Class-Incremental Learning for Multi-Label Audio Classification
CoRR(2024)
摘要
In this paper, we propose a method for class-incremental learning of
potentially overlapping sounds for solving a sequence of multi-label audio
classification tasks. We design an incremental learner that learns new classes
independently of the old classes. To preserve knowledge about the old classes,
we propose a cosine similarity-based distillation loss that minimizes
discrepancy in the feature representations of subsequent learners, and use it
along with a Kullback-Leibler divergence-based distillation loss that minimizes
discrepancy in their respective outputs. Experiments are performed on a dataset
with 50 sound classes, with an initial classification task containing 30 base
classes and 4 incremental phases of 5 classes each. After each phase, the
system is tested for multi-label classification with the entire set of classes
learned so far. The proposed method obtains an average F1-score of 40.9
the five phases, ranging from 45.2
4 on 50 classes. Average performance degradation over incremental phases is
only 0.7 percentage points from the initial F1-score of 45.2
更多查看译文
关键词
Class-incremental learning,independent learning,knowledge transfer,multi-label audio classification
AI 理解论文
溯源树
样例
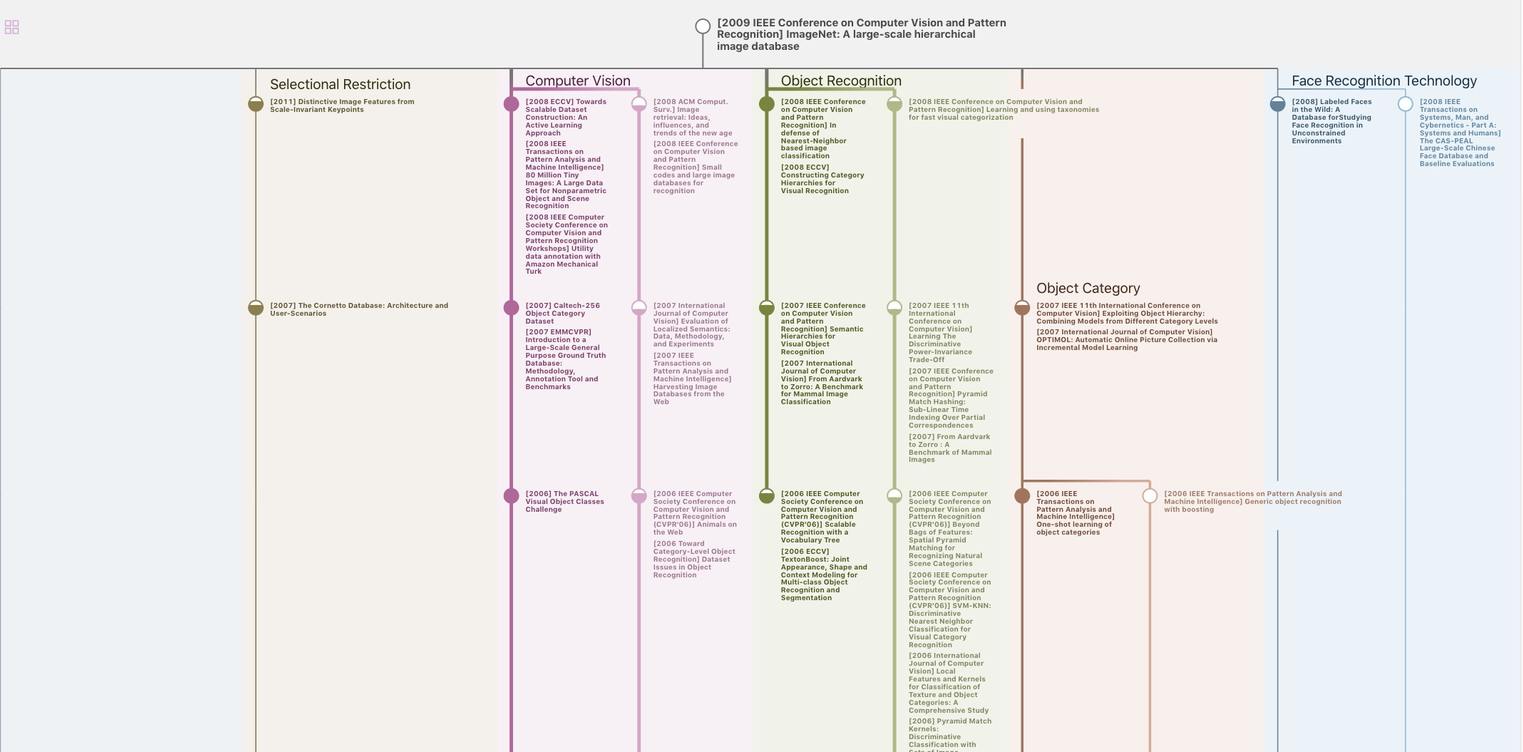
生成溯源树,研究论文发展脉络
Chat Paper
正在生成论文摘要