Optimal bipartite graph matching-based goal selection for policy-based hindsight learning
Neurocomputing(2024)
摘要
The sparse reward problem stands as a significant challenge in the field of reinforcement learning. Hindsight Experience Replay (HER) addresses this by goal relabeling, allowing the agent to learn from unsuccessful experiences. Some studies combine policy gradient methods with HER, resulting in policy-based hindsight learning algorithms. However, Policy-based hindsight learning involves the use of importance sampling, where the distribution of hindsight goals and the distribution of desired goals contribute to the computation of importance weights. When there is a significant difference between the two distributions, importance weights may become skewed, thereby impacting the evaluation of the policy and leading to suboptimal policies. To address this, we propose modeling the goal selection as an optimization problem for distribution matching. After we augment the original desired goals using Kernel Density Estimation (KDE), we further convert the optimization problem for distribution matching into a bipartite graph matching problem that minimizes the sum of weights. Our optimal bipartite graph matching-based hindsight goal selection method can select hindsight goals that are the most closely aligned with the original goals. Experimental results show that algorithms combined with the optimal bipartite graph matching-based hindsight goal selection outperform the original algorithms. Visualizations also demonstrate the superiority of our method in selecting hindsight goals.
更多查看译文
关键词
Goal-conditioned reinforcement learning,Sparse rewards,Goal relabeling,Policy-based hindsight learning
AI 理解论文
溯源树
样例
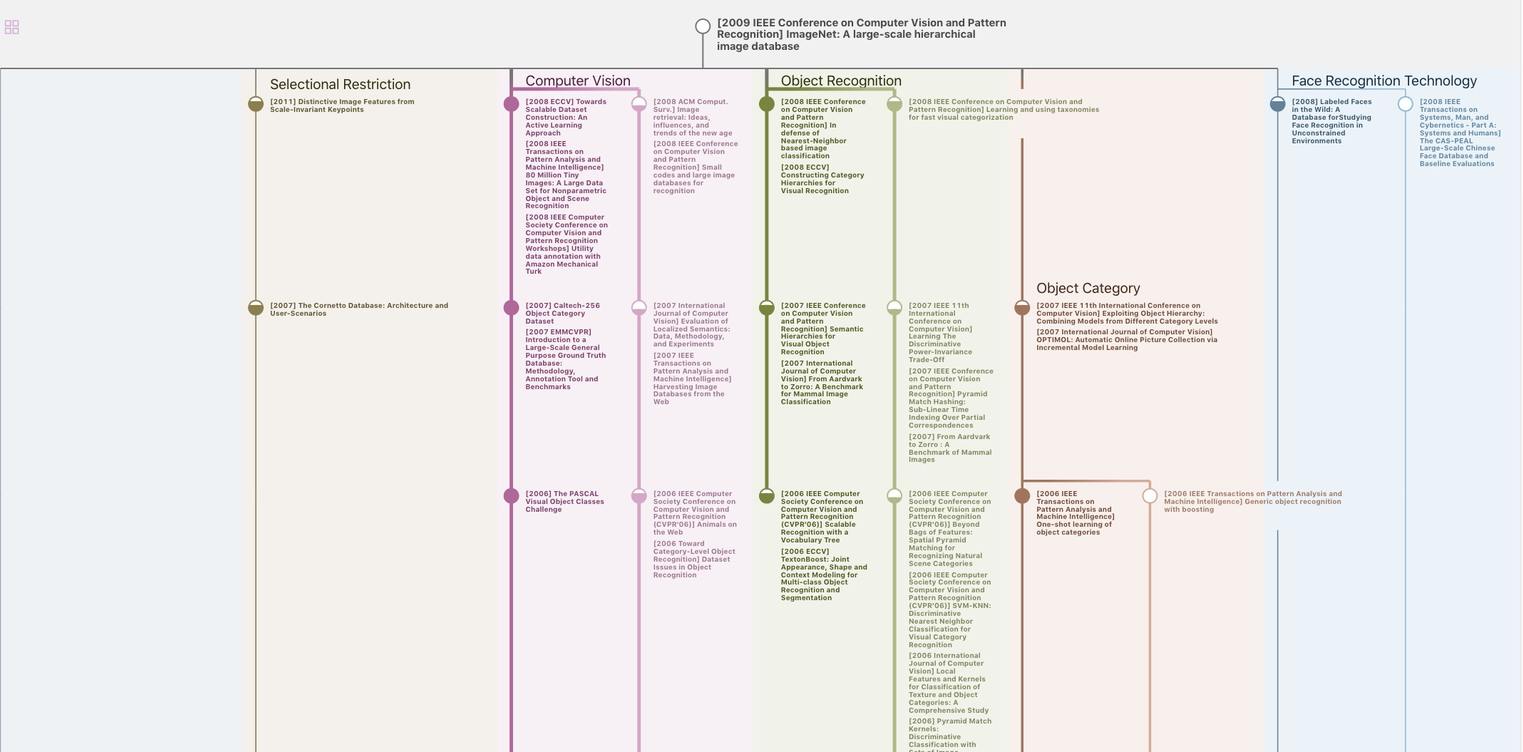
生成溯源树,研究论文发展脉络
Chat Paper
正在生成论文摘要