Nonnegative Matrix Functional Factorization for Hyperspectral Unmixing With Nonuniform Spectral Sampling
IEEE TRANSACTIONS ON GEOSCIENCE AND REMOTE SENSING(2024)
摘要
Unmixing is a crucial technique in analyzing hyperspectral imaging (HSI) data, which involves identifying the endmembers present in the data and estimating their abundance maps. Due to some practical constraints in the atmospheric environment, HSI data is usually nonuniformly distributed along the spectral domain, which brings incomplete spectral information in the hyperspectral unmixing. To overcome this issue, we propose, in this article, nonnegative matrix functional factorization (NMFF) which is an extension of classical nonnegative matrix factorization (NMF) for hyperspectral unmixing. In particular, we present a novel functional factorization model by incorporating the implicit neural representations (INRs) to learn about endmembers. Our method effectively characterizes endmembers by learning a continuous representation through INR with positional encoding, capturing the nonuniform distribution of spectral wavelengths. This distinct approach streamlines NMFF's iterative process for abundance extraction, bypassing the conventionally complex and cumbersome processing. When tested on various datasets, our hyperspectral unmixing approach consistently outperforms established techniques, showcasing the enhanced capabilities of our proposed model.
更多查看译文
关键词
Hyperspectral unmixing,implicit neural representation (INR),nonuniform sampling,nonnegative matrix factorization (NMF),positional encoding,spectral domain
AI 理解论文
溯源树
样例
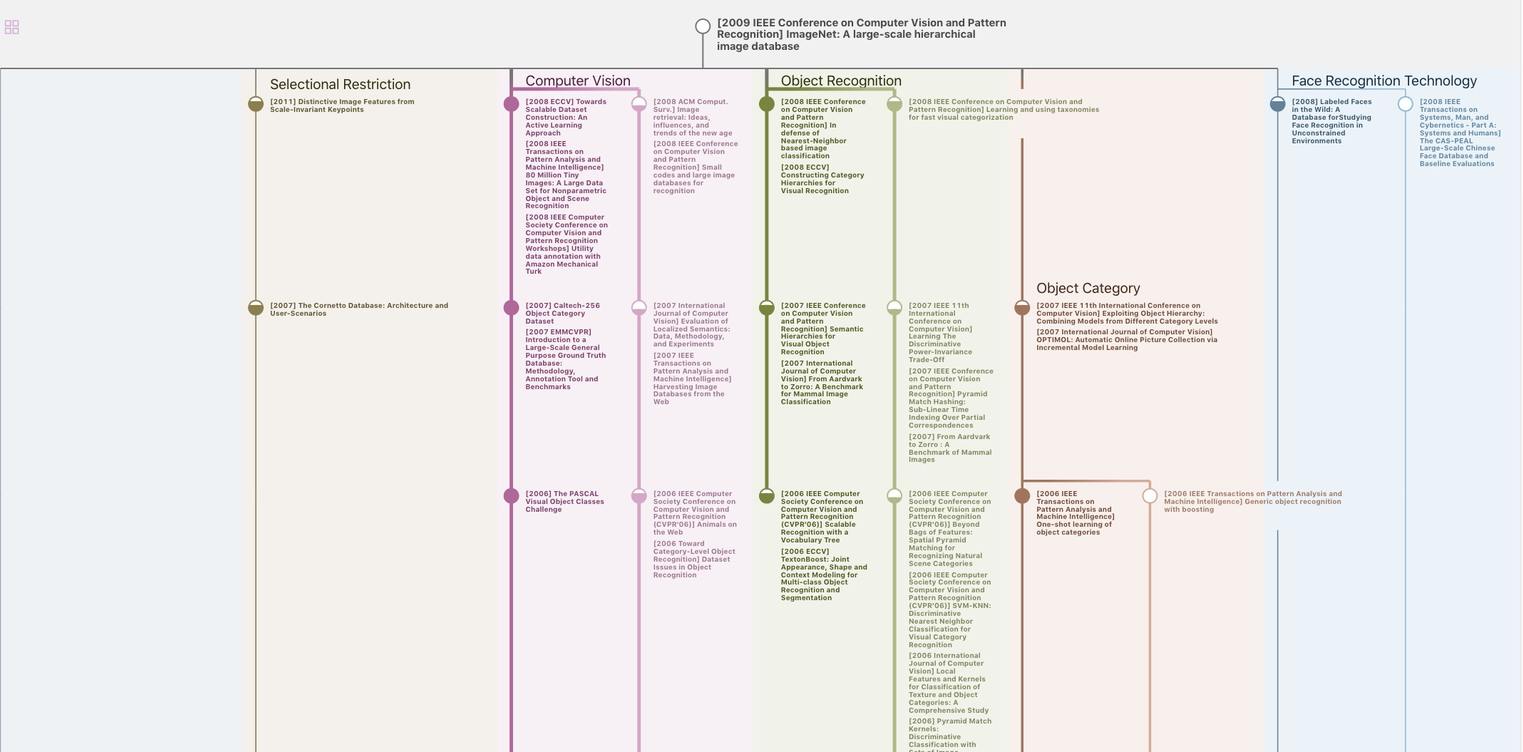
生成溯源树,研究论文发展脉络
Chat Paper
正在生成论文摘要