Leveraging Deep Neural Networks for Estimating Vickers Hardness from Nanoindentation Hardness
MATERIALS(2024)
摘要
This research presents a comprehensive analysis of deep neural network models (DNNs) for the precise prediction of Vickers hardness (HV) in nitrided and carburized M50NiL steel samples, with hardness values spanning from 400 to 1000 HV. By conducting rigorous experimentation and obtaining corresponding nanoindentation data, we evaluated the performance of four distinct neural network architectures: Multilayer Perceptron (MLP), Convolutional Neural Network (CNN), Long Short-Term Memory network (LSTM), and Transformer. Our findings reveal that MLP and LSTM models excel in predictive accuracy and efficiency, with MLP showing exceptional iteration efficiency and predictive precision. The study validates models for broad application in various steel types and confirms nanoindentation as an effective direct measure for HV hardness in thin films and gradient-variable regions. This work contributes a validated and versatile approach to the hardness assessment of thin-film materials and those with intricate microstructures, enhancing material characterization and potential application in advanced material engineering.
更多查看译文
关键词
deep neural network,hardness,nanoindentation,nitriding
AI 理解论文
溯源树
样例
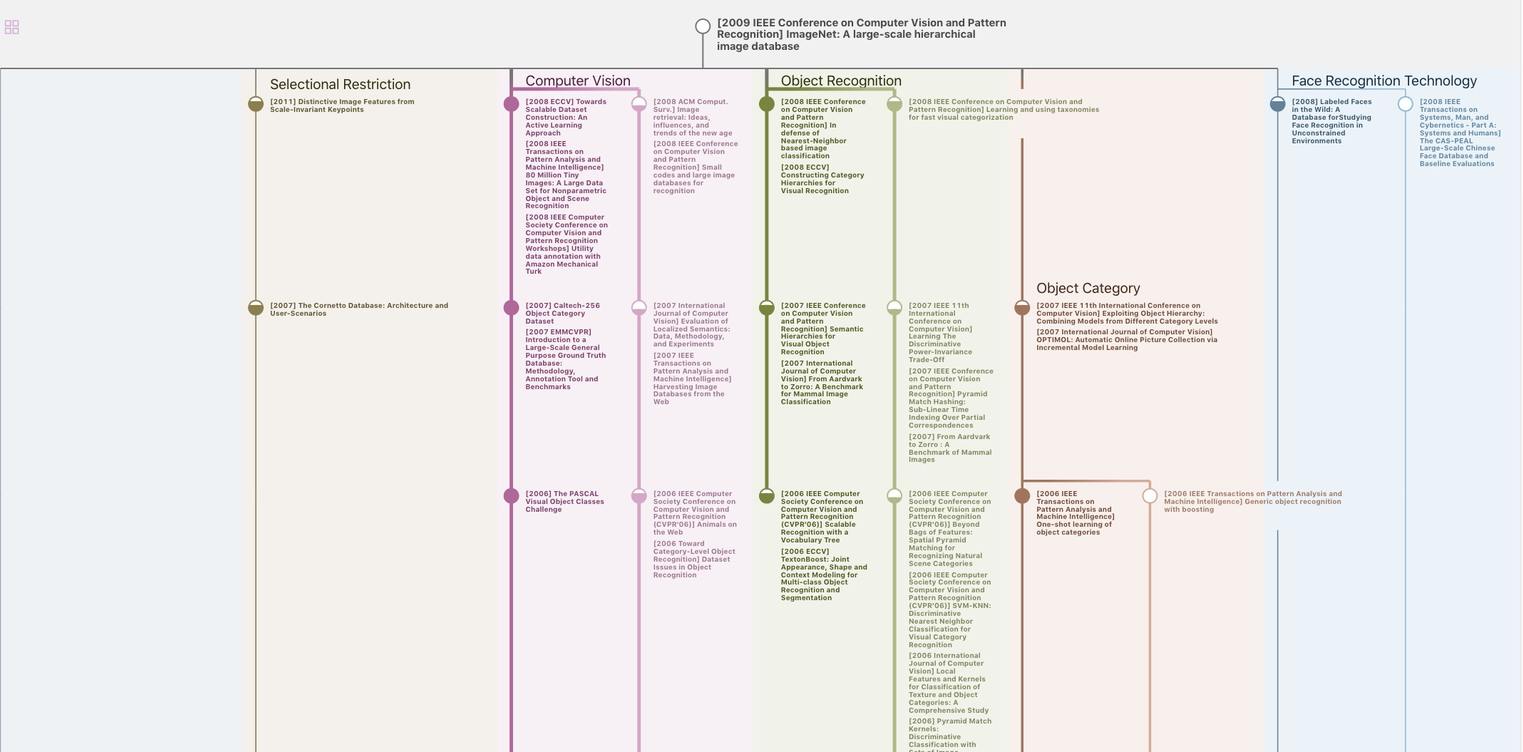
生成溯源树,研究论文发展脉络
Chat Paper
正在生成论文摘要