Contrastive Personalized Exercise Recommendation With Reinforcement Learning
IEEE TRANSACTIONS ON LEARNING TECHNOLOGIES(2024)
摘要
Personalized exercise recommendation is a challenging task in the field of artificial intelligence in education due to several problems. First, the mainstream approaches focus more on the exercises that students have not mastered, while overlooking their long-term needs during the learning process. Second, it is difficult to capture students' knowledge states caused by sparse interactions with exercises. Moreover, most recommendation methods are dedicated to the performance of the recommendation w.r.t. accuracy, disregarding the students' learning ability. We introduce a new framework called contrastive personalized exercise recommendation with reinforcement learning (RCL4ER) to tackle these issues. Our framework augments the standard recommendation model with an output layer of self-supervised learning and reinforcement learning. The reinforcement allows the supervised layer to focus on specific rewards, acting as a regularizer. The self-supervised layer provides a powerful signal for parameter updating. Three data augmentation methods are used to provide additional data, which are leveraged to conduct contrastive learning and incorporated into reinforcement learning as supplementary information. In addition, we adopt a trained deep knowledge tracing model to capture the changes in students' knowledge states, so as to establish a dynamic reward mechanism. Experiments of our framework based on four recommendation backbones on several public datasets demonstrate the effectiveness of our RCL4ER, which successfully promotes students' capacity and improves the recommendation performance.
更多查看译文
关键词
Contrastive learning,knowledge tracing,q-learning,recommendation,reinforcement learning
AI 理解论文
溯源树
样例
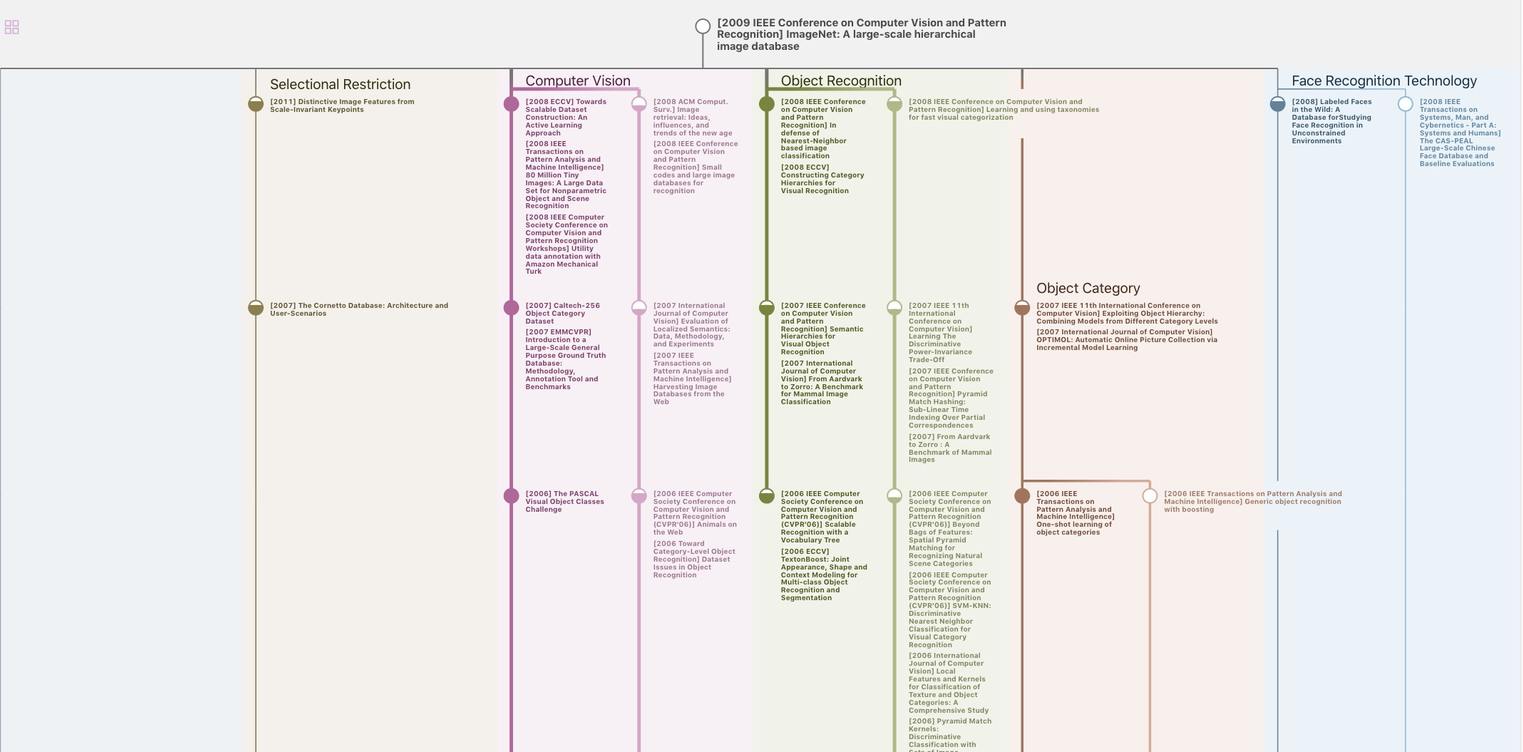
生成溯源树,研究论文发展脉络
Chat Paper
正在生成论文摘要