DMCH: A Deep Metric and Category-Level Semantic Hashing Network for Retrieval in Remote Sensing
REMOTE SENSING(2024)
摘要
The effectiveness of hashing methods in big data retrieval has been proved due to their merit in computational and storage efficiency. Recently, encouraged by the strong discriminant capability of deep learning in image representation, various deep hashing methodologies have emerged to enhance retrieval performance. However, maintaining the semantic richness inherent in remote sensing images (RSIs), characterized by their scene intricacy and category diversity, remains a significant challenge. In response to this challenge, we propose a novel two-stage deep metric and category-level semantic hashing network termed DMCH. First, it introduces a novel triple-selection strategy during the semantic metric learning process to optimize the utilization of triple-label information. Moreover, it inserts a hidden layer to enhance the latent correlation between similar hash codes via a designed category-level classification loss. In addition, it employs additional constraints to keep bit-uncorrelation and bit-balance of generated hash codes. Furthermore, a progressive coarse-to-fine hash code sorting scheme is used for superior fine-grained retrieval and more effective hash function learning. Experiment results on three datasets illustrate the effectiveness and superiority of the proposed method.
更多查看译文
关键词
deep hash learning,category-level semantics,remote sensing image retrieval
AI 理解论文
溯源树
样例
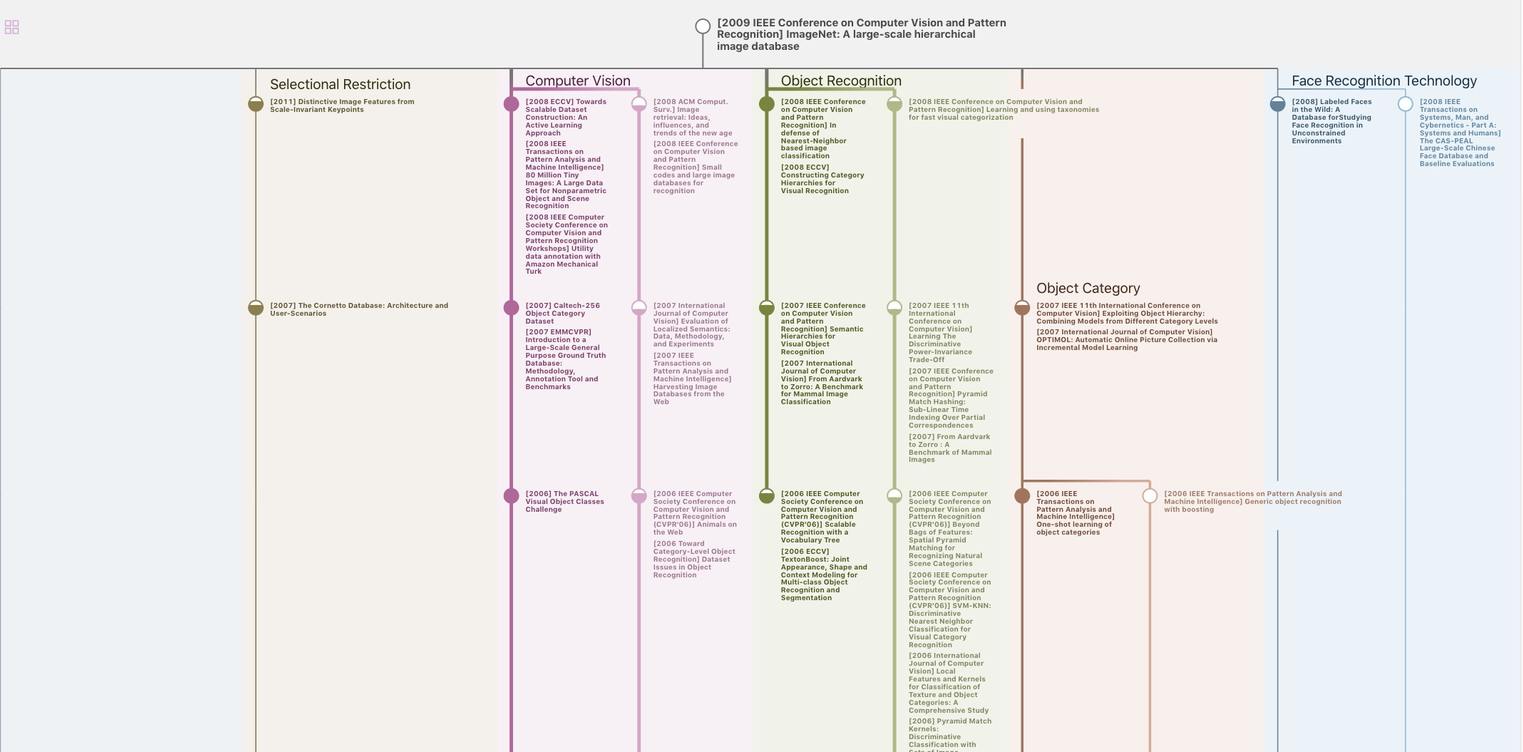
生成溯源树,研究论文发展脉络
Chat Paper
正在生成论文摘要