A Coherence-Guided InSAR Phase Unwrapping Method With Cycle-Consistent Adversarial Networks
IEEE JOURNAL OF SELECTED TOPICS IN APPLIED EARTH OBSERVATIONS AND REMOTE SENSING(2024)
摘要
Phase unwrapping (PU) is a critical processing step for obtaining information on land surface deformation from interferometric synthetic aperture radar (InSAR) images. Traditional PU methods take the phase continuity assumption as the precondition. However, in actual applications, there are many factors that can cause phase discontinuities, resulting in large errors in the PU results. With the fast development of deep learning, the data-driven deep learning methods for PU may help address this issue. Therefore, this study proposes a coherence-guided InSAR PU method with cycle-consistent adversarial networks. In this method, PU was considered a pixel-level regression problem from the interferogram to the unwrapped phase; cycle-consistent adversarial networks as one-step PU model were imported with the interferogram as the input and the unwrapped phase as the output. Except for adversarial loss and cycle consistency loss, based on the PU theory and the coherence of SAR images, the L-1 loss with the weight of the coherence coefficient was added to guide the training process of networks. In addition to the traditional mean square error index, the root mean square value of the phase loop closure was introduced to evaluate the quality of the generated unwrapped phase. The results show that the proposed method can not only achieve the accuracy of the traditional PU method in the region with good coherence but also obtain better unwrapping results in the region with poor coherence, where the traditional PU method is restricted. It is also superior to the existing PU method with a conditional adversarial network.
更多查看译文
关键词
Coherence,cycle consistency,generative adversarial networks,interferometric synthetic aperture radar (InSAR),phase unwrapping (PU)
AI 理解论文
溯源树
样例
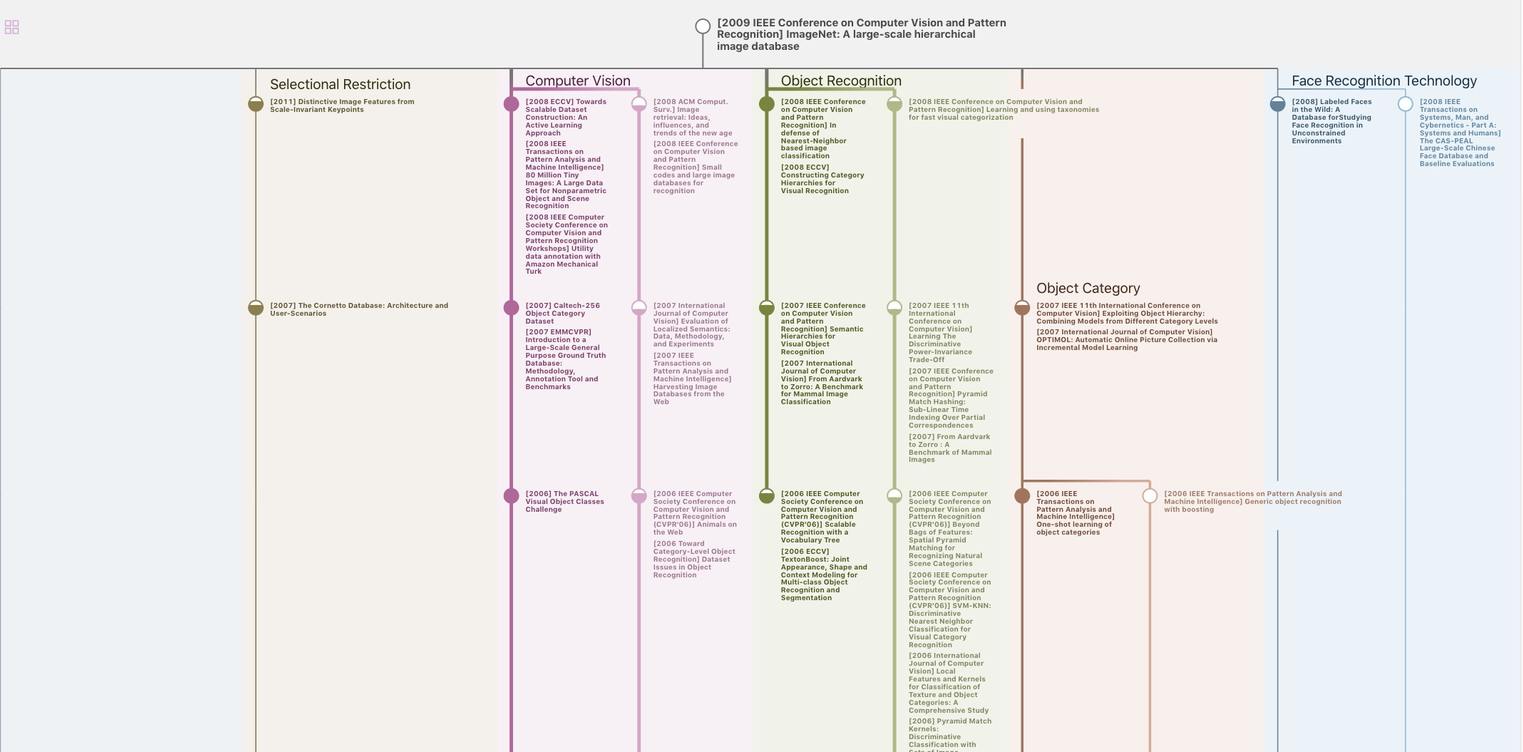
生成溯源树,研究论文发展脉络
Chat Paper
正在生成论文摘要