Spectral-Spatial-Language Fusion Network for Hyperspectral, LiDAR, and Text Data Classification
IEEE TRANSACTIONS ON GEOSCIENCE AND REMOTE SENSING(2024)
摘要
The fusion classification of hyperspectral image (HSI) and light detection and ranging (LiDAR) data has gained widespread attention because of its ability to obtain more comprehensive spatial and spectral information. However, the heterogeneous gap between HSI and LiDAR data also adversely affects the classification performance. Despite the excellent performance of traditional multimodal fusion classification models, language information containing much linguistic priori knowledge to enrich visual representations needs to be addressed. Therefore, we design a spectral-spatial-language fusion network (S2LFNet), which can fuse visual and language features to broaden the semantic space using linguistic priori knowledge commonly shared between spectral features and spatial features. First, we propose a dual-channel cascaded image fusion encoder (DCIFencoder) for visual feature extraction and progressive feature fusion of different levels for HSI and LiDAR data. Then, three aspects of text data are designed to extract linguistic priori knowledge using the text encoder. Finally, contrastive learning is utilized to construct a unified semantic space, and spectral-spatial-language fusion features are obtained for classification tasks. We evaluate the classification performance of the proposed S2LFNet on three datasets through extensive experiments, and the results show that it outperforms the state-of-the-art fusion classification methods.
更多查看译文
关键词
Contrastive learning,hyperspectral image (HSI) classification,image classification,light detection and ranging (LiDAR),multimodal
AI 理解论文
溯源树
样例
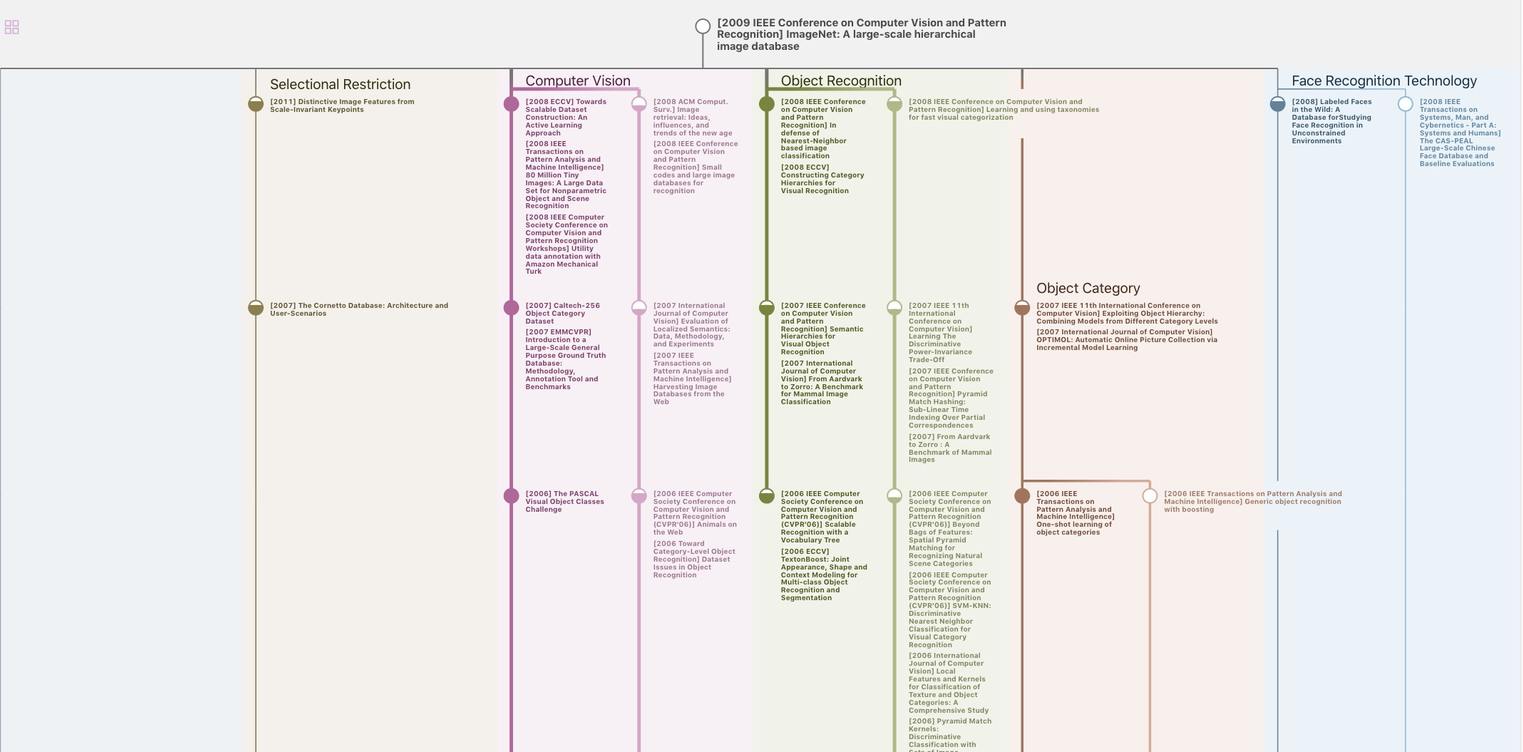
生成溯源树,研究论文发展脉络
Chat Paper
正在生成论文摘要