Enhanced lightweight deep network for efficient livestock detection in grazing areas
INTERNATIONAL JOURNAL OF ADVANCED ROBOTIC SYSTEMS(2024)
摘要
There are problems in the special pastoral environment, including large changes in target size and serious interference from light and environmental factors. To solve the above problems, an enhanced YOLOv4-tiny target detection network is proposed in this study. This network first solves the problem of livestock size fluctuation in pastoral areas, uses a pyramid network with multiscale feature fusion, and considers shallow local detail features and deep semantic information. Subsequently, a novel compound multichannel attention mechanism is proposed to increase the accuracy of the target detection network for the pastoral environment. The problem of poor accuracy of target detection network is solved. The algorithm is ported to Jetson AGX embedded platform for validation to examine the real-time performance of the algorithm. As revealed by the experimental results, enhanced YOLOv4-tiny achieves 89.77% detection accuracy and 30 frames/second detection speed, which increases the average detection accuracy by 11.67% compared with the conventional YOLOv4-tiny while maintaining almost the same detection rate.
更多查看译文
关键词
Object recognition and classification,deep learning,YOLOv4-tiny,attention mechanism,computer vision
AI 理解论文
溯源树
样例
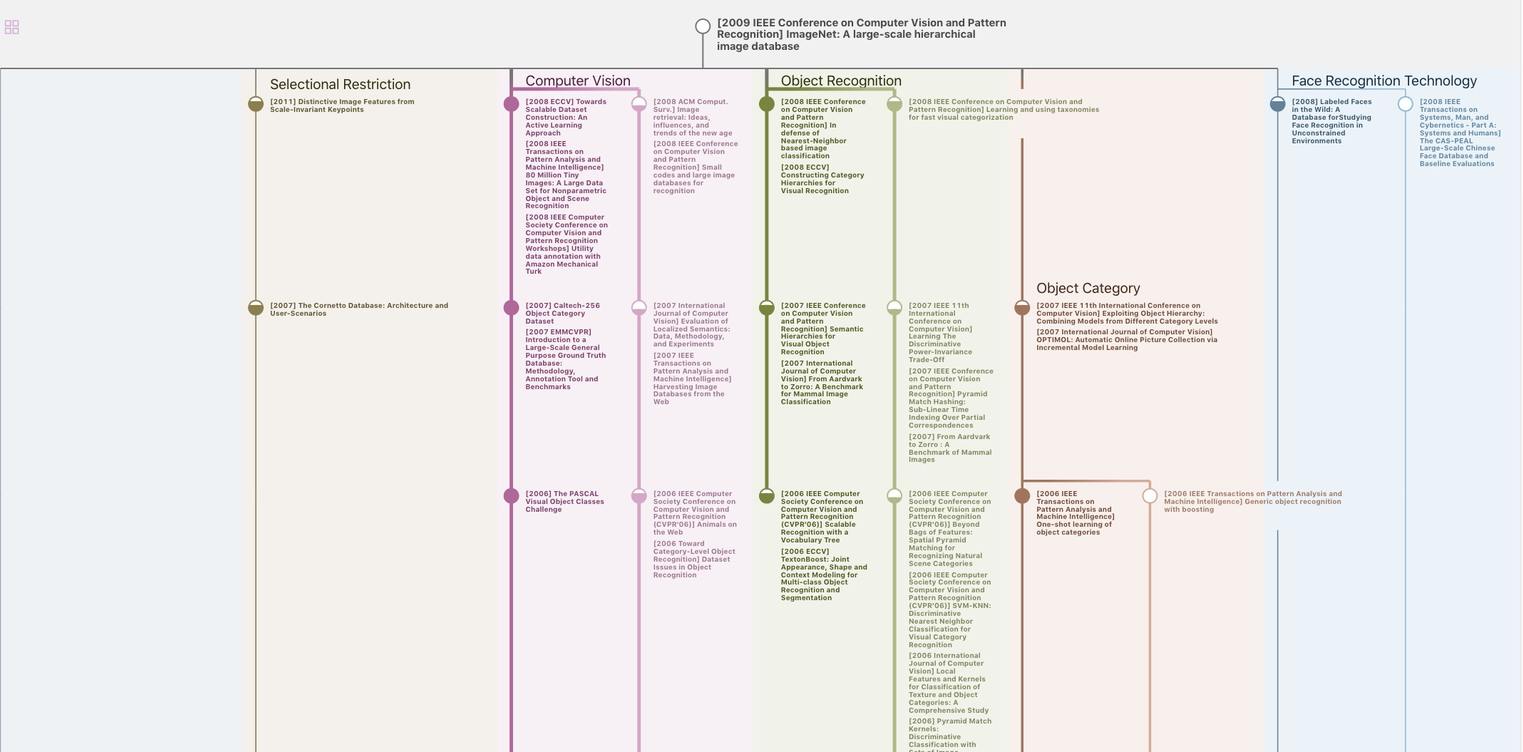
生成溯源树,研究论文发展脉络
Chat Paper
正在生成论文摘要