Machine learning interpretable models of cell mechanics from protein images
CELL(2024)
摘要
Cellular form and function emerge from complex mechanochemical systems within the cytoplasm. Currently, no systematic strategy exists to infer large-scale physical properties of a cell from its molecular components. This is an obstacle to understanding processes such as cell adhesion and migration. Here, we develop a data -driven modeling pipeline to learn the mechanical behavior of adherent cells. We first train neural networks to predict cellular forces from images of cytoskeletal proteins. Strikingly, experimental images of a single focal adhesion (FA) protein, such as zyxin, are sufficient to predict forces and can generalize to unseen biological regimes. Using this observation, we develop two approaches-one constrained by physics and the other agnostic-to construct data -driven continuum models of cellular forces. Both reveal how cellular forces are encoded by two distinct length scales. Beyond adherent cell mechanics, our work serves as a case study for integrating neural networks into predictive models for cell biology.
更多查看译文
关键词
machine learning,traction force microscopy,cell mechanics,focal adhesions,biophysics,biomechanics,zyxin,neural network
AI 理解论文
溯源树
样例
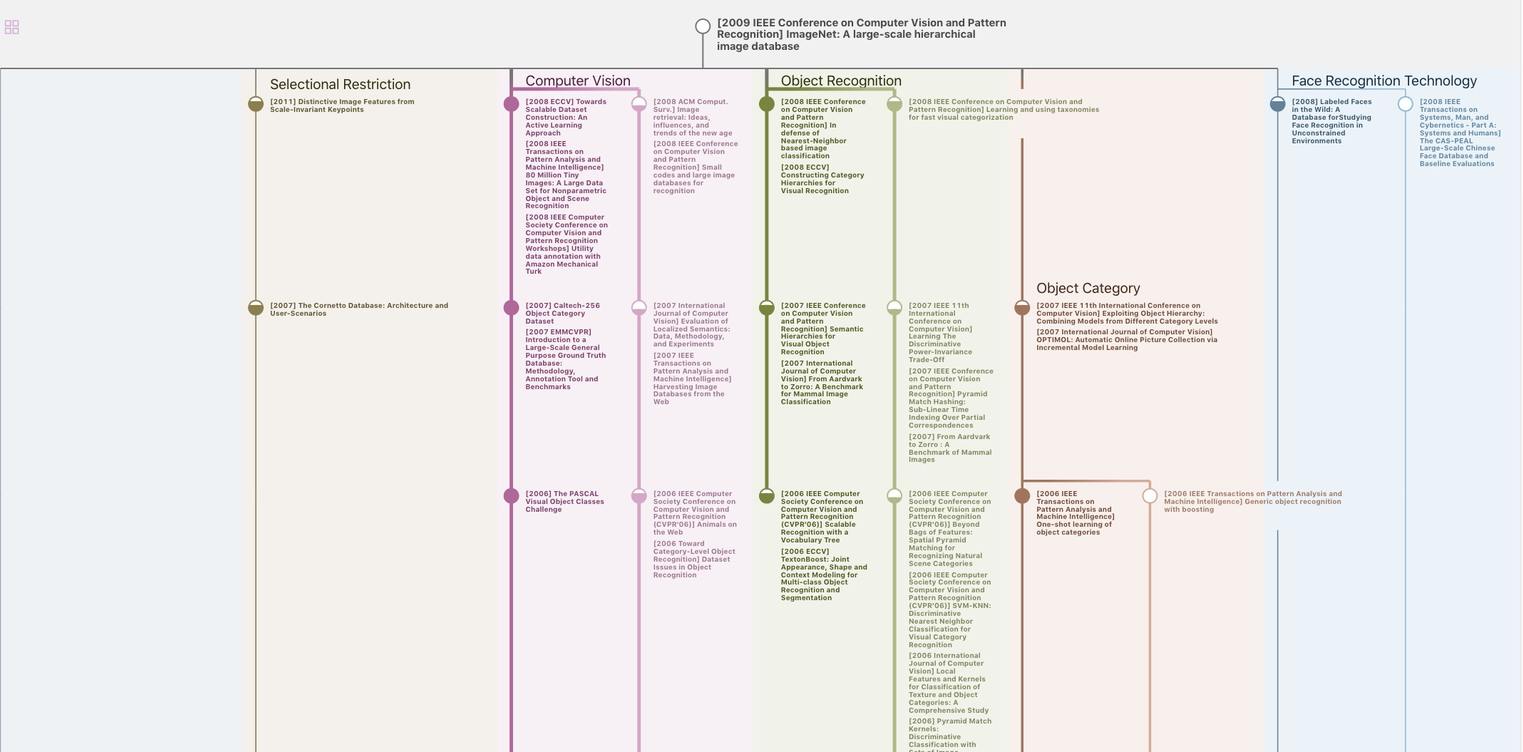
生成溯源树,研究论文发展脉络
Chat Paper
正在生成论文摘要