Guiding discovery of protein sequence-structure-function modeling
BIOINFORMATICS(2024)
摘要
Motivation Protein engineering techniques are key in designing novel catalysts for a wide range of reactions. Although approaches vary in their exploration of the sequence-structure-function paradigm, they are often hampered by the labor-intensive steps of protein expression and screening. In this work, we describe the development and testing of a high-throughput in silico sequence-structure-function pipeline using AlphaFold2 and fast Fourier transform docking that is benchmarked with enantioselectivity and reactivity predictions for an ancestral sequence library of fungal flavin-dependent monooxygenases. Results The predicted enantioselectivities and reactivities correlate well with previously described screens of an experimentally available subset of these proteins and capture known changes in enantioselectivity across the phylogenetic tree representing ancestorial proteins from this family. With this pipeline established as our functional screen, we apply ensemble decision tree models and explainable AI techniques to build sequence-function models and extract critical residues within the binding site and the second-sphere residues around this site. We demonstrate that the top-identified key residues in the control of enantioselectivity and reactivity correspond to experimentally verified residues. The in silico sequence-to-function pipeline serves as an accelerated framework to inform protein engineering efforts from vast informative sequence landscapes contained in protein families, ancestral resurrects, and directed evolution campaigns. Availability Jupyter notebooks detailing the sequence-structure-function pipeline are available at https://github.com/BrooksResearchGroup-UM/seq_struct_func
更多查看译文
关键词
protein,discovery,sequence-structure-function
AI 理解论文
溯源树
样例
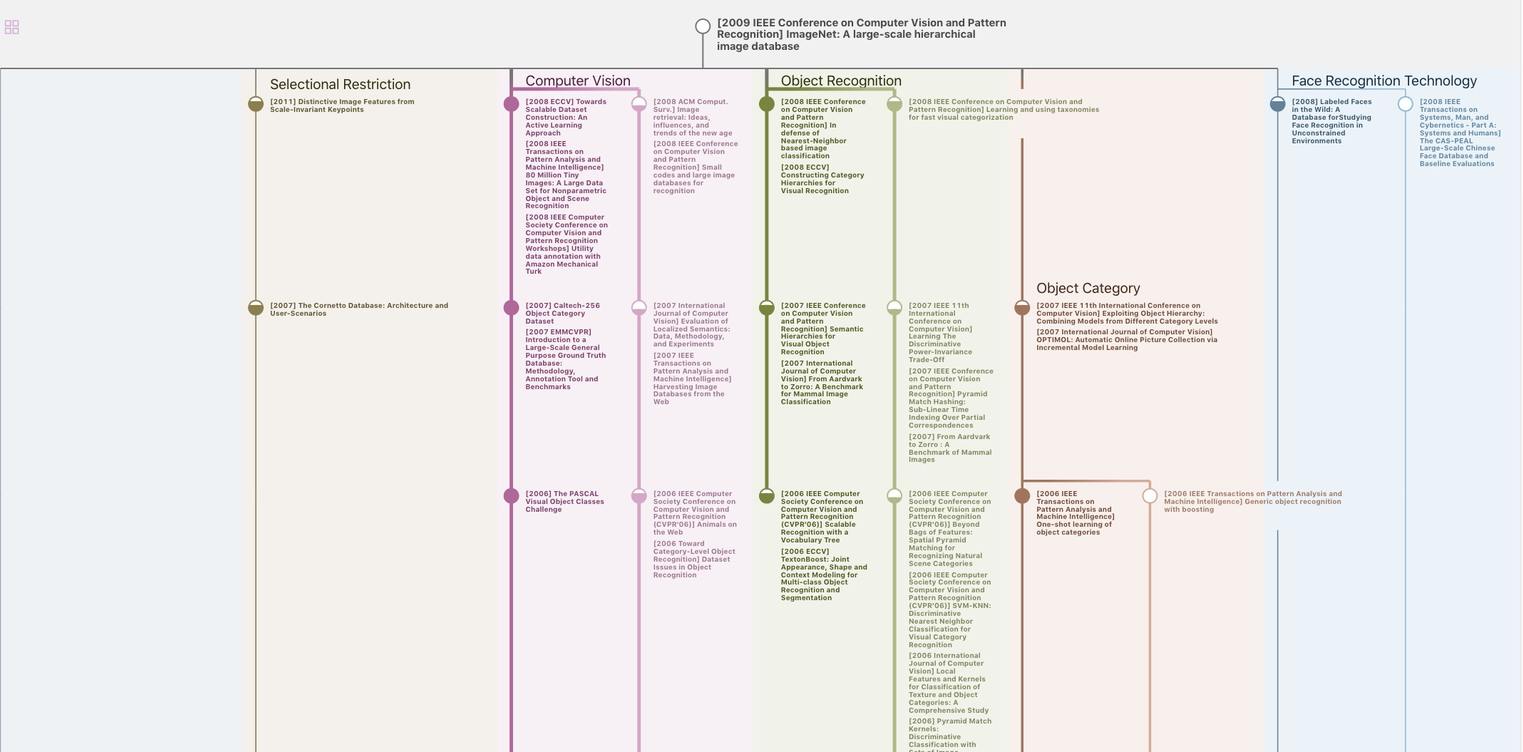
生成溯源树,研究论文发展脉络
Chat Paper
正在生成论文摘要