Unagi: Deep Generative Model for Deciphering Cellular Dynamics and In-Silico Drug Discovery in Complex Diseases.
Research square(2023)
摘要
Human diseases are characterized by intricate cellular dynamics. Single-cell sequencing provides critical insights, yet a persistent gap remains in computational tools for detailed disease progression analysis and targeted in-silico drug interventions. We introduce UNAGI, a deep generative neural network tailored to analyze time-series single-cell transcriptomic data. This innovative tool captures the complex cellular dynamics underlying disease progression, enhancing drug perturbation modeling and discovery. When applied to a dataset from patients with Idiopathic Pulmonary Fibrosis (IPF), UNAGI adeptly learns disease-informed cell embeddings that sharpen our understanding of disease progression, leading to the identification of potential therapeutic drug candidates. Validation via proteomics reveals the accuracy of UNAGI's cellular dynamics analyses, and the use of the Fibrotic Cocktail treated human Precision-cut Lung Slices confirms UNAGI's predictions that Nifedipine, an antihypertensive drug, may have antifibrotic effects on human tissues. UNAGI's versatility extends to other diseases, including a COVID dataset, demonstrating adaptability and confirming its broader applicability in decoding complex cellular dynamics beyond IPF, amplifying its utility in the quest for therapeutic solutions across diverse pathological landscapes.
更多查看译文
AI 理解论文
溯源树
样例
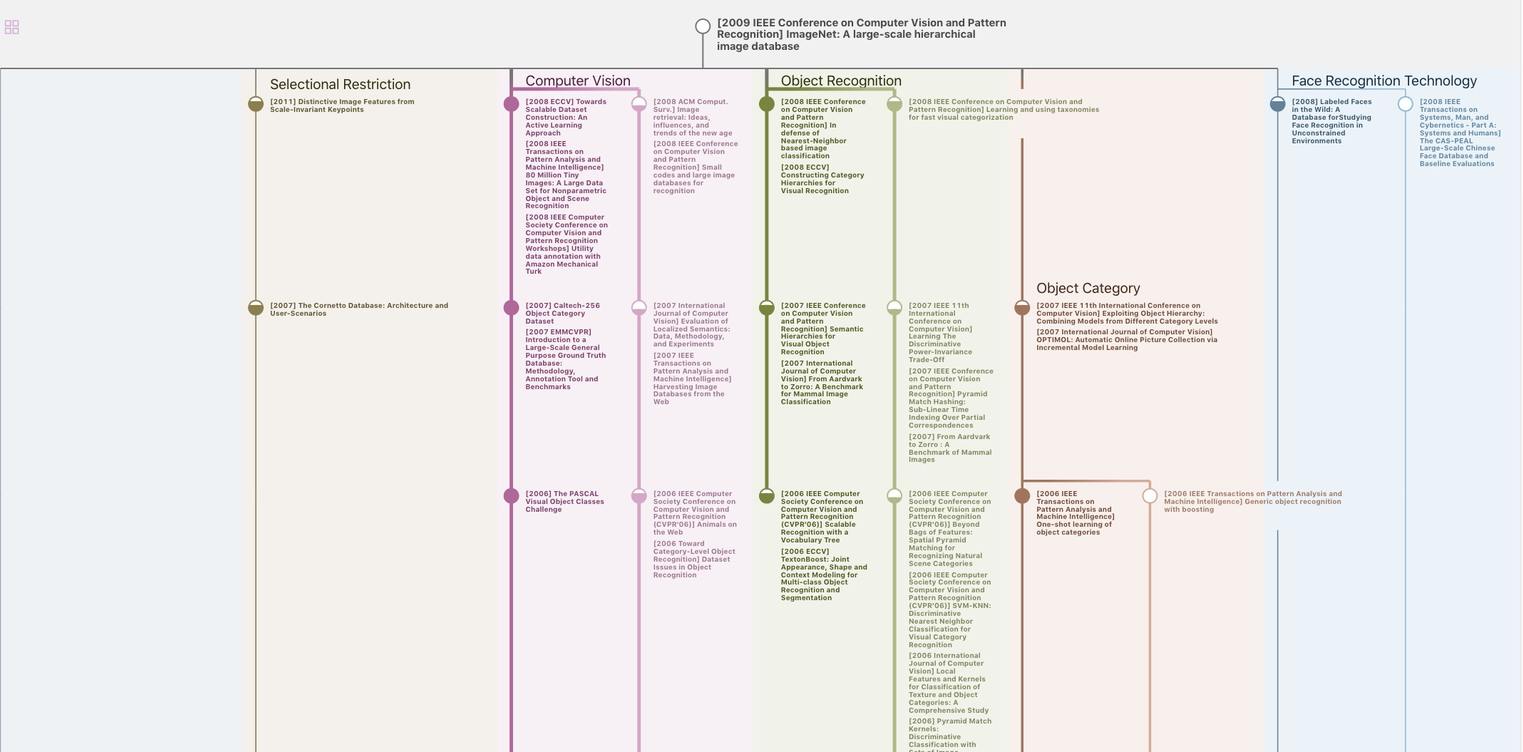
生成溯源树,研究论文发展脉络
Chat Paper
正在生成论文摘要