Shape-Sensitive Feature Extraction for Large-Aspect-Ratio Object Detection
IEEE GEOSCIENCE AND REMOTE SENSING LETTERS(2024)
摘要
The detection of objects with larger aspect ratios (OLARs) is a challenging problem in a special application scenario, such as remote-sensing object recognition and scene text detection. However, current object detectors perform poorly in OLAR feature extraction because they are incapable of adaptively responding to object shapes, which leads to severe misalignment between impure feature representations and region proposals. In this letter, we aim to solve this problem by proposing our shape-sensitive convolution network (SSC-Net). SSC-Net is carefully embedded with a feature enhancement module (SSC module) specifically suitable for OLAR. This module can use fewer sampling points to achieve more intelligent feature sampling area transformation, thus achieving the goal of enhancing OLAR feature representation. Extensive experiments on benchmark datasets that are rich in OLARs have proved the superiority of our method. Besides, we further verified the plug-and-play performance of the SSC module, and the experimental results show that it can significantly improve the detection performance of the detector for OLAR.
更多查看译文
关键词
Deep learning,feature extraction,large aspect ratios,object detection,remote sensing
AI 理解论文
溯源树
样例
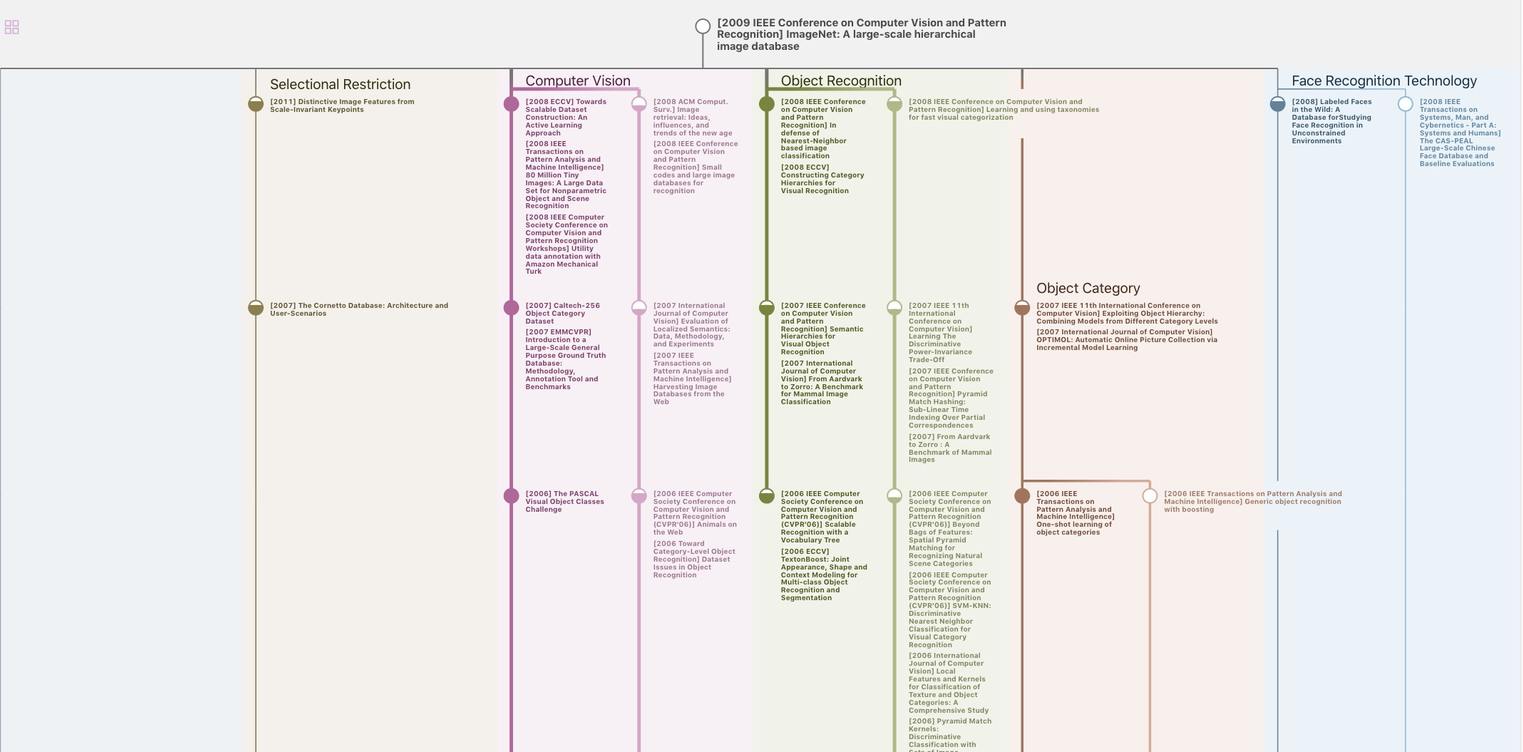
生成溯源树,研究论文发展脉络
Chat Paper
正在生成论文摘要