Manifold-driven decomposition for adversarial robustness
FRONTIERS IN COMPUTER SCIENCE(2024)
摘要
The adversarial risk of a machine learning model has been widely studied. Most previous studies assume that the data lie in the whole ambient space. We propose to take a new angle and take the manifold assumption into consideration. Assuming data lie in a manifold, we investigate two new types of adversarial risk, the normal adversarial risk due to perturbation along normal direction and the in-manifold adversarial risk due to perturbation within the manifold. We prove that the classic adversarial risk can be bounded from both sides using the normal and in-manifold adversarial risks. We also show a surprisingly pessimistic case that the standard adversarial risk can be non-zero even when both normal and in-manifold adversarial risks are zero. We finalize the study with empirical studies supporting our theoretical results. Our results suggest the possibility of improving the robustness of a classifier without sacrificing model accuracy, by only focusing on the normal adversarial risk.
更多查看译文
关键词
robustness,adversarial attack,manifold,topological analysis of network,generalization
AI 理解论文
溯源树
样例
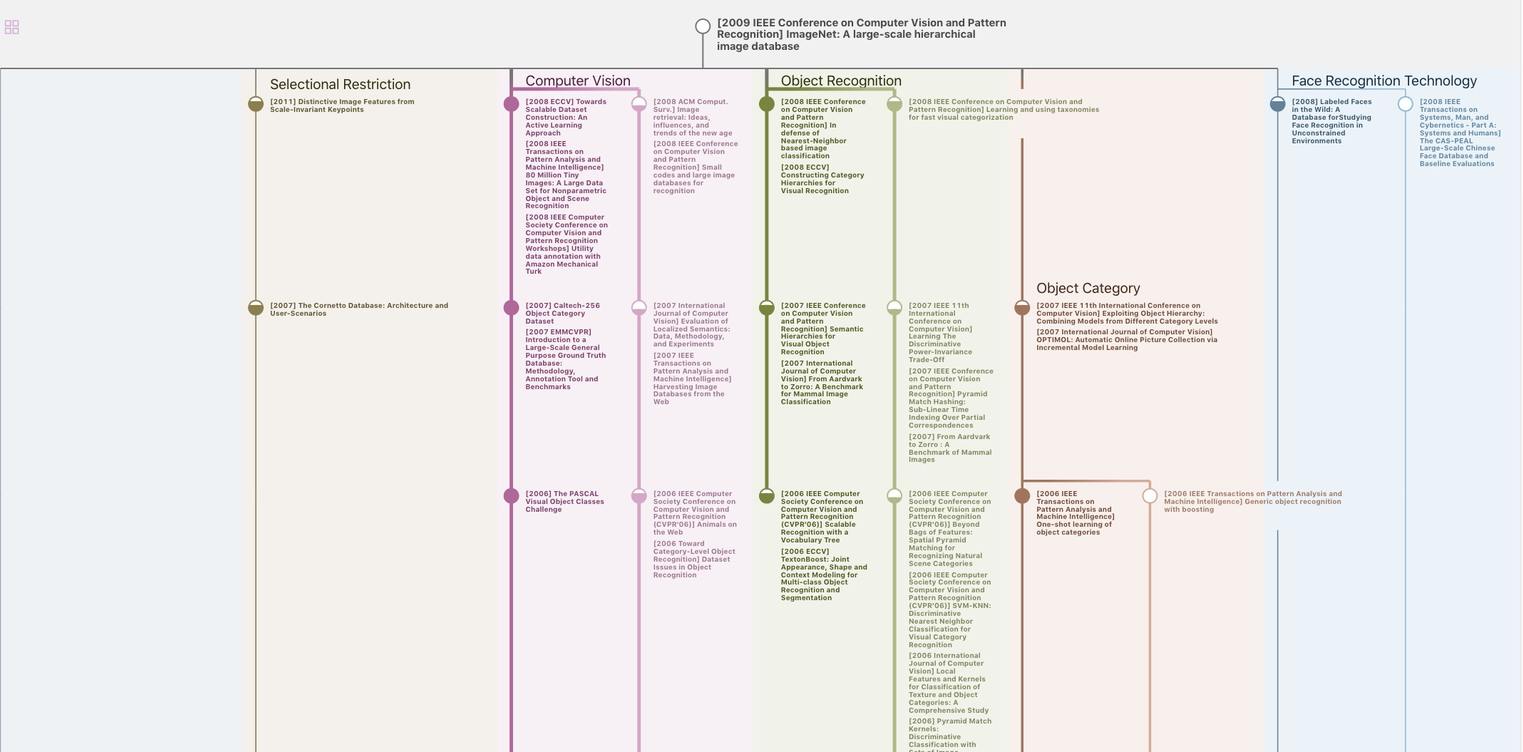
生成溯源树,研究论文发展脉络
Chat Paper
正在生成论文摘要