Supervised Contrastive Learning for Fault Diagnosis Based on Phase-Resolved Partial Discharge in Gas-Insulated Switchgear
ENERGIES(2024)
摘要
Supervised contrastive learning (SCL) has recently emerged as an alternative to conventional machine learning and deep neural networks. In this study, we propose an SCL model with data augmentation techniques using phase-resolved partial discharge (PRPD) in gas-insulated switchgear (GIS). To increase the fault data for training, we employ Gaussian noise adding, Gaussian noise scaling, random cropping, and phase shifting for supervised contrastive loss. The performance of the proposed SCL was verified by four types of faults in the GIS and on-site noise using an on-line ultra-high-frequency (UHF) partial discharge (PD) monitoring system. The experimental results show that the proposed SCL achieves a classification accuracy of 97.28% and outperforms the other algorithms, including support vector machines (SVM), multilayer perceptron (MLP), and convolution neural networks (CNNs) in terms of classification accuracy, by 6.8%, 4.28%, 2.04%, respectively.
更多查看译文
关键词
data augmentation,fault diagnosis,phase-resolved partial discharge (PRPD),supervised contrastive learning (SCL)
AI 理解论文
溯源树
样例
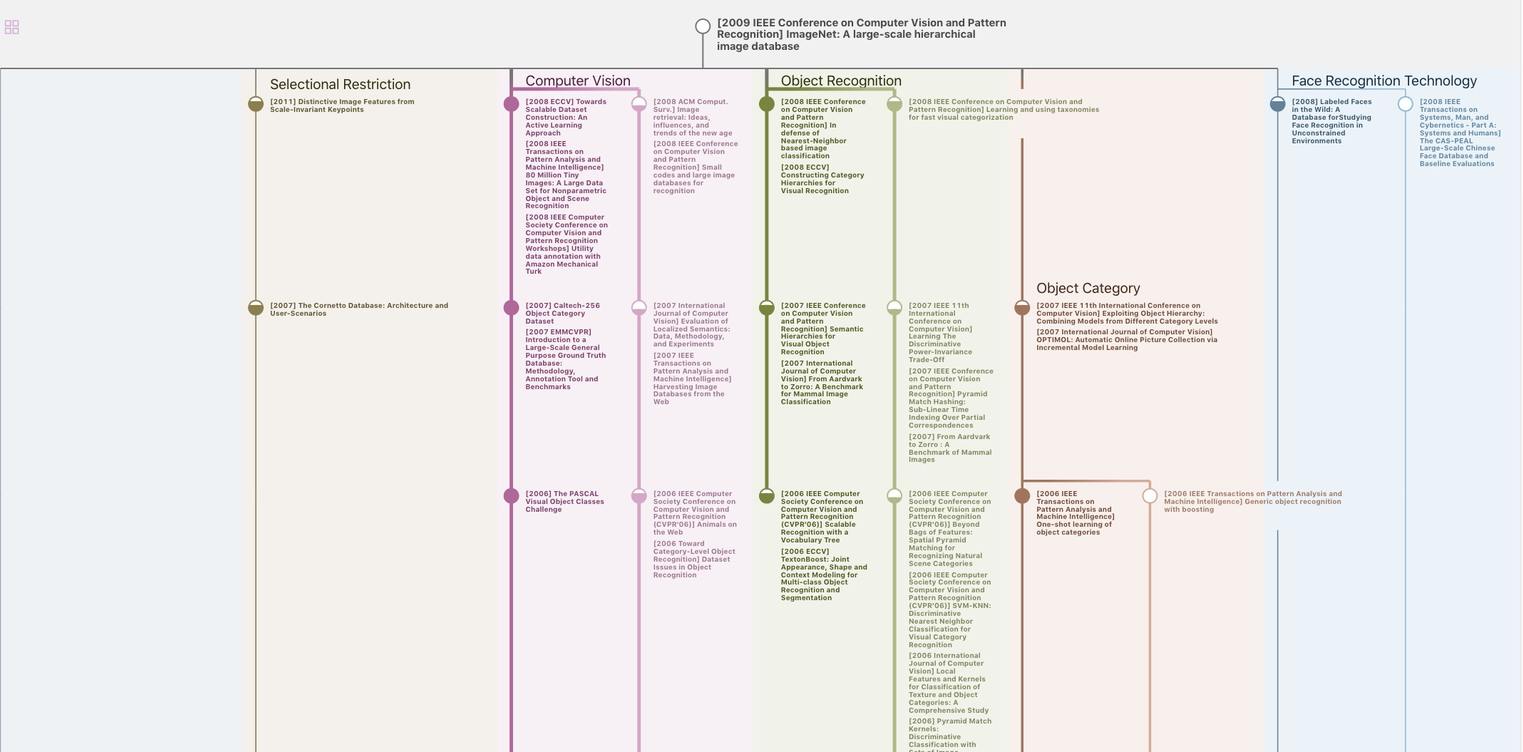
生成溯源树,研究论文发展脉络
Chat Paper
正在生成论文摘要