Demand forecasting of shared bicycles based on combined deep learning models
PHYSICA A-STATISTICAL MECHANICS AND ITS APPLICATIONS(2024)
摘要
The combined deep learning model for bicycle sharing demand prediction is designed to solve the "last 1 km" problem. At present, there are many companies providing bicycle sharing services at home and abroad, and how to dispatch shared bicycles more efficiently has become an important issue in traffic information research. Sometimes it is difficult to find shared bikes at the exit of some subway stations, along commercial streets, or under some office buildings, while sometimes there are mountains of shared bikes. Therefore, performing demand prediction of shared bikes can efficiently increase the scheduling efficiency of shared bikes, optimize the distribution of shared bikes, and provide more convenient travel services for users. Based on traffic flow prediction theory, this paper studies the spatial and temporal features of shared bicycles. The results show that factors such as time of day, season, weather, and temperature have an effect on the demand for bicycles. Based on the above-mentioned characteristic influencing factors, a CNNLSTM-Attention algorithm is proposed to forecast the demand for shared bicycles in this paper. Firstly, a CNN-LSTM-Attention model is constructed to predict the demand for bicycle sharing based on the open-source data provided by Capital Bicycle Company. Secondly, it is proved that CNN-LSTM-Attention model is better than 1DCNN-LSTM-Attention, CNN-LSTM, LSTM, SVRbased model and BP neural network model in the precision prediction of shared bicycles, in which the prediction accuracy reaches 97.50%, which confirms the practicality and effectiveness of the model.
更多查看译文
关键词
Urban transportation,Bicycle sharing,Data analysis,CNN-LSTM-attention,Demand forecasting
AI 理解论文
溯源树
样例
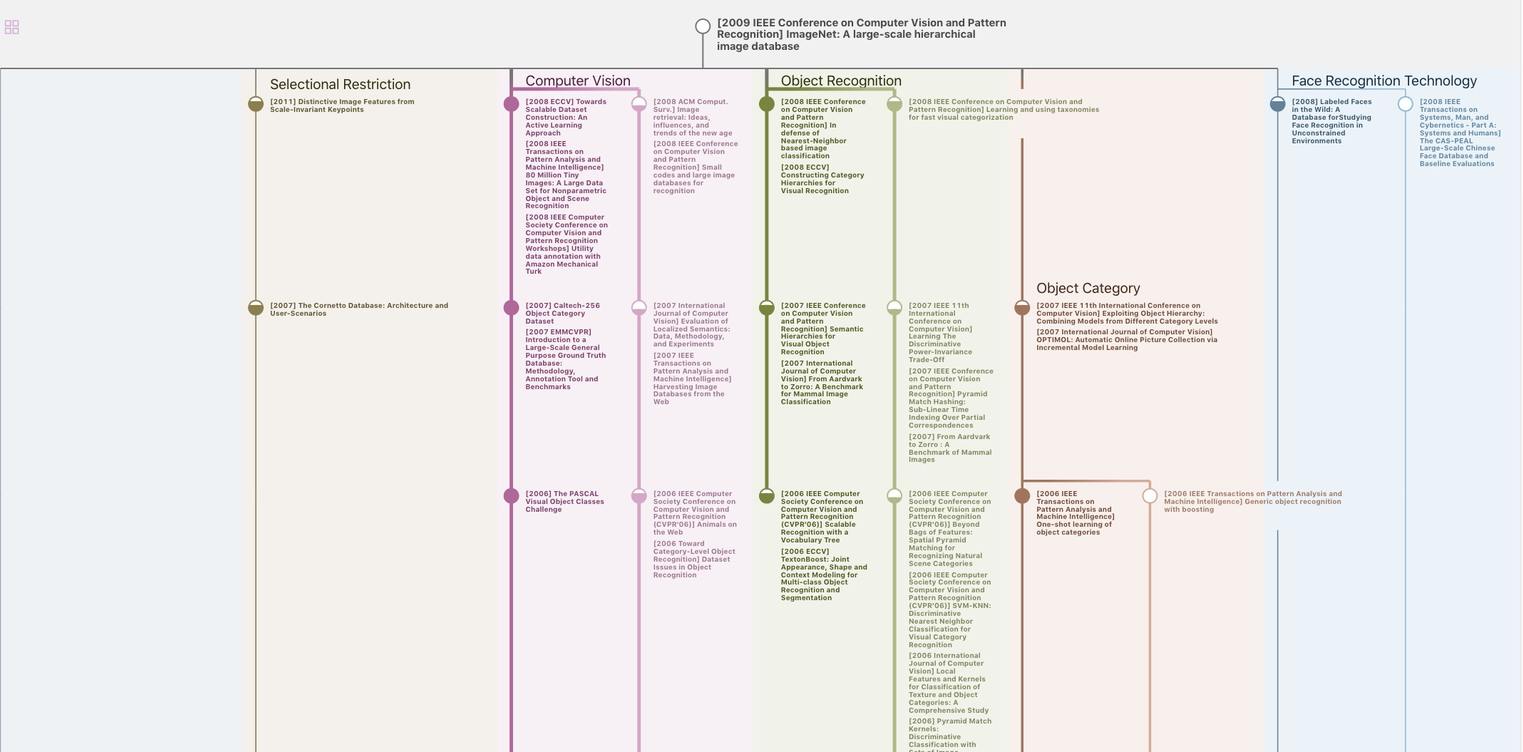
生成溯源树,研究论文发展脉络
Chat Paper
正在生成论文摘要