Capsule Networks With Residual Pose Routing
IEEE TRANSACTIONS ON NEURAL NETWORKS AND LEARNING SYSTEMS(2024)
摘要
Capsule networks (CapsNets) have been known difficult to develop a deeper architecture, which is desirable for high performance in the deep learning era, due to the complex capsule routing algorithms. In this article, we present a simple yet effective capsule routing algorithm, which is presented by a residual pose routing. Specifically, the higher-layer capsule pose is achieved by an identity mapping on the adjacently lower-layer capsule pose. Such simple residual pose routing has two advantages: 1) reducing the routing computation complexity and 2) avoiding gradient vanishing due to its residual learning framework. On top of that, we explicitly reformulate the capsule layers by building a residual pose block. Stacking multiple such blocks results in a deep residual CapsNets (ResCaps) with a ResNet-like architecture. Results on MNIST, AffNIST, SmallNORB, and CIFAR-10/100 show the effectiveness of ResCaps for image classification. Furthermore, we successfully extend our residual pose routing to large-scale real-world applications, including 3-D object reconstruction and classification, and 2-D saliency dense prediction.
更多查看译文
关键词
3-D point cloud,capsule network (CapsNet),part-whole,residual routing,salient object detection
AI 理解论文
溯源树
样例
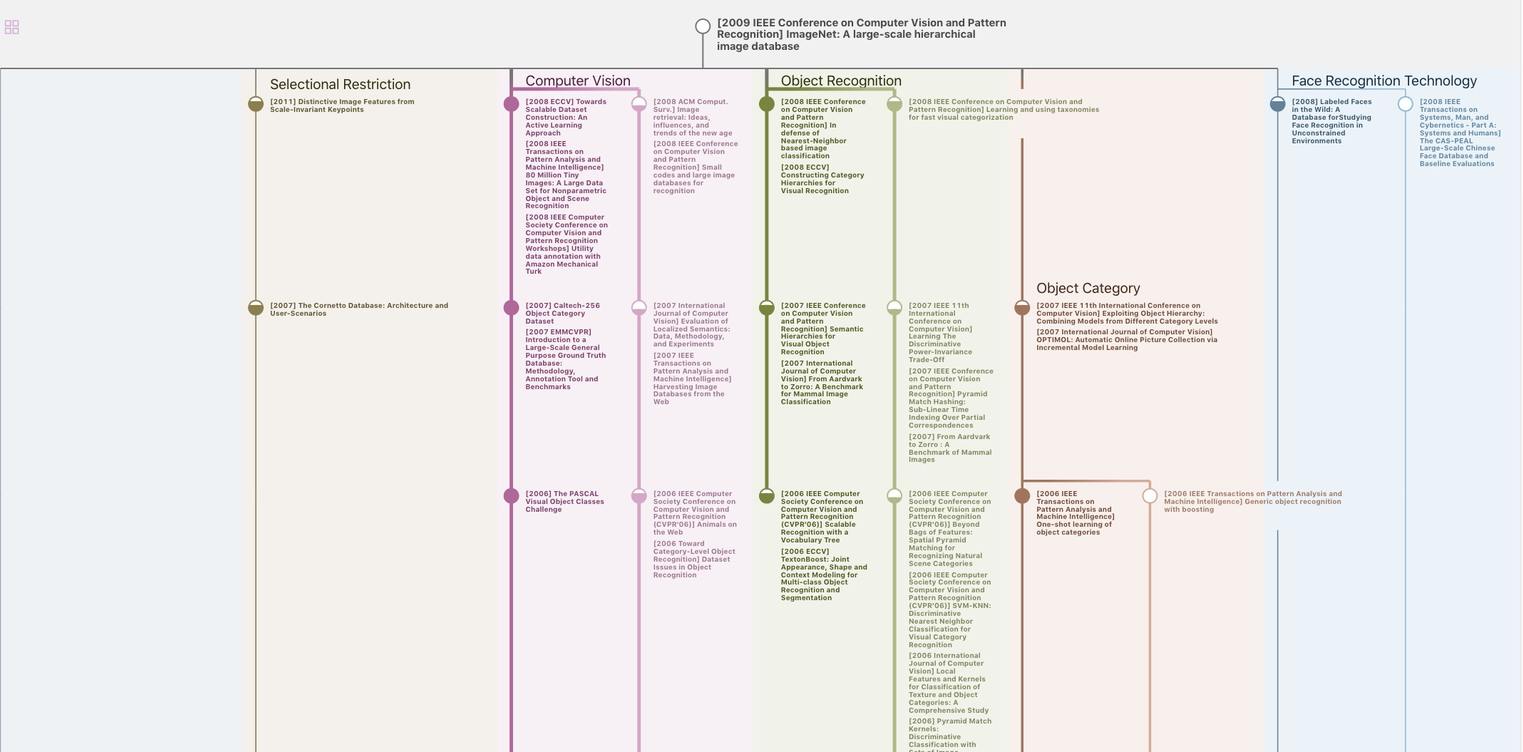
生成溯源树,研究论文发展脉络
Chat Paper
正在生成论文摘要