Reducing measurement costs by recycling the Hessian in adaptive variational quantum algorithms
arxiv(2024)
摘要
Adaptive protocols enable the construction of more efficient state preparation circuits in variational quantum algorithms (VQAs) by utilizing data obtained from the quantum processor during the execution of the algorithm. This idea originated with ADAPT-VQE, an algorithm that iteratively grows the state preparation circuit operator by operator, with each new operator accompanied by a new variational parameter, and where all parameters acquired thus far are optimized in each iteration. In ADAPT-VQE and other adaptive VQAs that followed it, it has been shown that initializing parameters to their optimal values from the previous iteration speeds up convergence and avoids shallow local traps in the parameter landscape. However, no other data from the optimization performed at one iteration is carried over to the next. In this work, we propose an improved quasi-Newton optimization protocol specifically tailored to adaptive VQAs. The distinctive feature in our proposal is that approximate second derivatives of the cost function are recycled across iterations in addition to parameter values. We implement a quasi-Newton optimizer where an approximation to the inverse Hessian matrix is continuously built and grown across the iterations of an adaptive VQA. The resulting algorithm has the flavor of a continuous optimization where the dimension of the search space is augmented when the gradient norm falls below a given threshold. We show that this inter-optimization exchange of second-order information leads the Hessian in the state of the optimizer to better approximate the exact Hessian. As a result, our method achieves a superlinear convergence rate even in situations where the typical quasi-Newton optimizer converges only linearly. Our protocol decreases the measurement costs in implementing adaptive VQAs on quantum hardware as well as the runtime of their classical simulation.
更多查看译文
AI 理解论文
溯源树
样例
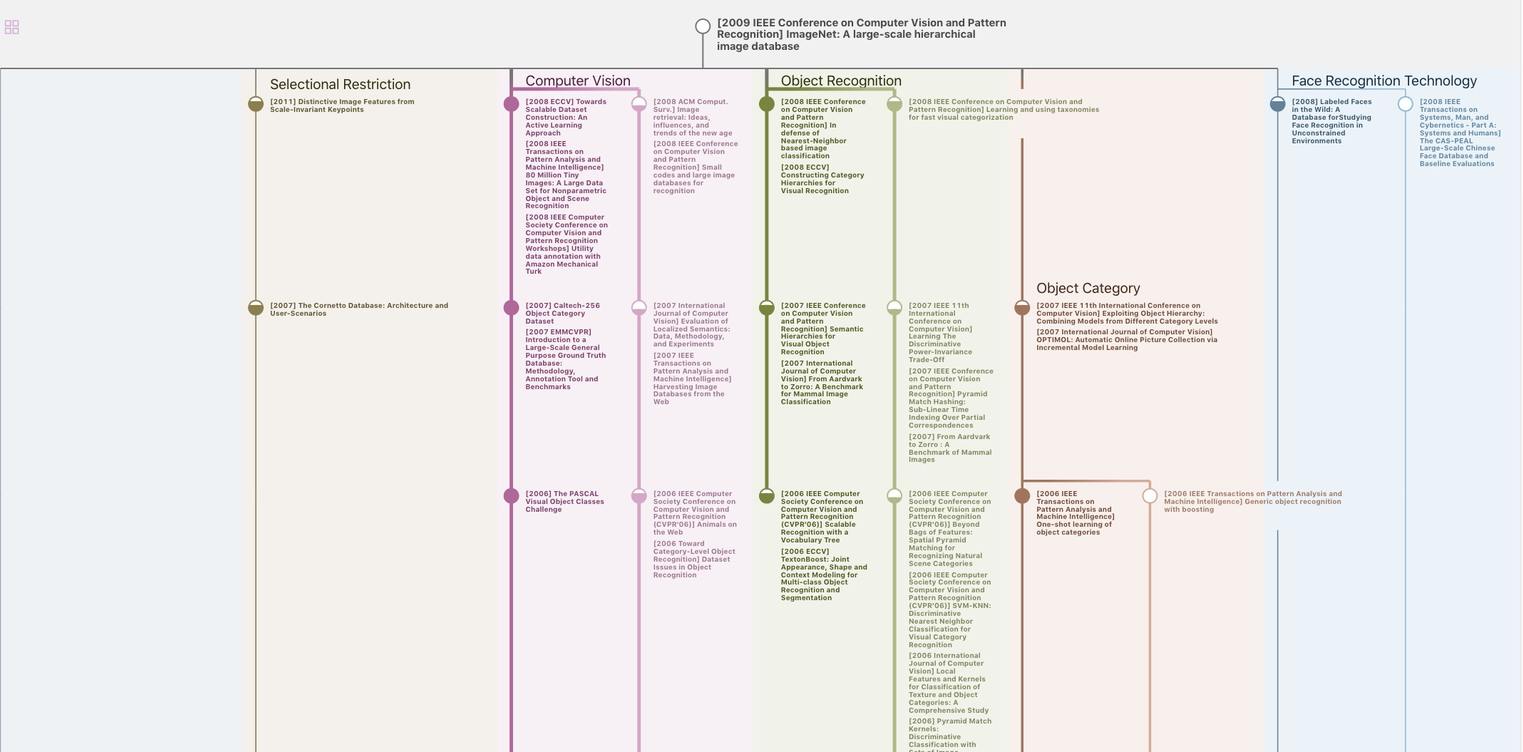
生成溯源树,研究论文发展脉络
Chat Paper
正在生成论文摘要