SOAP: Cross-sensor Domain Adaptation for 3D Object Detection Using Stationary Object Aggregation Pseudo-labelling
CoRR(2024)
摘要
We consider the problem of cross-sensor domain adaptation in the context of
LiDAR-based 3D object detection and propose Stationary Object Aggregation
Pseudo-labelling (SOAP) to generate high quality pseudo-labels for stationary
objects. In contrast to the current state-of-the-art in-domain practice of
aggregating just a few input scans, SOAP aggregates entire sequences of point
clouds at the input level to reduce the sensor domain gap. Then, by means of
what we call quasi-stationary training and spatial consistency post-processing,
the SOAP model generates accurate pseudo-labels for stationary objects, closing
a minimum of 30.3
show that state-of-the-art domain adaptation approaches can achieve even
greater performance in combination with SOAP, in both the unsupervised and
semi-supervised settings.
更多查看译文
关键词
Algorithms,3D computer vision,Applications,Autonomous Driving
AI 理解论文
溯源树
样例
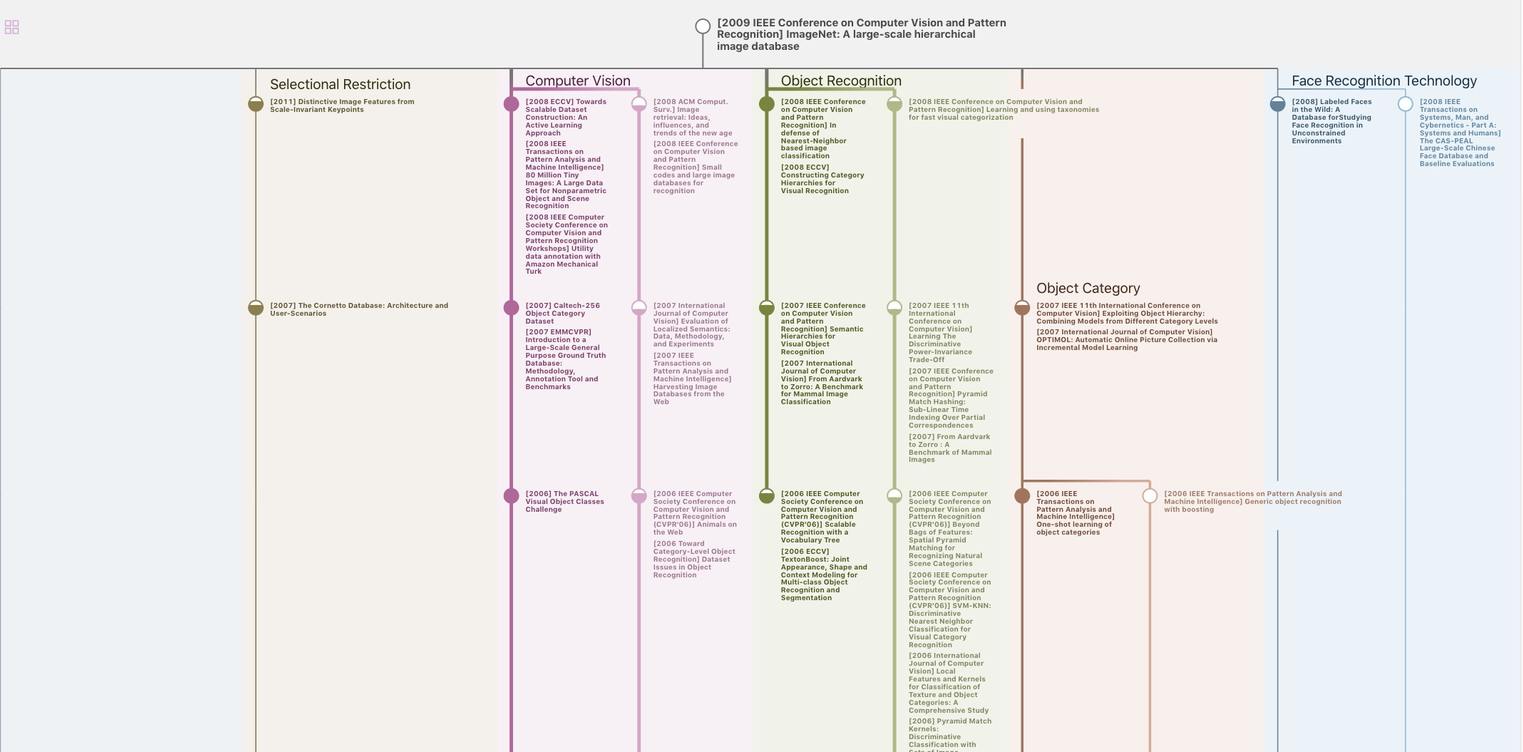
生成溯源树,研究论文发展脉络
Chat Paper
正在生成论文摘要