树,研究论文发展脉络
Chat Paper
正在生成论文摘要