Safe-Learning-Based Location-Privacy-Preserved Task Offloading in Mobile Edge Computing
ELECTRONICS(2024)
摘要
Mobile edge computing (MEC) integration with 5G/6G technologies is an essential direction in mobile communications and computing. However, it is crucial to be aware of the potential privacy implications of task offloading in MEC scenarios, specifically the leakage of user location information. To address this issue, this paper proposes a location-privacy-preserved task offloading (LPTO) scheme based on safe reinforcement learning to balance computational cost and privacy protection. This scheme uses the differential privacy technique to perturb the user's actual location to achieve location privacy protection. We model the privacy-preserving location perturbation problem as a Markov decision process (MDP), and we develop a safe deep Q-network (DQN)-based LPTO (SDLPTO) scheme to select the offloading policy and location perturbation policy dynamically. This approach effectively mitigates the selection of high-risk state-action pairs by conducting a risk assessment for each state-action pair. Simulation results show that the proposed SDLPTO scheme has a lower computational cost and location privacy leakage than the benchmarks. These results highlight the significance of our approach in protecting user location privacy while achieving improved performance in MEC environments.
更多查看译文
关键词
mobile edge computing,location privacy,differential privacy,location perturbation,safe reinforcement learning
AI 理解论文
溯源树
样例
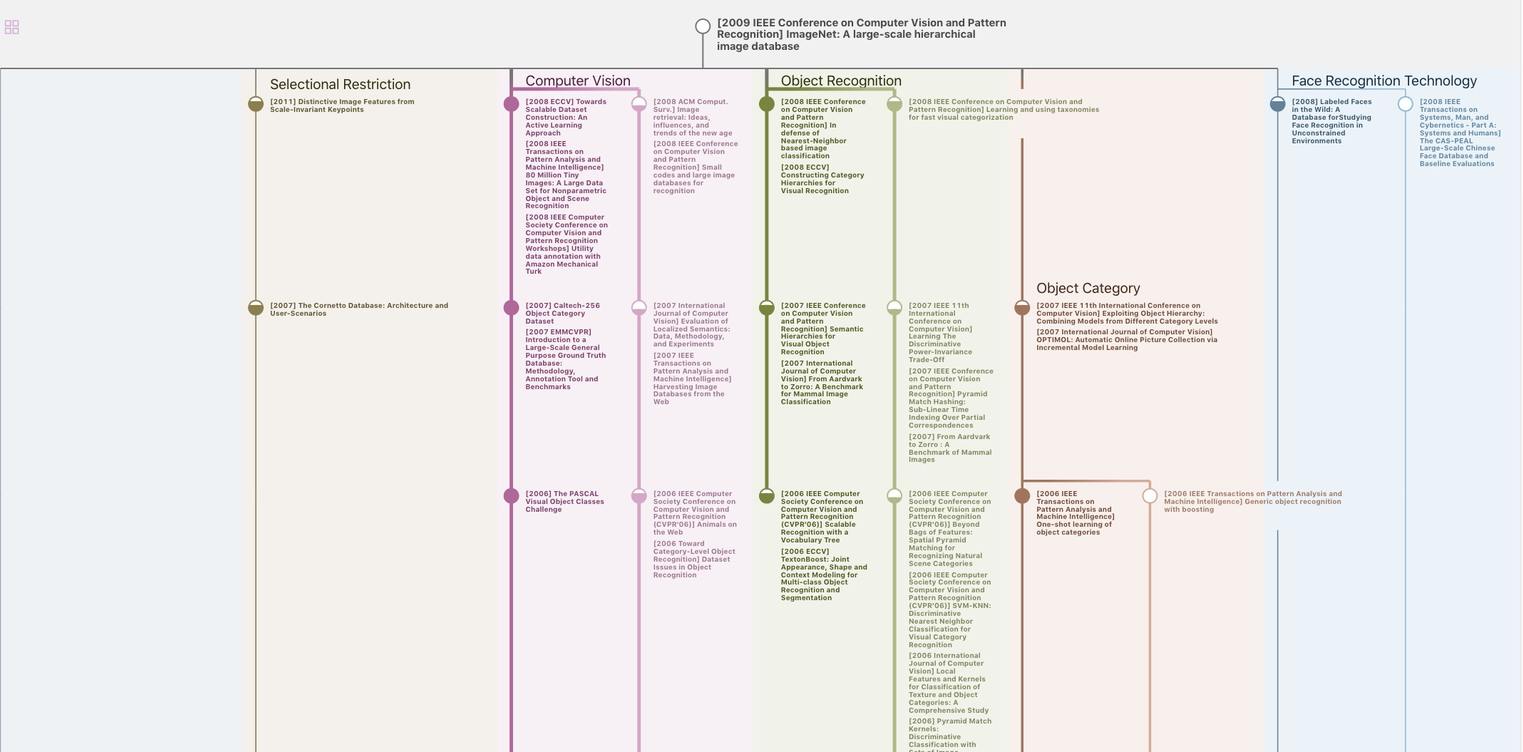
生成溯源树,研究论文发展脉络
Chat Paper
正在生成论文摘要