PrOuD: Probabilistic Outlier Detection Solution for Time-Series Analysis of Real-World Photovoltaic Inverters
ENERGIES(2024)
摘要
Anomaly detection methods applied to time series are mostly viewed as black boxes that solely provide a deterministic answer for the detected target. Without a convincing explanation, domain experts can hardly trust the detection results and must conduct further time-series diagnoses in real-world applications. To overcome this challenge, we mathematically analyzed the sources of anomalies and novelties in multivariate time series as well as their relationships from the perspective of Gaussian-distributed non-stationary noise. Furthermore, we proposed mathematical methods to generate artificial time series and synthetic anomalies, with the goal of solving the problem of it being difficult to train and evaluate models for real-world applications due to the lack of sufficient data. In addition, we designed Probabilistic Outlier Detection (PrOuD), which is a general solution to provide interpretable detection results to assist domain experts with time-series analysis. PrOuD can convert the predictive uncertainty of a time-series value from a trained model into the estimated uncertainty of the detected outlier through Monte Carlo estimation. The experimental results obtained on both artificial time series and real-world photovoltaic inverter data demonstrated that the proposed solution can detect emerging anomalies accurately and quickly. The implemented PrOuD demo case shows its potential to make the detection results of existing detection methods more convincing so that domain experts can more efficiently complete their tasks, such as time-series diagnosis and anomalous pattern clustering.
更多查看译文
关键词
anomaly,novelty,outlier detection,photovoltaic,probabilistic forecasting,explainability,time series
AI 理解论文
溯源树
样例
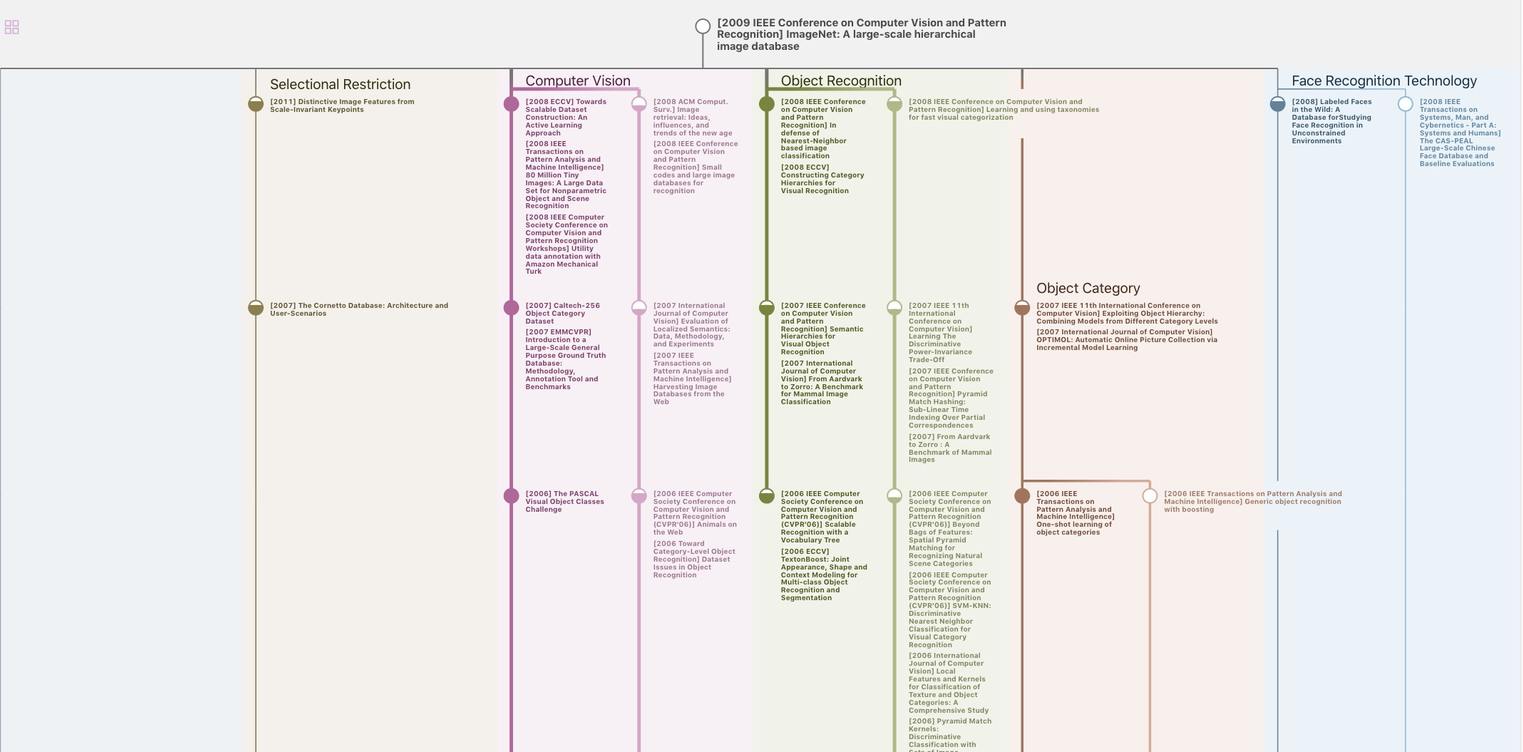
生成溯源树,研究论文发展脉络
Chat Paper
正在生成论文摘要