Audio Super-Resolution With Robust Speech Representation Learning of Masked Autoencoder
IEEE-ACM TRANSACTIONS ON AUDIO SPEECH AND LANGUAGE PROCESSING(2024)
摘要
This paper proposes Fre-Painter, a high-fidelity audio super-resolution system that utilizes robust speech representation learning with various masking strategies. Recently, masked autoencoders have been found to be beneficial in learning robust representations of audio for speech classification tasks. Following these studies, we leverage these representations and investigate several masking strategies for neural audio super-resolution. In this paper, we propose an upper-band masking strategy with the initialization of the mask token, which is simple but efficient for audio super-resolution. Furthermore, we propose a mix-ratio masking strategy that makes the model robust for input speech with various sampling rates. For practical applicability, we extend Fre-Painter to a text-to-speech system, which synthesizes high-resolution speech using low-resolution speech data. The experimental results demonstrate that Fre-Painter outperforms other neural audio super-resolution models.
更多查看译文
关键词
Superresolution,Task analysis,Speech processing,Self-supervised learning,Computational modeling,Decoding,Training,Audio super-resolution,bandwidth extension,self-supervised learning,masked autoencoder,audio synthesis
AI 理解论文
溯源树
样例
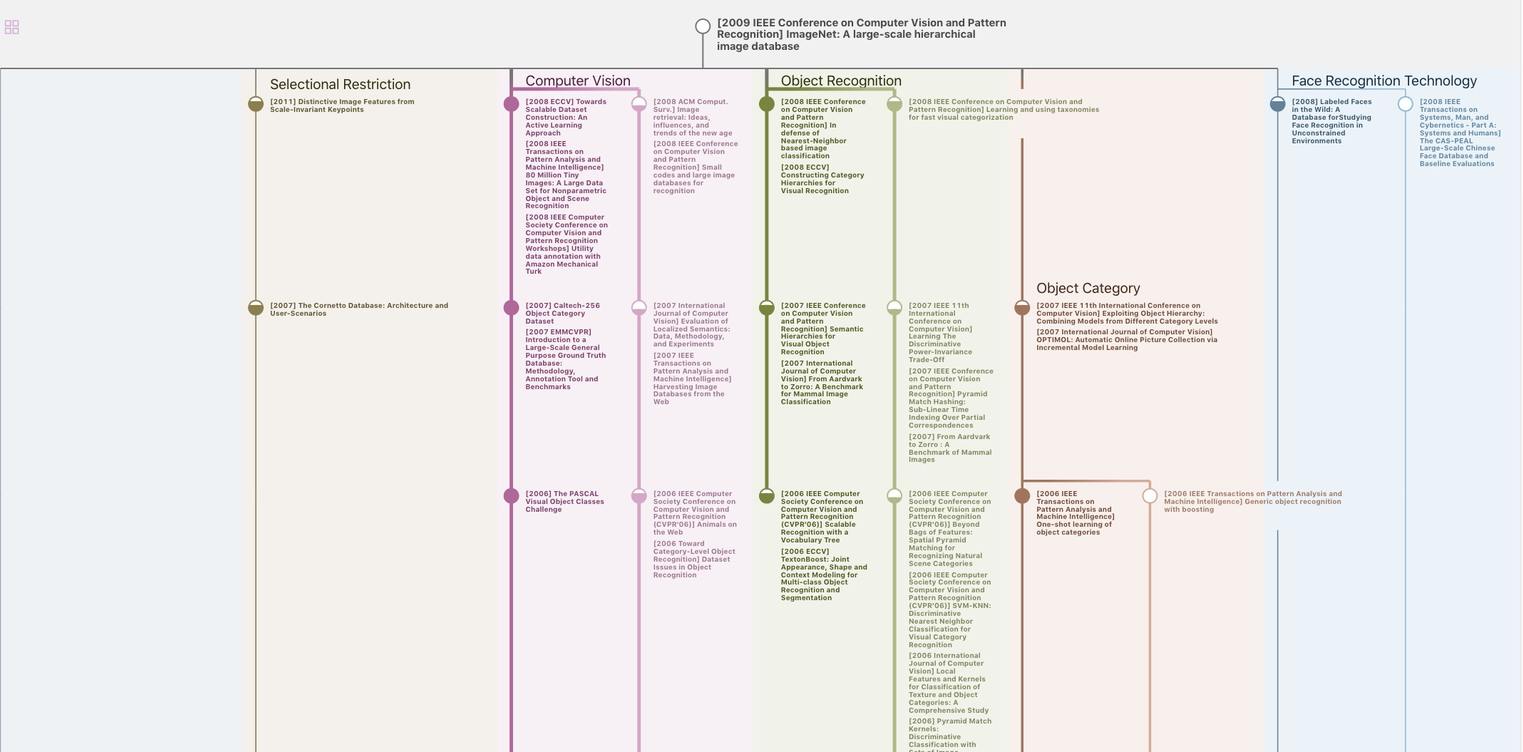
生成溯源树,研究论文发展脉络
Chat Paper
正在生成论文摘要