Adapting bandit algorithms for settings with sequentially available arms
ENGINEERING APPLICATIONS OF ARTIFICIAL INTELLIGENCE(2024)
摘要
Many real-world applications involve a sequential decision-making process where the options presented simultaneously. However, other applications, such as, Internet campaign management and environmental monitoring, the available options are presented sequentially to the decision-maker who, at each time, is asked to select the proposed option or not. This scenario is defined as the Sequential Pull/No-Pull setting The present study aims at developing a meta-algorithm, namely Sequential Pull/No-pull for MAB (Seq), to adapt any classical MAB (Multi-Armed Bandit) policy for this setting both in the case of regret minimization (RM) and best-arm identification (BAI) problems. This is achieved by exploting the sequential nature of the these settings allowing to select multiple arms and gather more information compared to classical policies. The proposed Seq meta-algorithm provides the same theoretical guarantees as the MAB policy employed, but was shown to provide improved performance compared to several classical MAB policies in RM and BAI problems employing real-world data. In particular, in the RM scenario regarding Internet advertising optimization, Seq-adapted algorithm resulted, on average, in approximate to 10% lower regret during the whole time horizon than using classical MAB policies. When tested in a BAI problem involving the identification of the time of the day characterized by the highest concentration of pollutants in a water monitoring scenario, Seq identified the correct time in less than 4 days and 28 measurement.
更多查看译文
关键词
Online learning,Multi-armed Bandit,Regret minimization,Best-arm identification
AI 理解论文
溯源树
样例
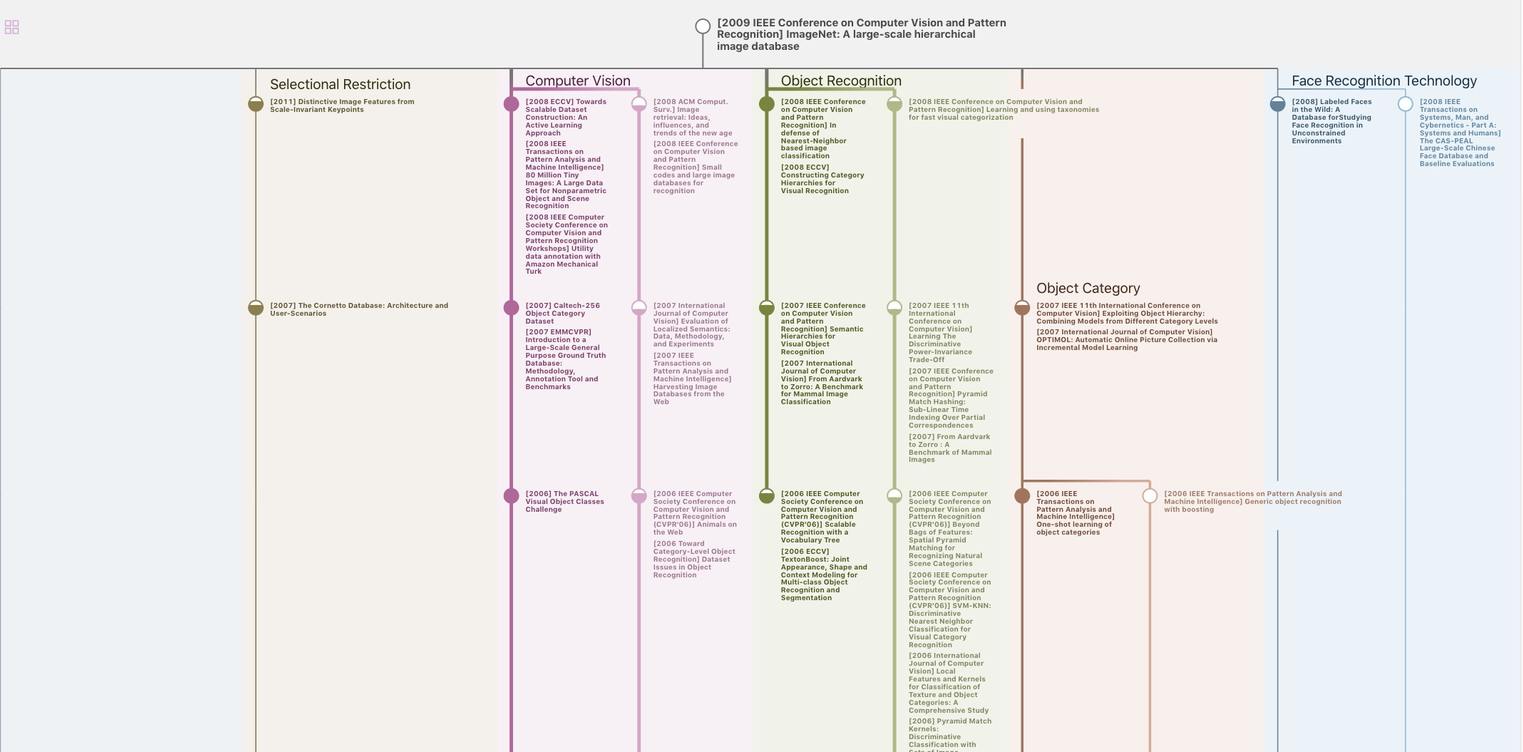
生成溯源树,研究论文发展脉络
Chat Paper
正在生成论文摘要