High-speed emerging memories for AI hardware accelerators
Nature Reviews Electrical Engineering(2024)
摘要
Applications of artificial intelligence (AI) necessitate AI hardware accelerators able to efficiently process data-intensive and computation-intensive AI workloads. AI accelerators require two types of memory: the weight memory that stores the parameters of the AI models and the buffer memory that stores the intermediate input or output data when computing a portion of the AI models. In this Review, we present the recent progress in the emerging high-speed memory for AI hardware accelerators and survey the technologies enabling the global buffer memory in digital systolic-array architectures. Beyond conventional static random-access memory (SRAM), we highlight the following device candidates: capacitorless gain cell-based embedded dynamic random-access memories (eDRAMs), ferroelectric memories, spin-transfer torque magnetic random-access memory (STT-MRAM) and spin-orbit torque magnetic random-access memory (SOT-MRAM). We then summarize the research advances in the industrial development and the technological challenges in buffer memory applications. Finally, we present a systematic benchmarking analysis on a tensor processing unit (TPU)-like AI accelerator in the edge and in the cloud and evaluate the use of these emerging memories.
更多查看译文
AI 理解论文
溯源树
样例
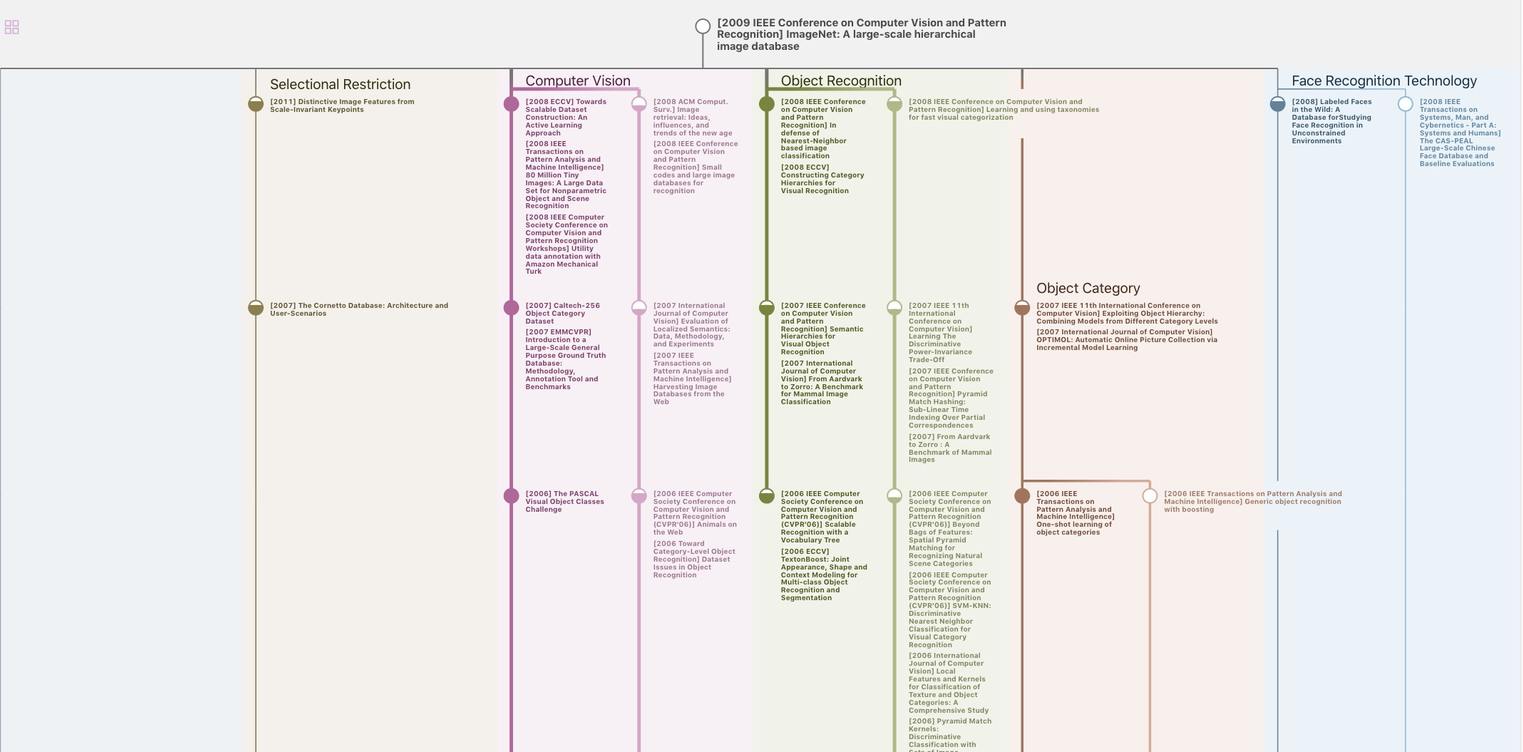
生成溯源树,研究论文发展脉络
Chat Paper
正在生成论文摘要