SAKMR: Industrial control anomaly detection based on semi-supervised hybrid deep learning
Peer-to-Peer Networking and Applications(2024)
摘要
With the advent of Industry 4.0, industrial control systems (ICS) are more and more closely connected with the Internet, leading to a rapid increase in the types and quantities of security threats that arise from ICS. Anomaly detection is an effective defense measure against attacks. At present, it is the main trend to use hybrid deep learning methods to realize ICS anomaly detection. However, we found that many ICS anomaly detection methods based on hybrid deep learning adopt phased learning, in which each phase is optimized separately with optimization goals deviating from the overall goal. In view of this issue, we propose an end-to-end anomaly detection method SAKMR based on hybrid deep learning. Our method uses radial basis function network (RBFN) to realize K-means clustering, and combines it with stacked auto-encoder (SAE), which is conducive to defining reconstruction error and clustering error into an objective function to ensure joint optimization of feature extraction and classification. Experiments were conducted on the commonly used KDDCUP99 and SWAT datasets. The results show that SAKMR is effective in detecting abnormal industrial control data and outperforms the baseline methods on multiple performance indicators such as F1-Measure.
更多查看译文
关键词
Hybrid deep learning,Industrial control system,Semi-supervision,Anomaly detection
AI 理解论文
溯源树
样例
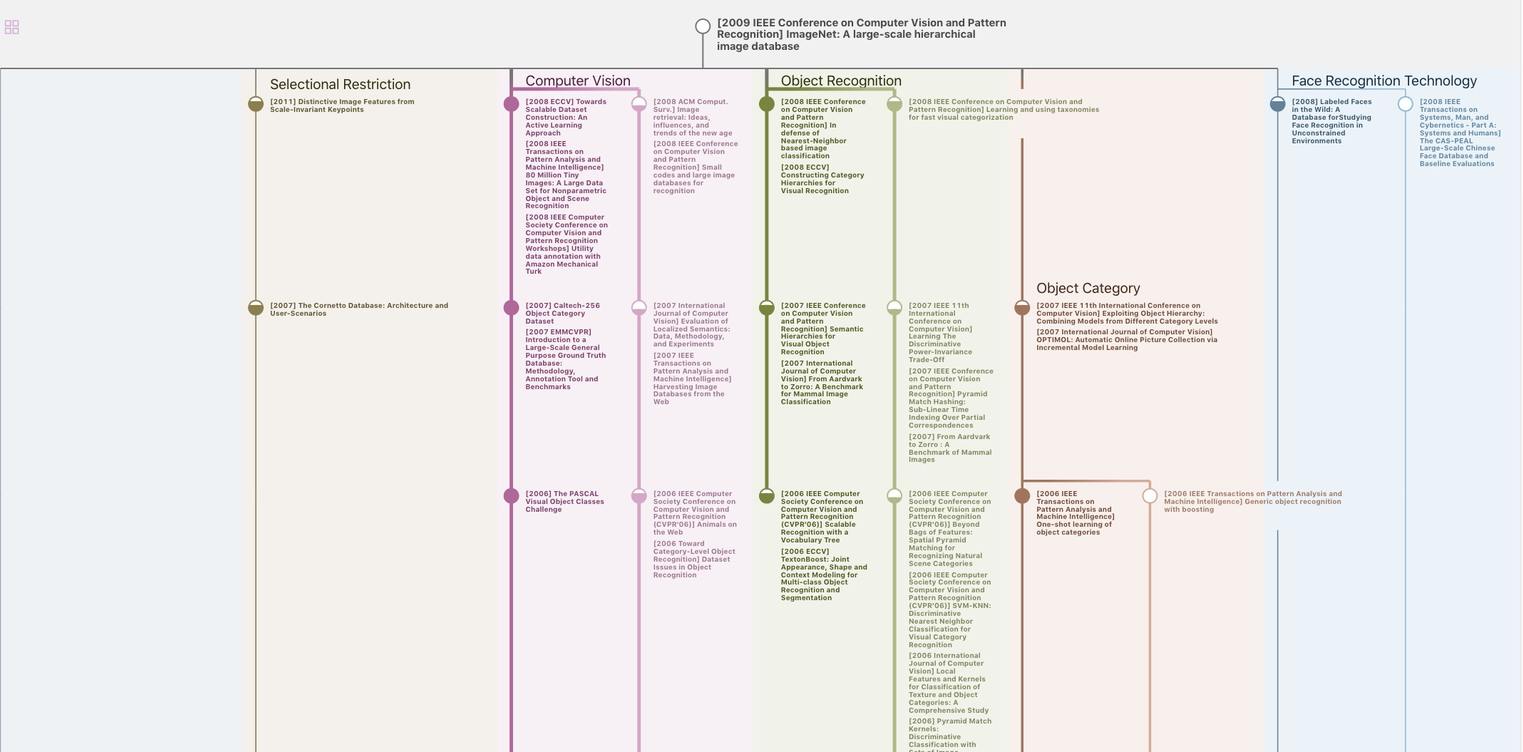
生成溯源树,研究论文发展脉络
Chat Paper
正在生成论文摘要