TTDAT: Two-Step Training Dual Attention Transformer for Malware Classification Based on API Call Sequences
APPLIED SCIENCES-BASEL(2024)
摘要
The surge in malware threats propelled by the rapid evolution of the internet and smart device technology necessitates effective automatic malware classification for robust system security. While existing research has primarily relied on some feature extraction techniques, issues such as information loss and computational overhead persist, especially in instruction-level tracking. To address these issues, this paper focuses on the nuanced analysis of API (Application Programming Interface) call sequences between the malware and system and introduces TTDAT (Two-step Training Dual Attention Transformer) for malware classification. TTDAT utilizes Transformer architecture with original multi-head attention and an integrated local attention module, streamlining the encoding of API sequences and extracting both global and local patterns. To expedite detection, we introduce a two-step training strategy: ensemble Transformer models to generate class representation vectors, thereby bolstering efficiency and adaptability. Our extensive experiments demonstrate TTDAT's effectiveness, showcasing state-of-the-art results with an average F1 score of 0.90 and an accuracy of 0.96.
更多查看译文
关键词
two-step training,dual attention,Transformer,malware classification,API call sequences
AI 理解论文
溯源树
样例
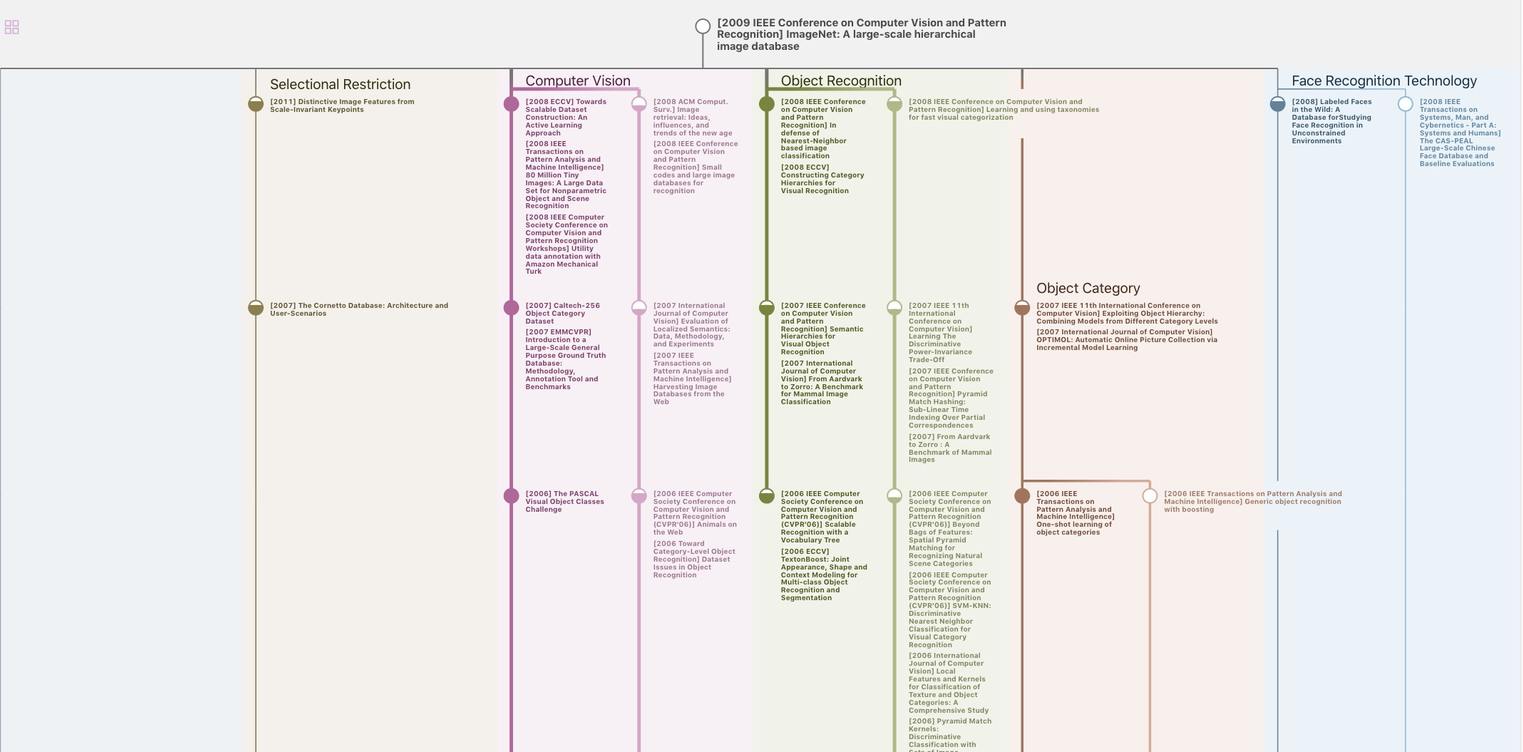
生成溯源树,研究论文发展脉络
Chat Paper
正在生成论文摘要