OEGR-DETR: A Novel Detection Transformer Based on Orientation Enhancement and Group Relations for SAR Object Detection
REMOTE SENSING(2024)
摘要
Object detection in SAR images has always been a topic of great interest in the field of deep learning. Early works commonly focus on improving performance on convolutional neural network frameworks. More recent works continue this path and introduce the attention mechanisms of Transformers for better semantic interpretation. However, these methods fail to treat the Transformer itself as a detection framework and, therefore, lack the development of various details that contribute to the state-of-the-art performance of Transformers. In this work, we first base our work on a fully multi-scale Transformer-based detection framework, DETR (DEtection TRansformer) to utilize its superior detection performance. Secondly, to acquire rotation-related attributes for better representation of SAR objects, an Orientation Enhancement Module (OEM) is proposed to facilitate the enhancement of rotation characteristics. Then, to enable learning of more effective and discriminative representations of foreground objects and background noises, a contrastive-loss-based GRC Loss is proposed to preserve patterns of both categories. Moreover, to not restrict comparisons exclusively to maritime objects, we have also developed an open-source labeled vehicle dataset. Finally, we evaluate both detection performance and generalization ability on two well-known ship datasets and our vehicle dataset. We demonstrated our method's superior performance and generalization ability on both datasets.
更多查看译文
关键词
Transformer framework,feature enhancement,contrastive loss,deep learning,object detection,SAR images
AI 理解论文
溯源树
样例
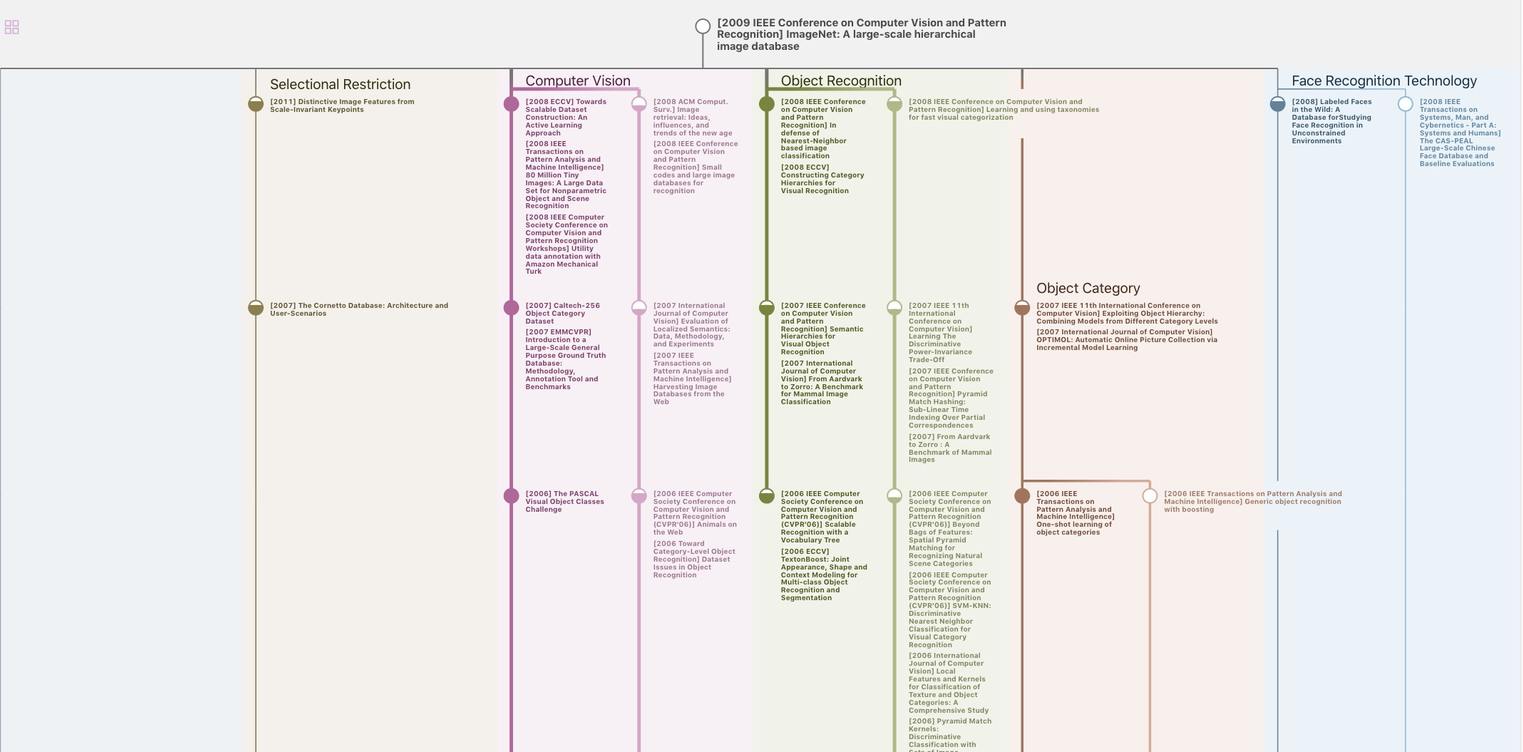
生成溯源树,研究论文发展脉络
Chat Paper
正在生成论文摘要