Non-Intrusive Reduced-Order Modeling Based on Parametrized Proper Orthogonal Decomposition
ENERGIES(2024)
摘要
A new non-intrusive reduced-order modeling method based on space-time parameter decoupling for parametrized time-dependent problems is proposed. This method requires the preparation of a database comprising high-fidelity solutions. The spatial bases are extracted from the database through first-level proper orthogonal decomposition (POD). The algebraic relationship between the time trajectory/parameter positions and the projection coefficient is described by the linear superposition of the second-level POD bases (temporal bases) and the second-level projection coefficients (parameter-dependent coefficients). This decomposition strategy decouples the space-time parameter effects, providing a stable foundation for fast predictions of parametrized time-dependent problems. The mappings between the parameter locations and the parameter-dependent coefficients are approximated as Gaussian process regression (GPR) models. The accuracy and efficiency of the PPOD-ROM are demonstrated through two numerical examples: flows past a cylinder and turbine flows with a clocking effect.
更多查看译文
关键词
non-intrusive reduced-order modeling,parametrized time-dependent problems,proper orthogonal decomposition,Gaussian process regression
AI 理解论文
溯源树
样例
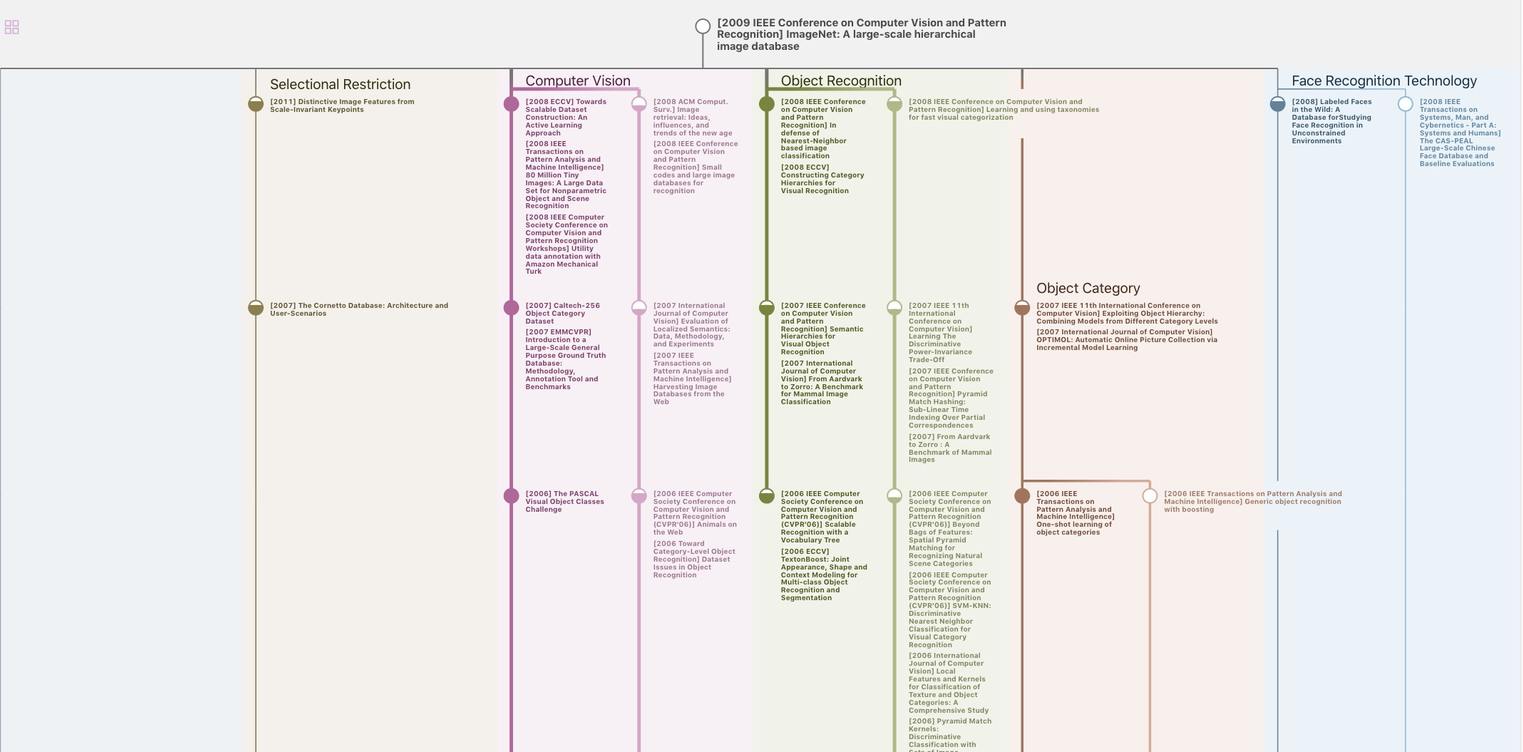
生成溯源树,研究论文发展脉络
Chat Paper
正在生成论文摘要