A modified deep semantic binarization network for degradation removal in palm leaf manuscripts
Multimedia Tools and Applications(2024)
摘要
Palm leaves are the earliest forms of documentation for literature, showcasing rich traditions, philosophical insights, and scientific traditions in areas such as mathematics, medicine, agriculture, and martial arts, among others. This paper presents a deep semantic binarization network for enhancing 700-year-old Malayalam palm leaf manuscripts by addressing challenges such as uneven illumination, ink bleeds, stain marks, and brittleness. The learning model is trained with the ground truth data created using self-collected Malayalam palm leaf manuscripts, the Shiju Alex, and AMADI LONTAR degraded palm leaf manuscripts. The learning models are created by employing hyperparameter specifications of a fixed batch size of 32 with a learning rate of 0.00001, with epochs ranging from 100 to 500. Each learning model is analyzed by evaluating its performance using the proposed model, basic U-Net, and Sauvola Net on the datasets of AMADI LONTAR, Shiju Alex, and self-collected Malayalam manuscripts. The quantitative evaluation results show that the proposed model outperforms U-Net and the Sauvola Net models by achieving 90.55%, 0.205, and 90.44 of Accuracy, RMSE, and F-Measure towards validation set of self-collected datasets with batch sizes of 32 and 500 epochs. The data growth study conducted with varying training sample sizes shows a consistent increase in performance by the proposed model by achieving an accuracy of 90.55%, 88.26% precision, 70% recall, and 79% F-score towards validation of three datasets, demonstrating the effectiveness of the proposed method.
更多查看译文
关键词
Palm leaf documents,Image binarization,Preprocessing,U-Net,Sauvola Net,Transfer learning
AI 理解论文
溯源树
样例
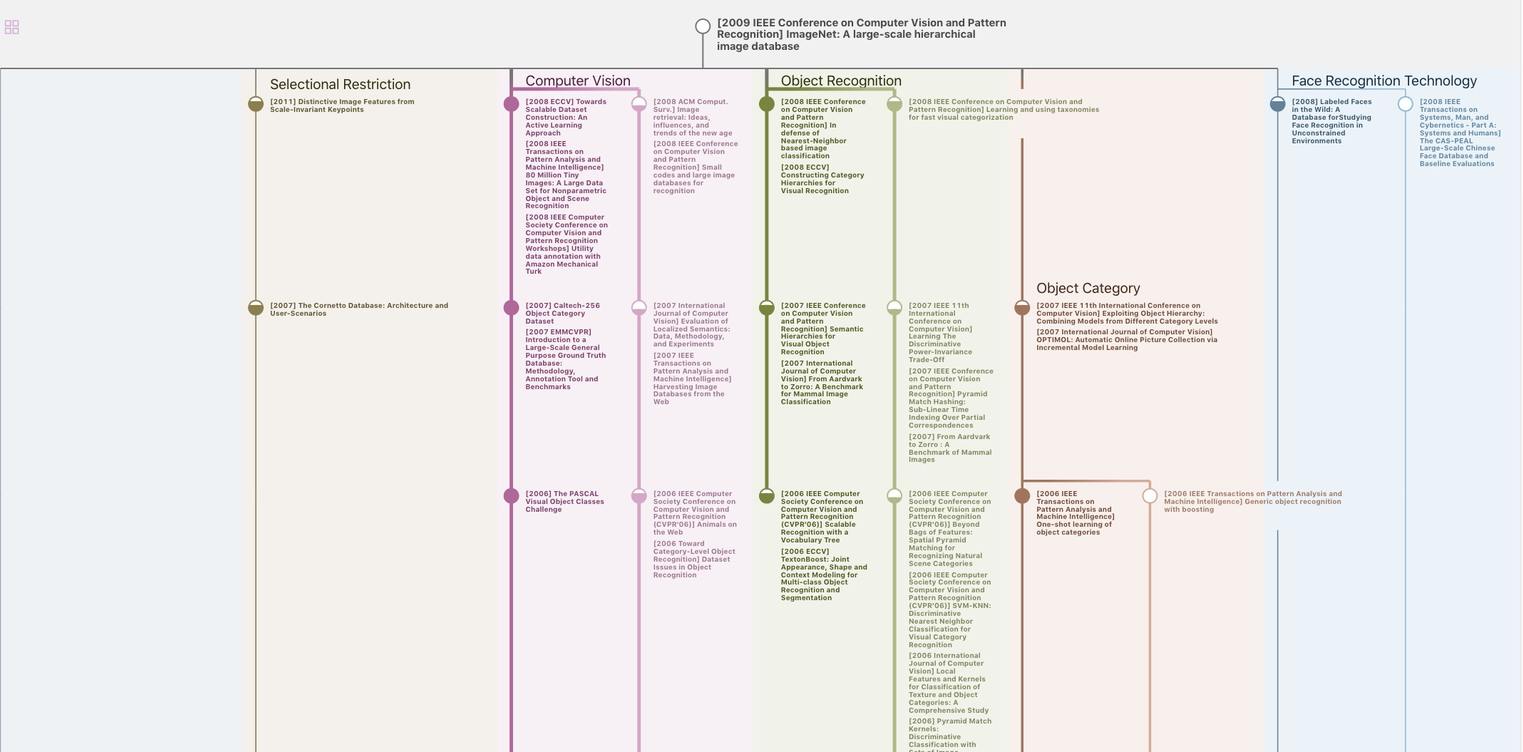
生成溯源树,研究论文发展脉络
Chat Paper
正在生成论文摘要